Similarity Graph-correlation Reconstruction Network for unsupervised cross-modal hashing
EXPERT SYSTEMS WITH APPLICATIONS(2024)
摘要
Existing cross-modal hash retrieval methods can simultaneously enhance retrieval speed and reduce storage space. However, these methods face a major challenge in determining the similarity metric between two modalities. Specifically, the accuracy of intra-modal and inter-modal similarity measurements is inadequate, and the large gap between modalities leads to semantic bias. In this paper, we propose a Similarity Graph-correlation Reconstruction Network (SGRN) for unsupervised cross-modal hashing. Particularly, the local relation graph rebasing module is used to filter out graph nodes with weak similarity and associate graph nodes with strong similarity, resulting in fine-grained intra-modal similarity relation graphs. The global relation graph reconstruction module is further strengthens cross-modal correlation and implements fine-grained similarity alignment between modalities. In addition, in order to bridge the modal gap, we combine the similarity representation of real-valued and hash features to design the intra-modal and inter-modal training strategies. SGRN conducted extensive experiments on two cross-modal retrieval datasets, and the experimental results effectively validated the superiority of the proposed method and significantly improved the retrieval performance.
更多查看译文
关键词
Cross-modal retrieval,Unsupervised cross-modal hashing,Similarity matrix,Graph rebasing,Similarity reconstruction
AI 理解论文
溯源树
样例
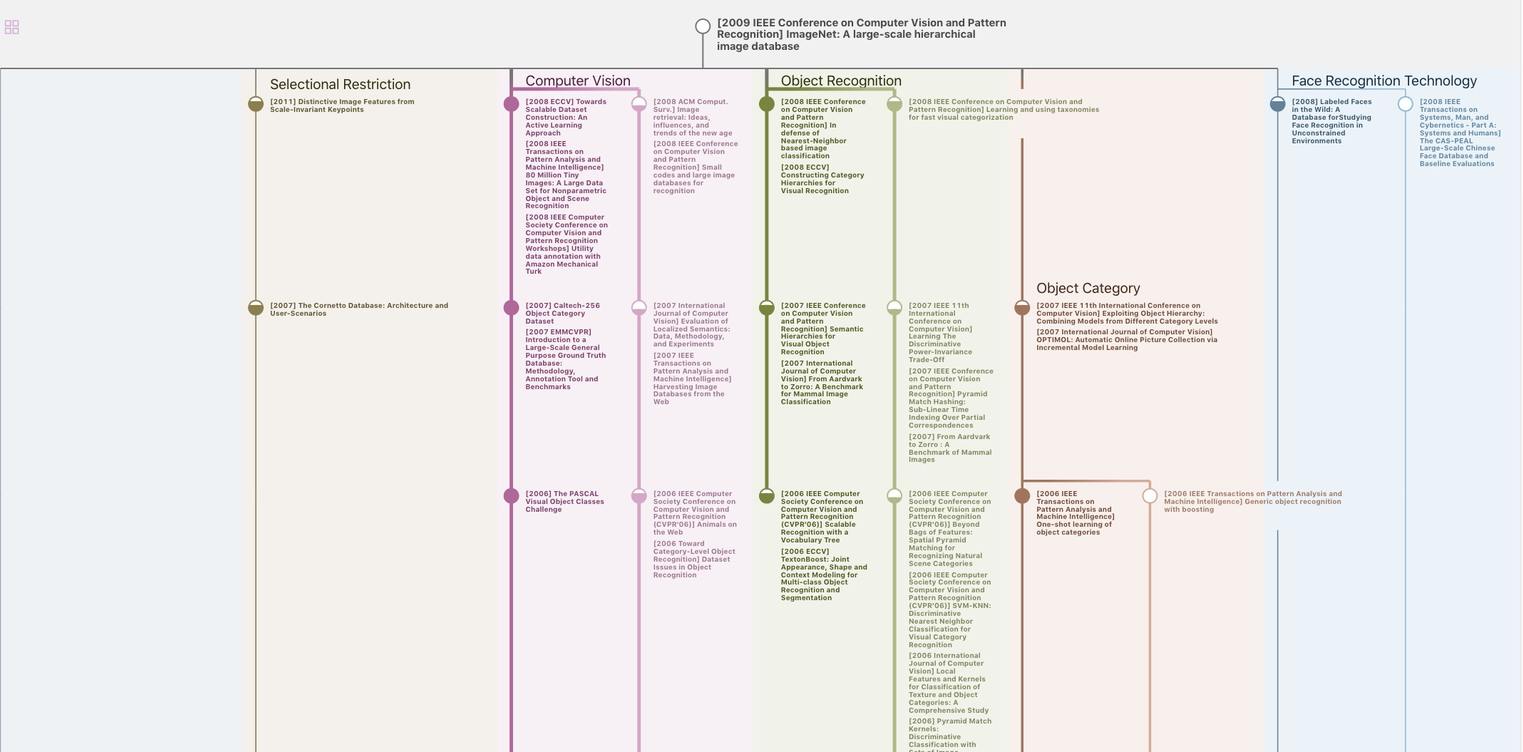
生成溯源树,研究论文发展脉络
Chat Paper
正在生成论文摘要