Predicting Herb-disease Associations Through Graph Convolutional Network
CURRENT BIOINFORMATICS(2023)
摘要
Background In recent years, herbs have become very popular worldwide as a form of complementary and alternative medicine (CAM). However, there are many types of herbs and diseases, whose associations are impossible to be fully revealed. Identifying new therapeutic indications of herbs, that is drug repositioning, is a critical supplement for new drug development. Considering that exploring the associations between herbs and diseases by wet-lab techniques is time-consuming and laborious, there is an urgent need for reliable computational methods to fill this gap. In this study, we first preprocessed the herbs and their indications in the TCM-Suit database, a comprehensive, accurate, and integrated traditional Chinese medicine database, to obtain the herb-disease association network. We then proposed a novel model based on a graph convolution network (GCN) to infer potential new associations between herbs and diseases.Background In recent years, herbs have become very popular worldwide as a form of complementary and alternative medicine (CAM). However, there are many types of herbs and diseases, whose associations are impossible to be fully revealed. Identifying new therapeutic indications of herbs, that is drug repositioning, is a critical supplement for new drug development. Considering that exploring the associations between herbs and diseases by wet-lab techniques is time-consuming and laborious, there is an urgent need for reliable computational methods to fill this gap. In this study, we first preprocessed the herbs and their indications in the TCM-Suit database, a comprehensive, accurate, and integrated traditional Chinese medicine database, to obtain the herb-disease association network. We then proposed a novel model based on a graph convolution network (GCN) to infer potential new associations between herbs and diseases.Methods In our method, the effective features of herbs and diseases were extracted through multi-layer GCN, then the layer attention mechanism was introduced to combine the features learned from multiple GCN layers, and jump connections were added to reduce the over-smoothing phenomenon caused by multi-layer GCN stacking. Finally, the recovered herb-disease association network was generated by the bilinear decoder. We applied our model together with four other methods (including SCMFDD, BNNR, LRMCMDA, and DRHGCN) to predict herb-disease associations. Compared with all other methods, our model showed the highest area under the receiver operating characteristic curve (AUROC), the area under the precision-recall curve (AUPRC), as well as the highest recall in the five-fold cross-validation.Conclusion We further used our model to predict the candidate herbs for Alzheimer's disease and found the compounds mediating herbs and diseases through the herb-compound-gene-disease network. The relevant literature also confirmed our findings.
更多查看译文
关键词
graph,predicting,herb-disease
AI 理解论文
溯源树
样例
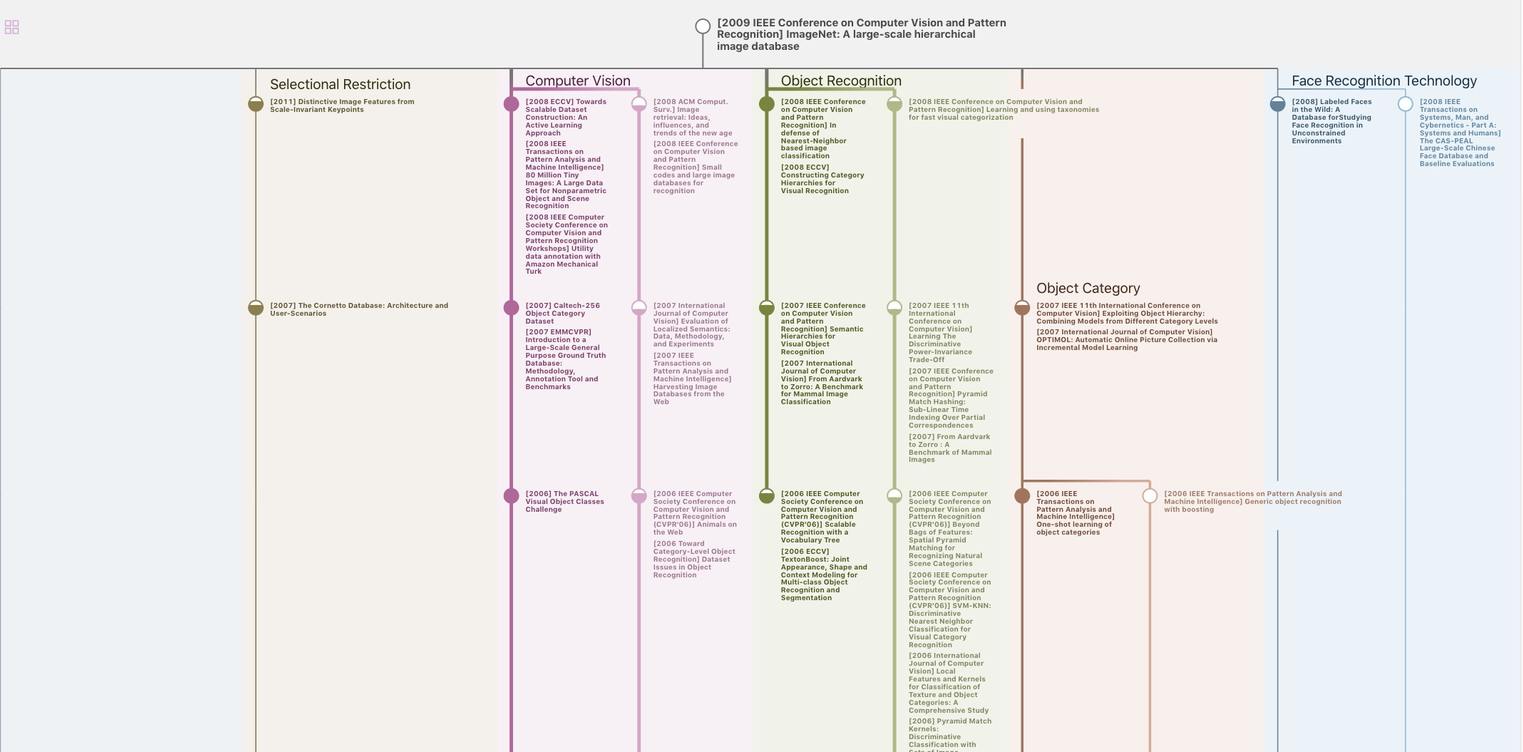
生成溯源树,研究论文发展脉络
Chat Paper
正在生成论文摘要