Contrastive learning via swapped cluster assignments for bearing fault diagnosis
MEASUREMENT SCIENCE AND TECHNOLOGY(2024)
摘要
In industrial scenarios, machinery and equipment operate in environments with many uncontrollable factors. These factors have a significant impact on vibration signals. For instance, differences in rotational speeds can make the collected data inconsistent. As a result, the tags collected from industrial environments are untrustworthy. It is impossible to know how many types of vibration signals are included in the dataset. In this paper, we propose a self-supervised pre-training method for bearing fault diagnosis called contrastive learning via swapped cluster assignments (CLSCA). Technically, CLSCA is based on momentum contrast (MoCo) and uses an unsupervised clustering algorithm to replace the classification of positive and negative samples in MoCo. By autonomously extracting representations from unlabelled datasets and then generating pseudo-labels using a clustering algorithm, CLSCA is able to learn in a self-supervised manner. When the value of the clustering center is in the appropriate range, the classification accuracy of CLSCA is improved by about 4% compared to MoCo.
更多查看译文
关键词
intelligent fault diagnosis,contrastive learning,data augmentation,clustering algorithm,dataset availability
AI 理解论文
溯源树
样例
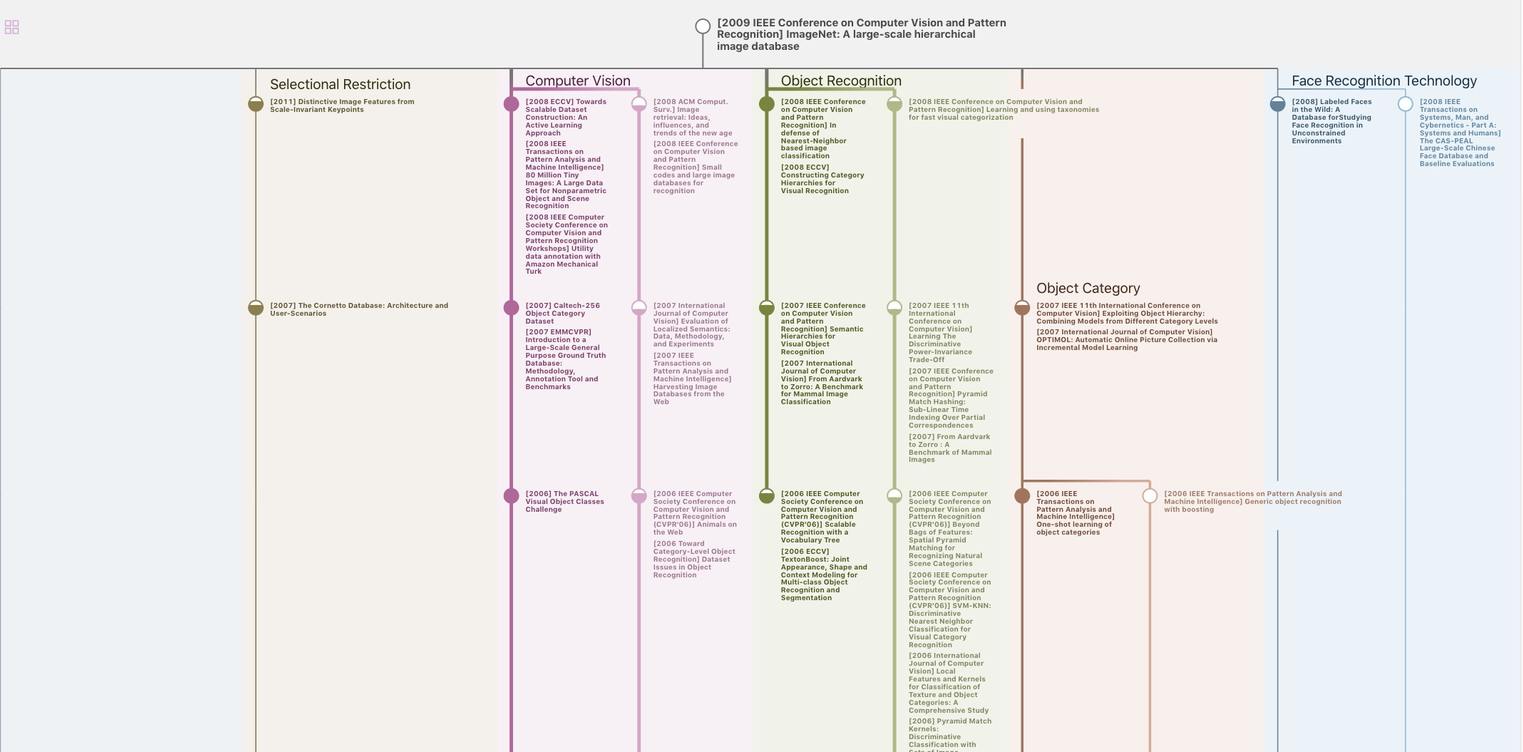
生成溯源树,研究论文发展脉络
Chat Paper
正在生成论文摘要