Uncertainty Characterization for 3D Object Detection Algorithms
2023 IEEE TRANSPORTATION ELECTRIFICATION CONFERENCE & EXPO, ITEC(2023)
摘要
Deep learning has long been the preferred method in acquiring accurate object detection in the context of autonomous driving. Research advancement in deep learning object detection keeps pushing the detection performance - typically measured by mean-average-precision (mAP) - to new records in open datasets like KITTI and NuScene. However, as the mAP measure of performance is based on a fixed threshold value of intersection of union (IOU), it overlooks the object localization error represented by the Euclidean distance between the detected object and ground truth. This paper presents a novel method to evaluate object detection algorithm performance by characterizing localization error and uncertainty. This study selects two different stereo vision algorithms and a corresponding Lidar algorithm for evaluation. The proposed method finds the uncertainty is more significant in the depth direction than in the lateral direction, giving the spatial uncertainty distribution an elliptical shape. The results also show that the error does not always increase monotonically with respect to the depth and is algorithm dependent. The uncertainty characteristics captured from this paper can improve object tracking and multi-sensor fusion performance.
更多查看译文
关键词
Computer vision, 3D object detection, object tracking, deep learning, uncertainty characterization
AI 理解论文
溯源树
样例
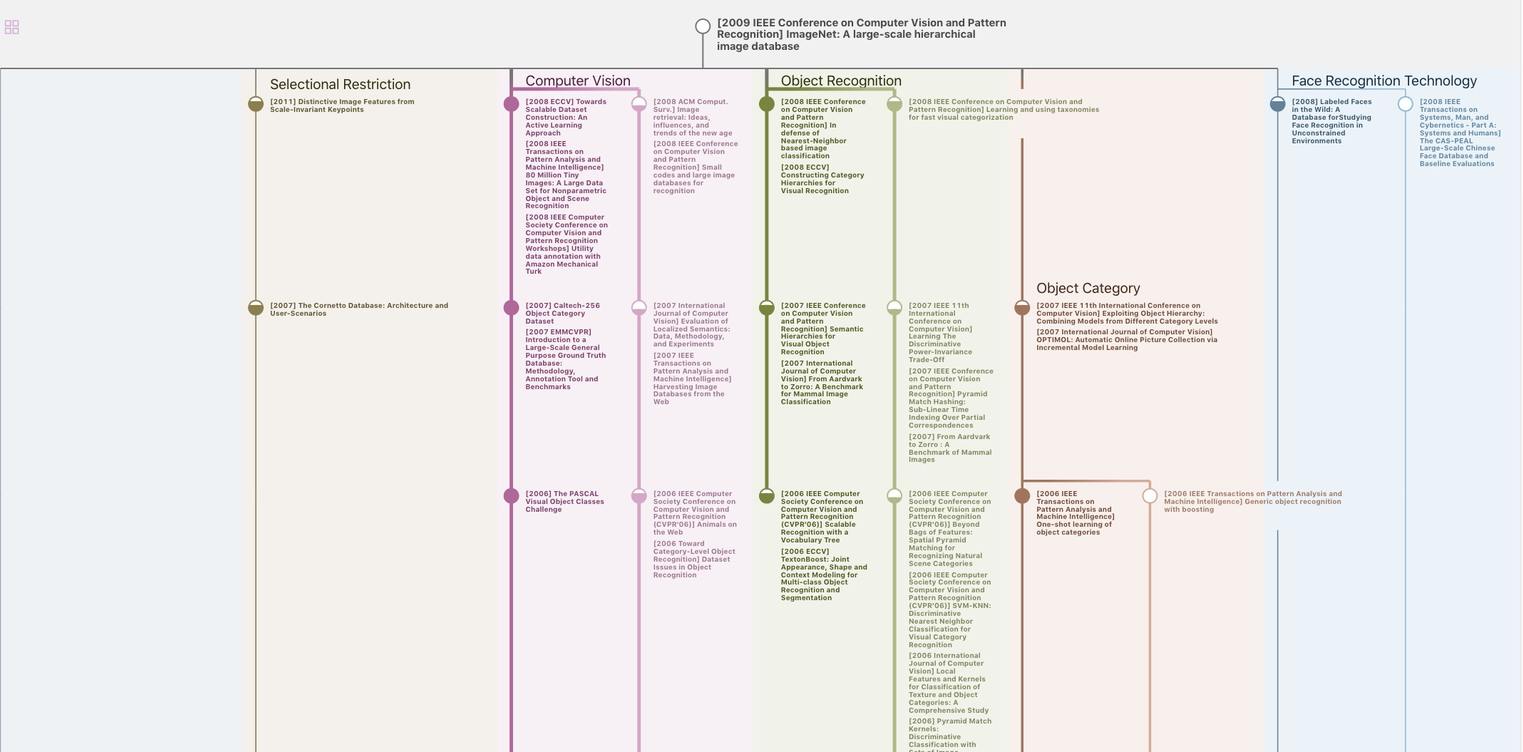
生成溯源树,研究论文发展脉络
Chat Paper
正在生成论文摘要