Embedding Prior Knowledge into Data-Driven Structural Performance Prediction to Extrapolate from Training Domains
JOURNAL OF ENGINEERING MECHANICS(2023)
Abstract
Machine learning (ML)-based data-driven approaches have become increasingly prevalent for predicting structural performance. Because a properly trained ML model can learn hidden patterns in databases of experimental samples, ML model performance sometimes exceeds that of mechanics-based predictive models, especially when the latter either conflates multiple phenomena into a single term or does not represent them at all. Nevertheless, there is almost always an inherent gap between the domain of the collected data-used in developing the predictive models-and the desired prediction domain. For instance, structural testing is often carried out on scaled components with approximated boundary conditions. Although mechanics-based models can usually bridge the said gaps, discrepancies are a perpetual challenge to the extrapolation capabilities of data-driven models. To address this issue, a new data-driven approach, a prior-knowledge embedded data-driven approach (PkeDA), is proposed herein, which integrates valuable prior knowledge embedded in empirical formulas into a data-driven model. A particular realization of this approach, based on artificial neural networks, PkeDA-ANN, is developed. To verify its feasibility and compare it with a model trained through a classic data-driven approach (CDA), a case study for predicting the bending capacities of reinforced concrete beams is carried out. The results indicate that when the model is trained via CDA, it exhibits good interpolation capabilities, as expected, but its extrapolation capabilities are observed to be severely limited. Under identical conditions, the proposed PkeDA was observed to have not only excellent interpolation and extrapolation capabilities but also to dramatically surpass the classic empirical formulas that have been developed for predicting the capacity of concrete beams under bending. As such, PkeDA appears to be a viable approach for developing highly accurate data-driven models when prior knowledge is available.
MoreTranslated text
Key words
Structural performance prediction, Data-driven method, Extrapolation capacity, Prior knowledge, Machine learning (ML)
AI Read Science
Must-Reading Tree
Example
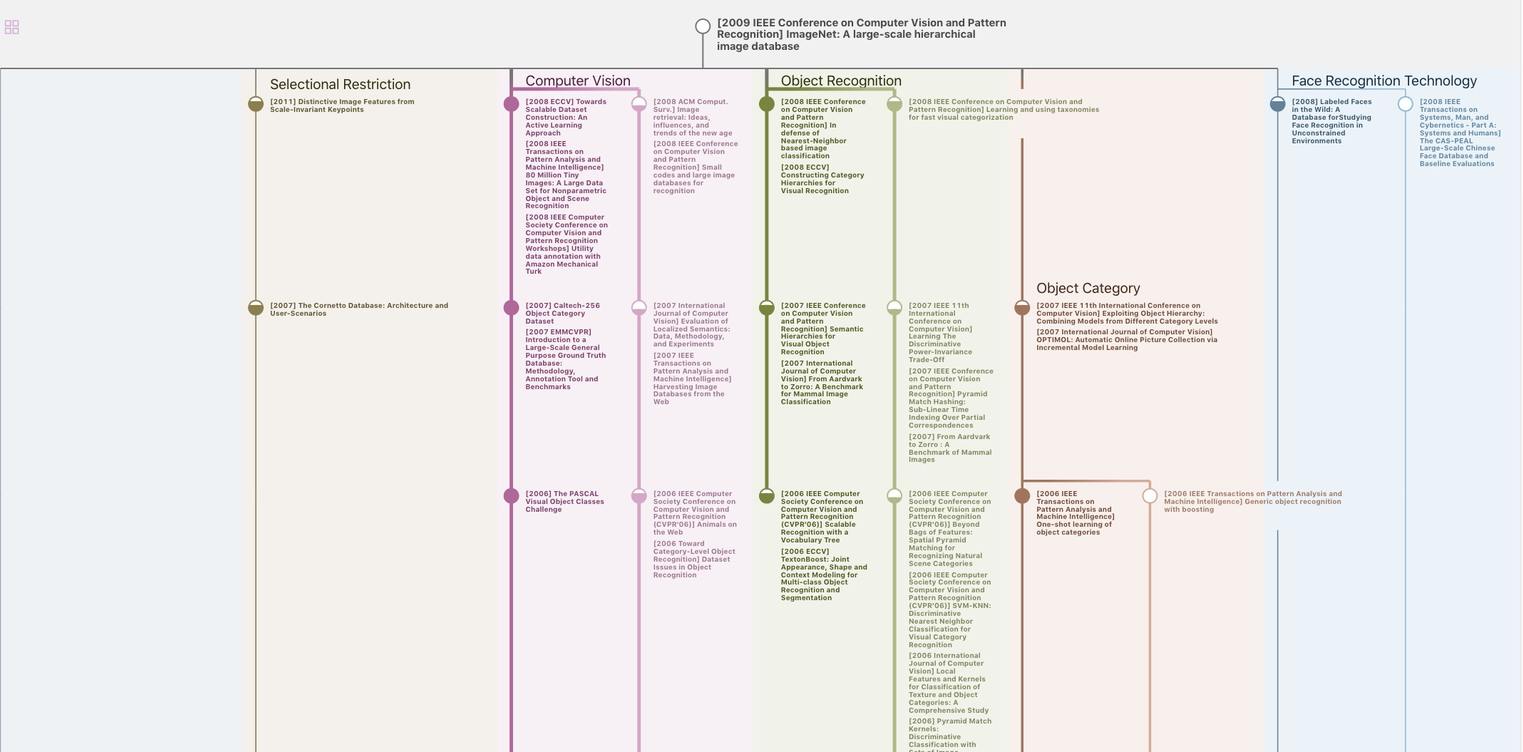
Generate MRT to find the research sequence of this paper
Chat Paper
Summary is being generated by the instructions you defined