Recurrence Network-Based 3D Geometry Representation Learning for Quality Control in Additive Manufacturing of Metamaterials
JOURNAL OF MANUFACTURING SCIENCE AND ENGINEERING-TRANSACTIONS OF THE ASME(2023)
摘要
Metamaterials are designed with intricate geometries to deliver unique properties, and recent years have witnessed an upsurge in leveraging additive manufacturing (AM) to produce metamaterials. However, the frequent occurrence of geometric defects in AM poses a critical obstacle to realizing the desired properties of fabricated metamaterials. Advances in three-dimensional (3D) scanning technologies enable the capture of fine-grained 3D geometric patterns, thereby providing a great opportunity for detecting geometric defects in fabricated metamaterials for property-oriented quality assurance. Realizing the full potential of 3D scanning-based quality control hinges largely on devising effective approaches to process scanned point clouds and extract geometric-pertinent information. In this study, a novel framework is developed to integrate recurrence network-based 3D geometry profiling with deep one-class learning for geometric defect detection in AM of metamaterials. First, we extend existing recurrence network models that focus on image data to represent 3D point clouds, by designing a new mechanism that characterizes points' geometric pattern affinities and spatial proximities. Then, a one-class graph neural network (GNN) approach is tailored to uncover topological variations of the recurrence network and detect anomalies associated with geometric defects in the fabricated metamaterial. The developed methodology is evaluated through comprehensive simulated and real-world case studies. Experimental results have highlighted the efficacy of the developed methodology in identifying both global and local geometric defects in AM-fabricated metamaterials.
更多查看译文
关键词
3d geometry representation learning,additive manufacturing,metamaterials
AI 理解论文
溯源树
样例
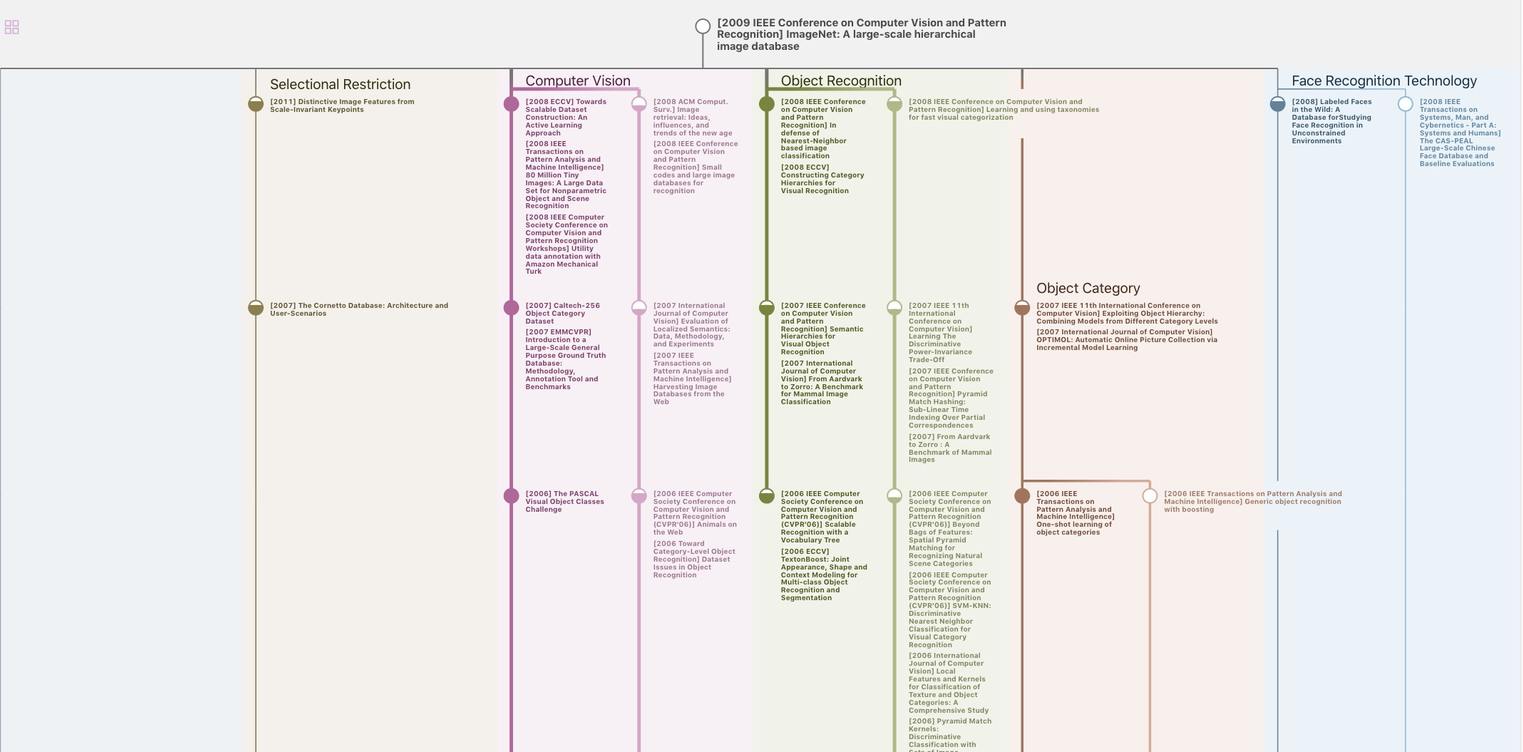
生成溯源树,研究论文发展脉络
Chat Paper
正在生成论文摘要