Data programming enabled weak supervised labeling for ECG time series
BIOMEDICAL SIGNAL PROCESSING AND CONTROL(2024)
摘要
Electrocardiogram (ECG) beat labeling performed using conventional methods is unsuitable for ECG signals obtained from Internet of Things (IoT) wearable devices. The conventional methods employ manually labeled data captured using multiple leads, while most IoT devices produce unlabeled single lead data. Getting ECG data labeled by a subject matter expert (SME) is a resource/time/cost-intensive task. Our research addresses this challenge by proposing an automatic labeling technique for ECG time-series data obtained from a single lead. The technique employs a data programming (DP) enabled weak supervised learning (WSL) technique for automatic labeling of ECG beats. We have proposed nine novel heuristics-based labeling functions (LFs), applied them to each ECG beat and subsequently used a generative model (GM) to assign a probabilistic label to each ECG beat employing both intra and inter-patient paradigm on MIT-BIH and INCART datasets. Further, a discriminative model (DM) is trained on top of the GM for maximizing data coverage and ensure robustness. Finally, data augmentation (DA) is used to solve the class imbalance problem inherent in ECG data. Our experimental results demonstrate a simpler, faster, and more accurate labeling method i.e., approximately 10(5) ECG beats are labeled in an hour with an accuracy of 92.2% from single lead data. In contrast with human annotators, the time and cost requirements of our proposed labeling method are significantly less.
更多查看译文
关键词
Data programming,Electrocardiogram,Few-shot learning,Multi-instance learning,Time-series classification,Weak supervised learning
AI 理解论文
溯源树
样例
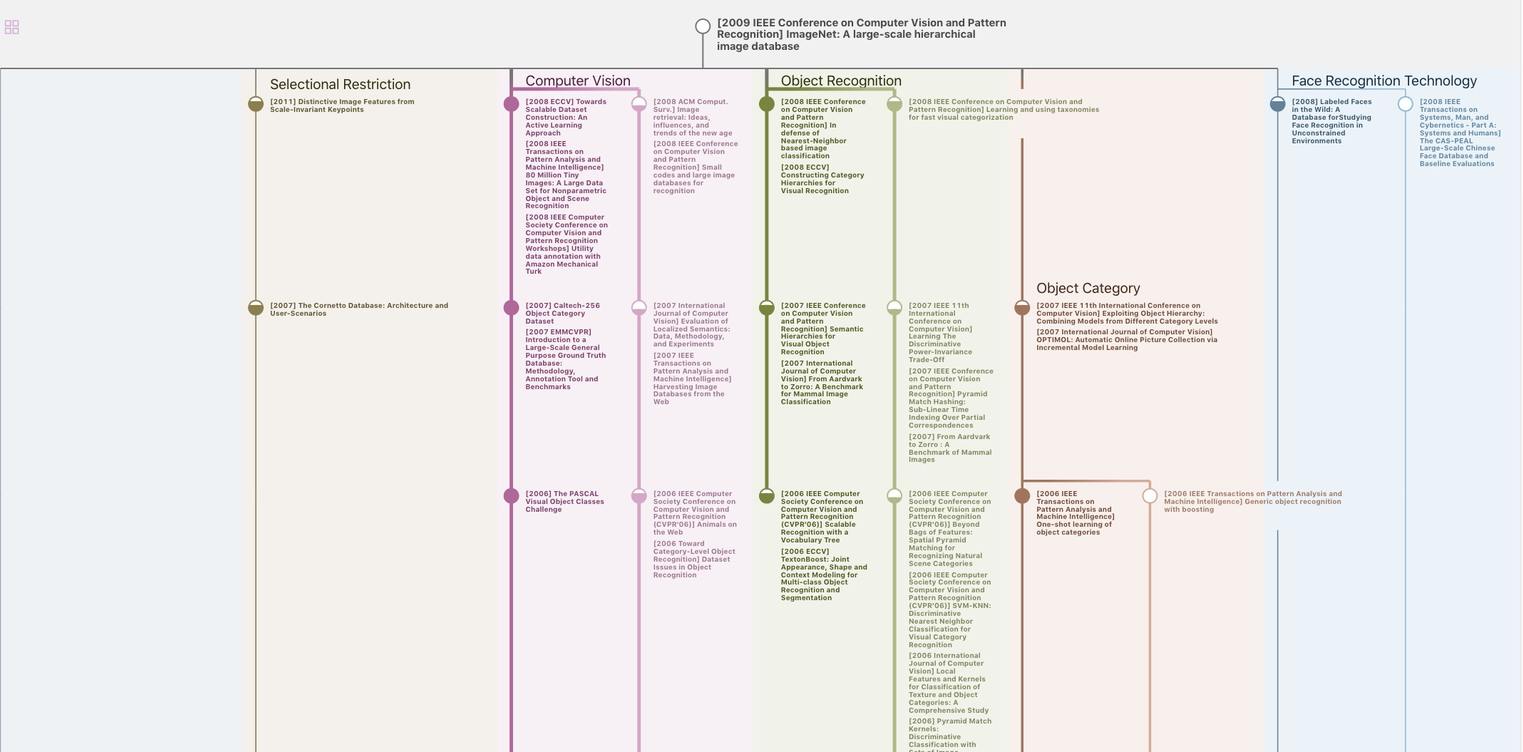
生成溯源树,研究论文发展脉络
Chat Paper
正在生成论文摘要