Locality Robust Domain Adaptation for cross-scene hyperspectral image classification
EXPERT SYSTEMS WITH APPLICATIONS(2024)
摘要
Domain adaptation (DA) has become a widely used technique for cross-scene hyperspectral image (HSI) classification. Most DA methods aim to learn a domain invariant subspace that reduce the domain discrepancy between source and target domains. However, some of them fail to explore the local manifold structure between different domains, while also neglecting the negative influences of abnormal features. To solve these problems, we propose a Locality Robust Domain Adaptation (LRDA) method for cross-domain data recognition. In LRDA, the statistical alignment is applied to reduce the domain-shift between the source and target domains. Then, LRDA combines the row-sparsity constraint and the discriminant regularization term to learn a robust projection matrix, while maintaining the discriminative capability of the matrix. Furthermore, a manifold regularization term is proposed to automatically learn the nearest neighbors and weights between two domains. The proposed LRDA not only reduces the discrepancy between two domains but also explores the local neighbor information between them. Experiment results on three HSI datasets illustrate that the proposed LRDA has better performance than other related methods.
更多查看译文
关键词
Feature extraction,Image classification,Domain adaptation,Hyperspectral image
AI 理解论文
溯源树
样例
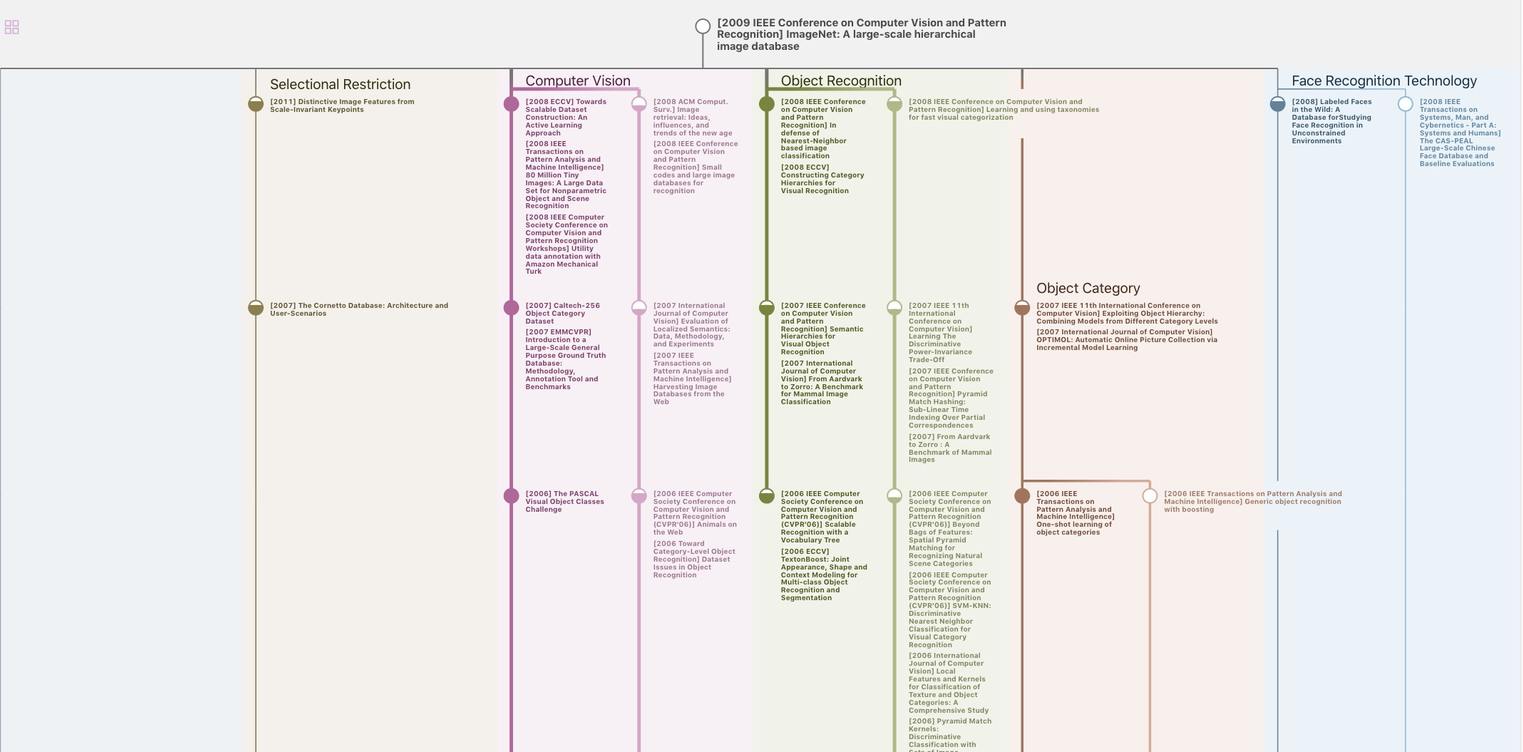
生成溯源树,研究论文发展脉络
Chat Paper
正在生成论文摘要