Reinforcement-Learning-Informed Prescriptive Analytics for Air Traffic Flow Management
IEEE TRANSACTIONS ON AUTOMATION SCIENCE AND ENGINEERING(2023)
摘要
Air Traffic Flow Management (ATFM) is a complex sequential decision-making problem that involves dynamically matching flights with sectors under changing environmental conditions. Finding an optimal solution for ATFM is challenging due to its dynamic nature and operational constraints. Reinforcement learning is a well-suited approach for sequential decision-making problems. However, ATFM poses three potential challenges: 1) large state space, 2) combinatorial action space, and 3) variational feasible action set, resulting from numerous agents with tightly-coupled constraints. These challenges can hinder the effectiveness of direct application of reinforcement learning methods. While prescriptive analytics can readily handle hard constraints via a mathematical optimization model, but it is computationally intractable for online sequential decision-making problems under changing environments. To address these challenges, we propose a novel framework, Reinforcement-Learning-Informed Prescriptive Analytics (RLIPA), in which an "informing" scheme is devised to integrate reinforcement learning and prescriptive analytics and leverage their strengths in predicting future reward and coping with hard constraints respectively. RLIPA is a general framework that can be adapted to other problems beyond ATFM, which typically involves many agents with tightly-coupled hard constraints. We demonstrate the usage and performance of RLIPA using numerical results and a real case study in comparison to two baseline approaches.Note to Practitioners-To improve Air Traffic Flow Management (ATFM) and reduce flight congestion, we propose a new method called reinforcement-learning-informed prescriptive analytics (RLIPA). RLIPA is a general framework that facilitates online sequential decision-making problems with multiple agents coupled with hard constraints. The approach consists of two stages: first, estimating future potential rewards for each agent via reinforcement learning, and second, informing the potential rewards to the following prescriptive analysis and using the information to construct and solve the downstream optimization problem dealing with hard coupling constraints among agents. Numerical experiments demonstrate the efficiency and effectiveness of RLIPA in the application of ATFM. In the most cases, RLIPA can offer more than 10x improvement in computational efficiency while maintaining or improving the level of optimality. The framework of RLIPA can be further extended to problems such as order dispatch in ride-hailing systems and food delivery.
更多查看译文
关键词
Air traffic flow management,reinforcement learning,prescriptive analytics,sequential decision-making problem
AI 理解论文
溯源树
样例
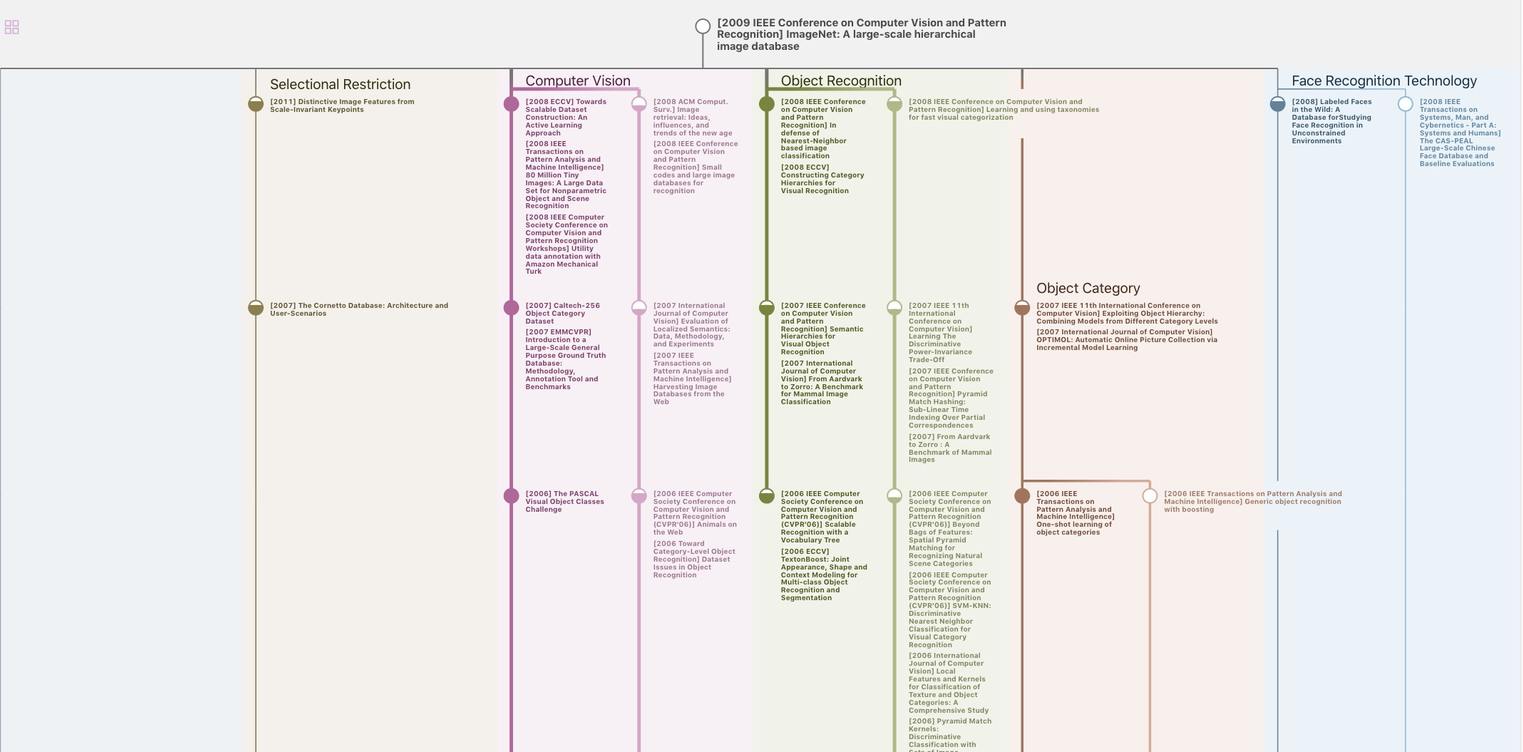
生成溯源树,研究论文发展脉络
Chat Paper
正在生成论文摘要