Generating embedding spaces for re-identifying pallets from their chipwood patterns
ENGINEERING APPLICATIONS OF ARTIFICIAL INTELLIGENCE(2023)
摘要
Modern logistics rely on continuous tracking of even most basic entities like pallets. While tracking is nowadays achieved via additional markers (e.g., barcodes), pallets have a unique woodchip pattern in their blocks that can be exploited for re-identification if suitable methods are employed. This paper shows how this task can be accomplished in an open-set scenario. In this work, images of pallet blocks are transformed into vector representations using neural networks based on the ResNet-50 architecture. The networks are trained such that the Euclidean distance between image vectors of the same palette block is small. Several approaches, i.e., cross entropy, contrastive and triplet loss, to create the embedding space are compared. It is shown that Triplet Loss has advantages over other methods with a top-1 accuracy of 0.86 on the pallet-block-32965 dataset. In addition, a neighborhood-based approach to novelty detection called NSAND is presented, which outperforms a purely distance-based approach by up to 20%.
更多查看译文
关键词
Machine learning,Re-identification,Novelty detection,Logistics,Warehousing,Tracking
AI 理解论文
溯源树
样例
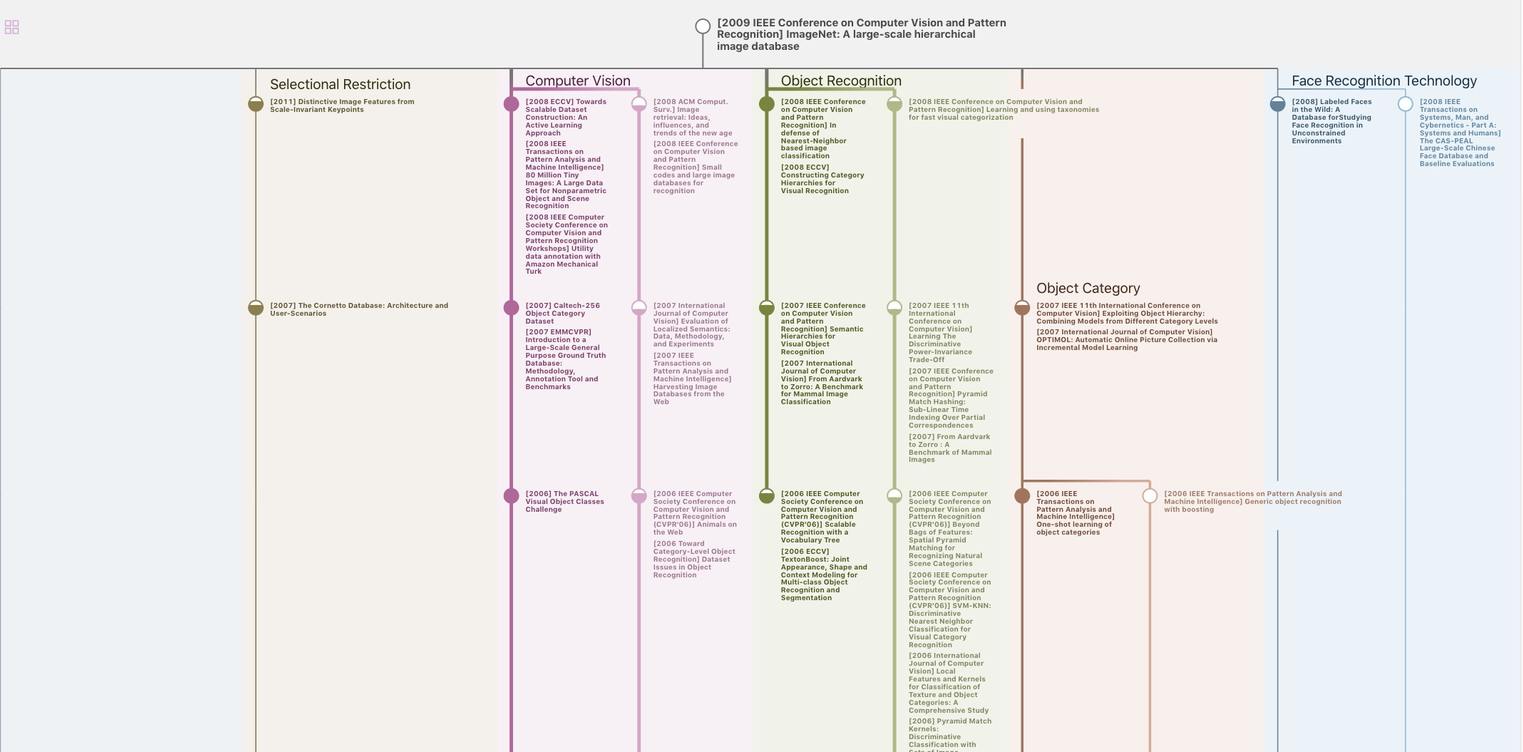
生成溯源树,研究论文发展脉络
Chat Paper
正在生成论文摘要