Global correlation analysis of strongly nonlinear frequency responses using the arclength-based separation and the Correlation-Map
JOURNAL OF SOUND AND VIBRATION(2024)
Abstract
Global correlation analysis is an important technique to quantify both the shape and amplitude differences between two response vectors. In linear dynamic systems, differences between two Frequency Response Functions (FRFs) are quantified as scalar number curves of the Global Shape Criterion (GSC) and the Global Amplitude Criterion (GAC), to represent FRF similarities at different frequencies. From linear to nonlinear, responses are usually obtained at different fre-quencies to form the Frequency Response Curve (FRC), replacing the FRF for dynamic analysis. Extending the concept of global correlation analysis from linear FRFs to nonlinear FRCs could quantify shape and amplitude similarities between nonlinear models. However, global correlation analysis for multivalued FRCs with a strong nonlinearity is hard to conduct, as strongly nonlinear correlation functions have complex multivalued phenomena with real/fake characteristics. In this paper, the Global Shape Curve Criterion (GSCC) and Global Amplitude Curve Criterion (GACC) are proposed for the correlation analysis of strongly nonlinear FRCs, which can quantify the similarity between two FRCs with different and complex multivalued phenomena. Through the arclength-based separation, multivalued FRCs are separated to single-valued response branches, in order to compute single-valued correlation functions that form the multivalued correlation function. The computed correlations contain the GSCC and GACC, which separately represent shape and amplitude differences between two FRCs at each frequency. The multivalued corre-lation function is represented as a Correlation-Map (C-MAP) to extract real correlation charac-teristics, for accurate correlation analysis. The multivalued correlation analysis is first verified on a 3 DOF model with a strong nonlinearity. Differences between the reference and initial multi-valued FRCs are successfully quantified as scalar curves and the GACC may be more sensitive than the GSCC on models with a local nonlinearity. Then, the proposed method is further validated on an experimental 3 DOF model. Very complex 15-valued correlation functions between FRCs with different multivalued phenomena are established. Even so, the real correlations are still suc-cessfully extracted by the C-MAP. These show the validity and superiority of the proposed method.
MoreTranslated text
Key words
Multivalued correlation function,Multivalued frequency response curve,Strong nonlinearity,Correlation-Map,Arclength-based separation
AI Read Science
Must-Reading Tree
Example
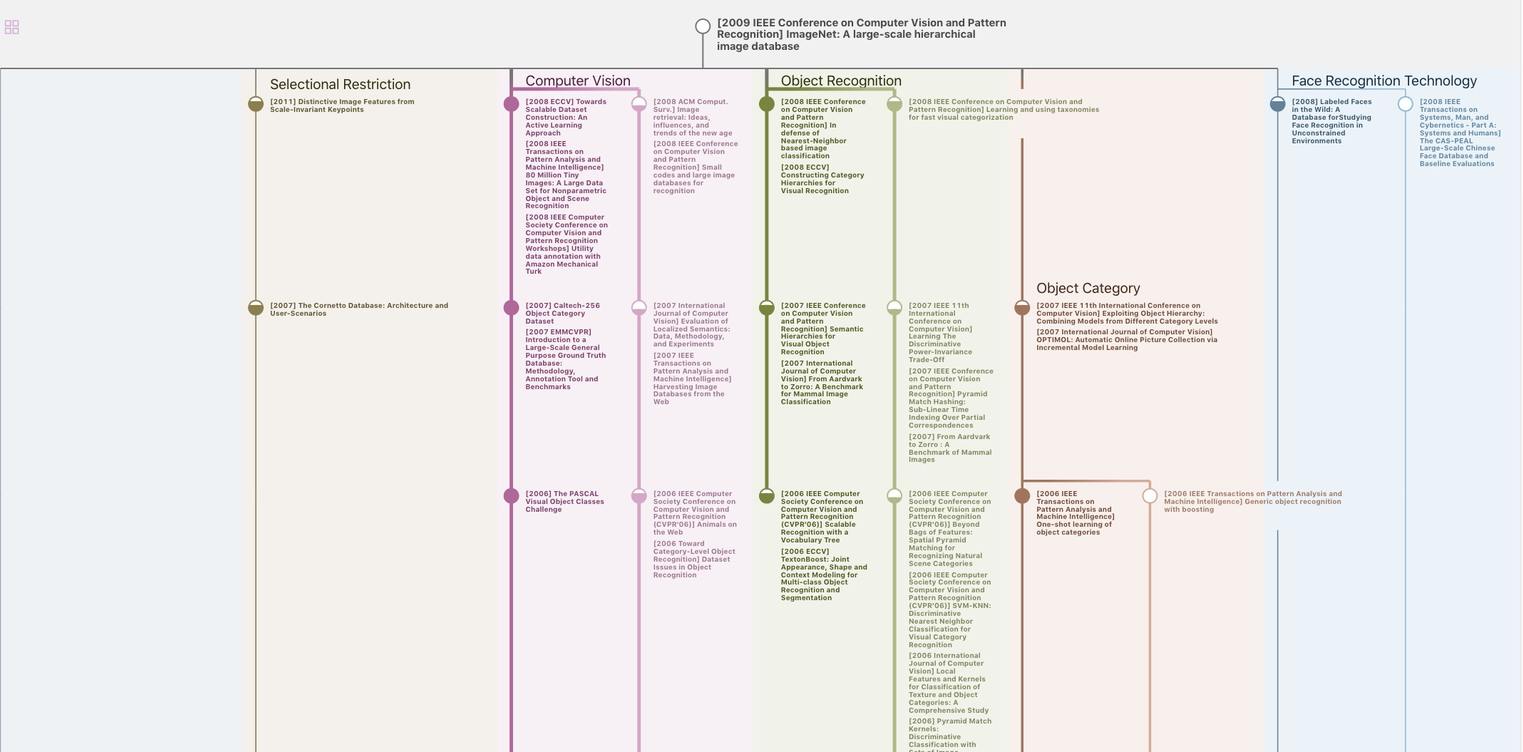
Generate MRT to find the research sequence of this paper
Chat Paper
Summary is being generated by the instructions you defined