Localization for Intelligent Vehicles in Underground Car Parks Based on Semantic Information
IEEE TRANSACTIONS ON INTELLIGENT TRANSPORTATION SYSTEMS(2024)
Abstract
Global navigation satellite system (GNSS) signals cannot be received indoors, thus to deploy intelligent vehicles in underground car parks other localization methods are needed. In this paper, we use various carpark signs that are widely and uniformly distributed in underground parking lots as localization references. We propose a coarse-to-fine multiscale localization method that relies solely on vision sensors for underground parking lot localization based on a preconstructed lightweight node map. In coarse localization, we propose a semantic keyframe topological localization method to predict the localization range (candidate set of nodes). In node-level localization, we extract features by learning-based neural networks and construct a hybrid k-nearest neighbor (H-KNN) model to search for the closest node within the coarse localization results. In metric localization, we construct plane homography and perspective-n-point (PnP) models, allowing the vehicle's pose (rotation and translation relative to the closest node) to be computed for refined localization. The proposed method has been tested in two underground parking lots of an office building and a shopping mall with different characteristics. Experimental results demonstrate that root mean square error (RMSE) is 0.38 m and the proposed method exhibits strong robustness in various scenarios.
MoreTranslated text
Key words
Intelligent vehicles,Cameras,Intelligent vehicle,vision-only localization,semantic keyframe,multiscale matching,multilayer map
AI Read Science
Must-Reading Tree
Example
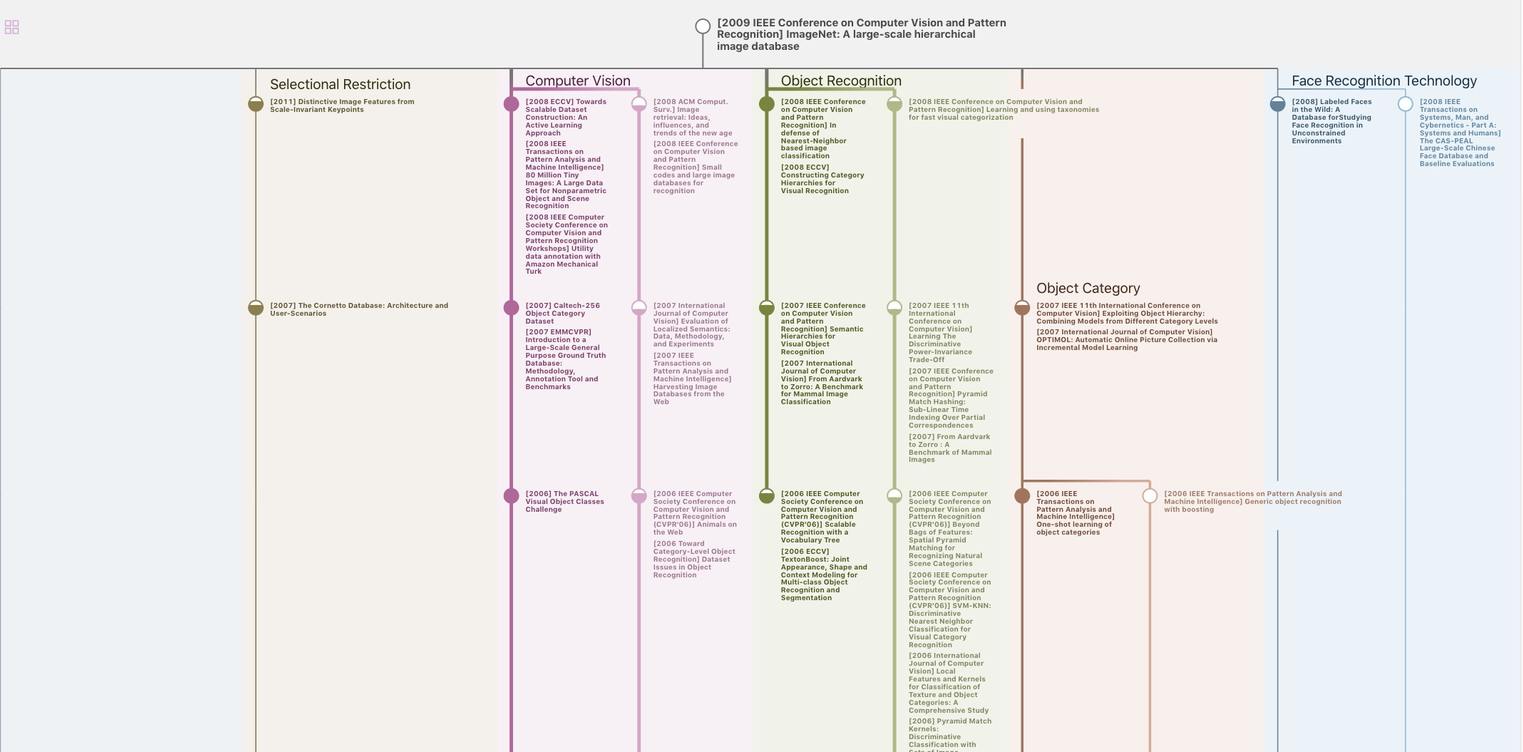
Generate MRT to find the research sequence of this paper
Chat Paper
Summary is being generated by the instructions you defined