A Hierarchical Resource Scheduling Method for Satellite Control System Based on Deep Reinforcement Learning
ELECTRONICS(2023)
摘要
Space-based systems providing remote sensing, communication, and navigation services are essential to the economy and national defense. Users' demand for satellites has increased sharply in recent years, but resources such as storage, energy, and computation are limited. Therefore, an efficient resource scheduling strategy is urgently needed to satisfy users' demands maximally and get high task execution benefits. A hierarchical scheduling method is proposed in this work, which combines improved ant colony optimization and an improved deep Q network. The proposed method considers the quality of current task execution and resource load balance. The entire resource scheduling process contains two steps, task allocation and resource scheduling in the timeline. The former mainly implements load balance by improved ant colony optimization, while the latter mainly accomplishes the high task completion rate by an improved deep Q network. Compared with several other heuristic algorithms, the proposed approach is proven to have advantages in terms of CPU runtime, task completion rate, and resource variance between satellites. In the simulation scenarios, the proposed method can achieve up to 97.3% task completion rate, with almost 50% of the CPU runtime required by HAW and HADRT. Furthermore, this method has successfully implemented load balance.
更多查看译文
关键词
resource scheduling, deep reinforcement learning, ant colony optimization, load balance
AI 理解论文
溯源树
样例
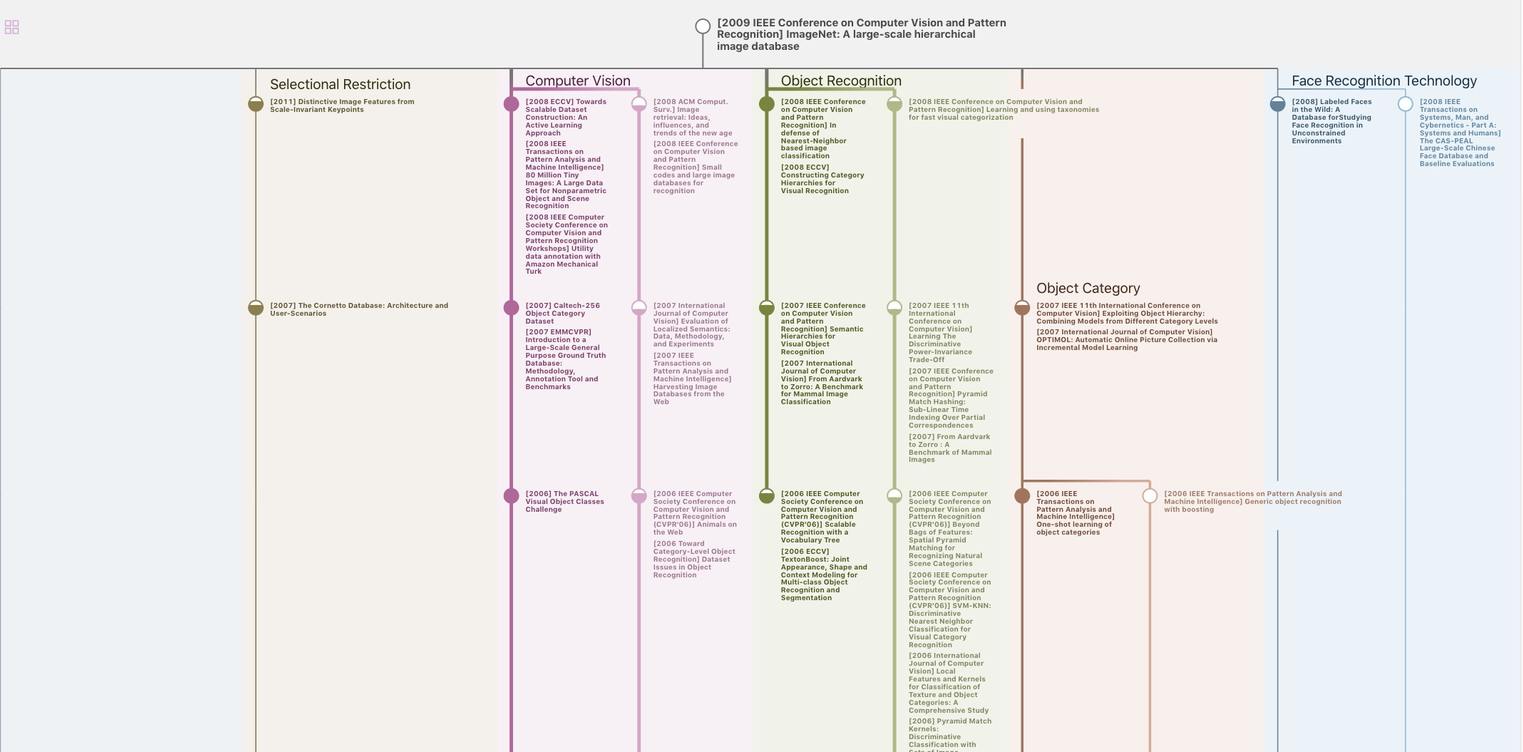
生成溯源树,研究论文发展脉络
Chat Paper
正在生成论文摘要