Advancing gene feature selection: Comprehensive learning modified hunger games search for high-dimensional data
BIOMEDICAL SIGNAL PROCESSING AND CONTROL(2024)
摘要
Gene selection eliminates redundant or duplicate information to optimize computational resources and improve classification accuracy. In this research, a novel gene selection technique called CLMHGS is introduced, which utilizes the hunger games search (HGS) framework along with an comprehensive learning strategy and a modified approach food strategy to gene selection. The primary objective of CLMHGS is to achieve global optimization. Additionally, its binary counterpart, bCLMHGS, has been employed to address the challenge of feature selection. To prove the efficacy of CLMHGS, it is compared to HGS, single strategy embedded HGS, eight recent algorithms, and seven advanced algorithms on the IEEE CEC 2017 suite. Besides, CLMHGS outperforms many CEC winners and highly effective differential evolution (DE)-based approaches on benchmark functions. Moreover, based on classification accuracy, the quantity of selected features, fitness values, and execution time, the experimental findings indicate that bCLMHGS outperforms both bHGS and the majority of other feature selection algorithms across 14 public datasets. Thus, bCLMHGS can be an excellent optimizer and a powerful wrapper-mode feature selection method.
更多查看译文
关键词
Feature selection,Hunger games search,HGS,Gene data analysis,Optimization
AI 理解论文
溯源树
样例
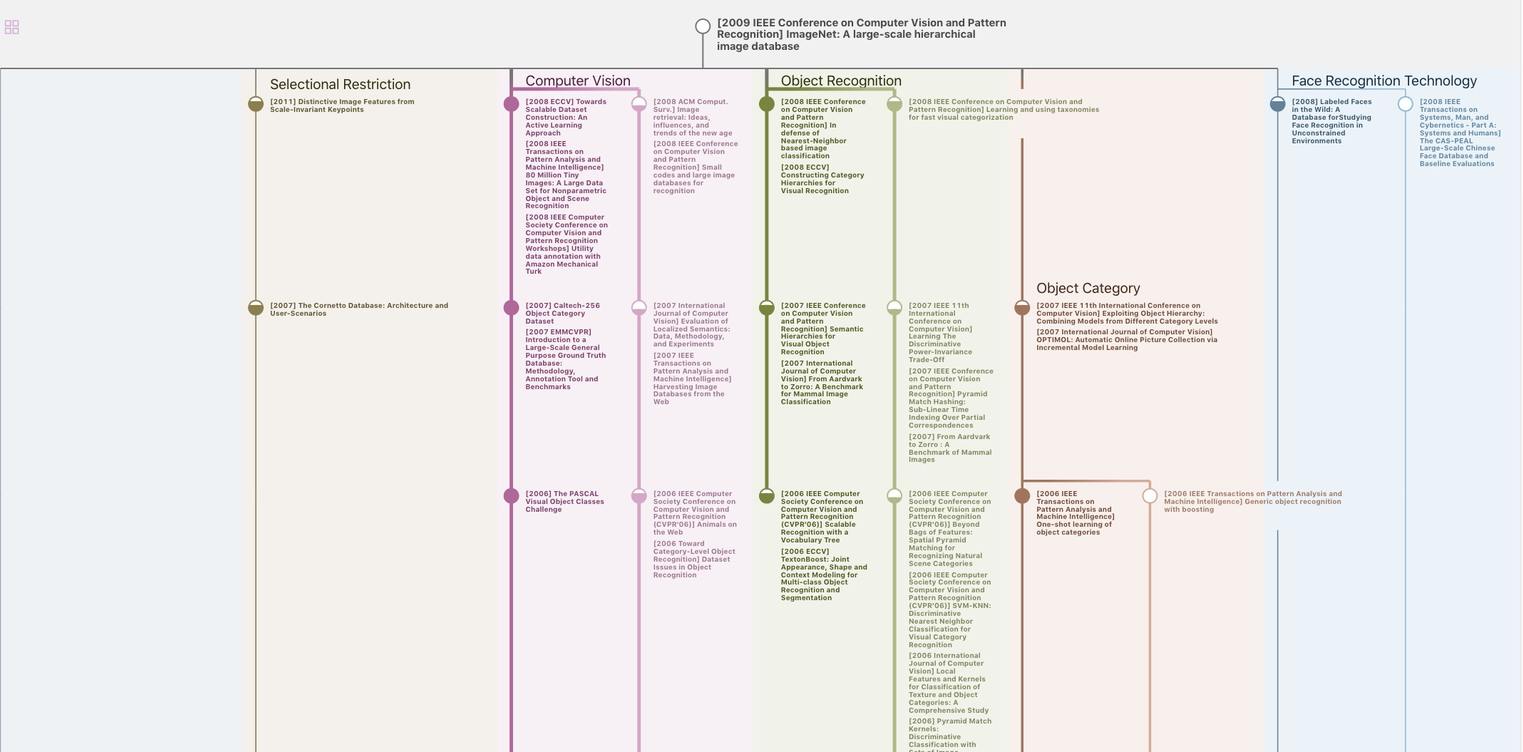
生成溯源树,研究论文发展脉络
Chat Paper
正在生成论文摘要