Evaluation of Neutron Cross-Section Data Using Gaussian Mixture Model and Digital Filter
NUCLEAR SCIENCE AND ENGINEERING(2023)
摘要
For the accurate estimation of neutron cross-section data, evaluation of nuclear data is mandatory to fulfill the need of nuclear science and technology. In this work, the evaluation of Th-232(n,2n)Th-231, Am-241(n,2n)Am-240, Mo-100(n,2n)Mo-99, and Mo-96(n,p)Nb-96 reaction cross-section data is carried out using a novel method. This novel method of evaluation employs a cluster-based piecewise evaluation followed by a digital filter for merging the evaluated curves. The clusters in the experimental data and model data are identified using the probabilistic method of the Gaussian Mixture Model. The clustered experimental data are then regressed using the polynomial regression technique. The model data are generated using the Talys 1.9 code, and the model deficiency due to the complex random nature of nuclear reactions is also accounted here using chi-squared analysis. Evaluation in each cluster is then carried out independently using the popular Kalman filter technique. For obtaining a single smooth evaluated curve for the whole energy range, the popular smoothing digital filter, the Savitzky-Golay Filter, is employed for the first time in nuclear data evaluation. The proposed evaluated curves and existing evaluated curves of Th-232(n,2n)Th-231, Am-241(n,2n)Am-240, Mo-100(n,2n)Mo-99, and Mo-96(n,p)Nb-96 reactions from nuclear data libraries such as ENDF/BVIII.0, JEFF-3.3, JENDL-4.0, CENDL-3.1, and TENDL 2021 are compared and found to be in good agreement. It is also found that generally, evaluation methods are data dependent, and so, a single evaluation method may not be applicable for all reactions of all nuclides. Since piecewise evaluation is cluster dependent, selecting the appropriate cluster makes this method robust for almost all reactions of all nuclides. Also, it is proven that this novel method of evaluation is a promising method demonstrating the potential of this approach for evaluation based on the chi-squared goodness-of-fit test with respect to standard evaluated library ENDF/BVIII.0 and experimental data.
更多查看译文
关键词
gaussian mixture model,cross-section
AI 理解论文
溯源树
样例
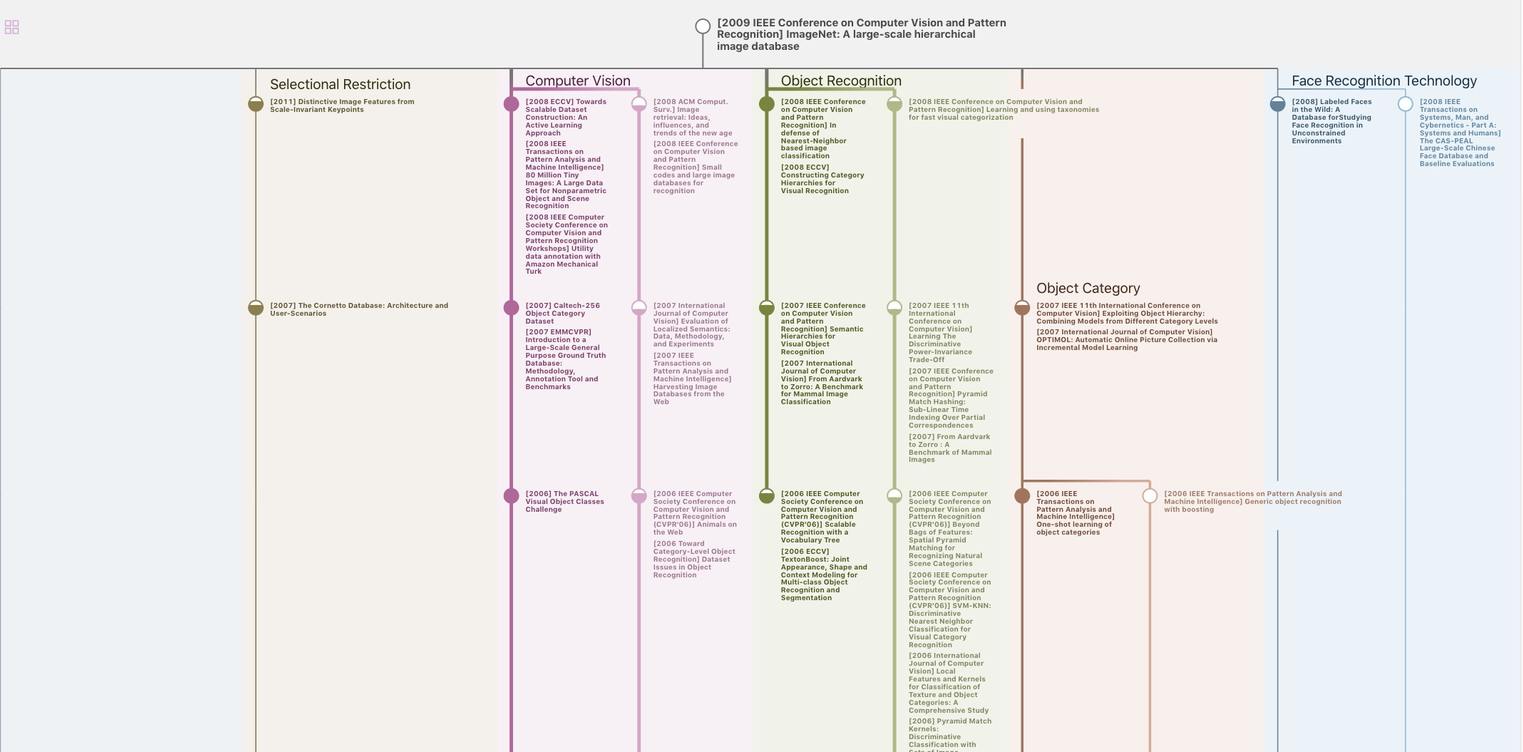
生成溯源树,研究论文发展脉络
Chat Paper
正在生成论文摘要