Data-driven safe control via finite-time Koopman identifier
INTERNATIONAL JOURNAL OF CONTROL(2023)
摘要
In this paper, a novel data-driven invariant-based safe control scheme for control of a nonlinear vehicle is developed. First, a novel incremental finite-time Koopman representation-based identifier is introduced to learn approximated lifted-states linear system model of system from a limited budget of incrementally sampled data points which not only minimises the instantaneous Koopman representation's identification errors but also the identification errors for a batch of past samples collected in a history stack. A great advantage of the presented identifier is that the uncertainty of the identified system is also quantified and updated over time on a slower time scale. Using set-theoretic tools, then, the quantified uncertainty will be leveraged in conjunction with the learned model in order to impose robust contractivity on the safe set, which guarantees its safety and stability. The proposed approach significantly reduces conservativeness through quantifying and updating the uncertainty level of the learned model over time.
更多查看译文
关键词
safe control,data-driven,finite-time
AI 理解论文
溯源树
样例
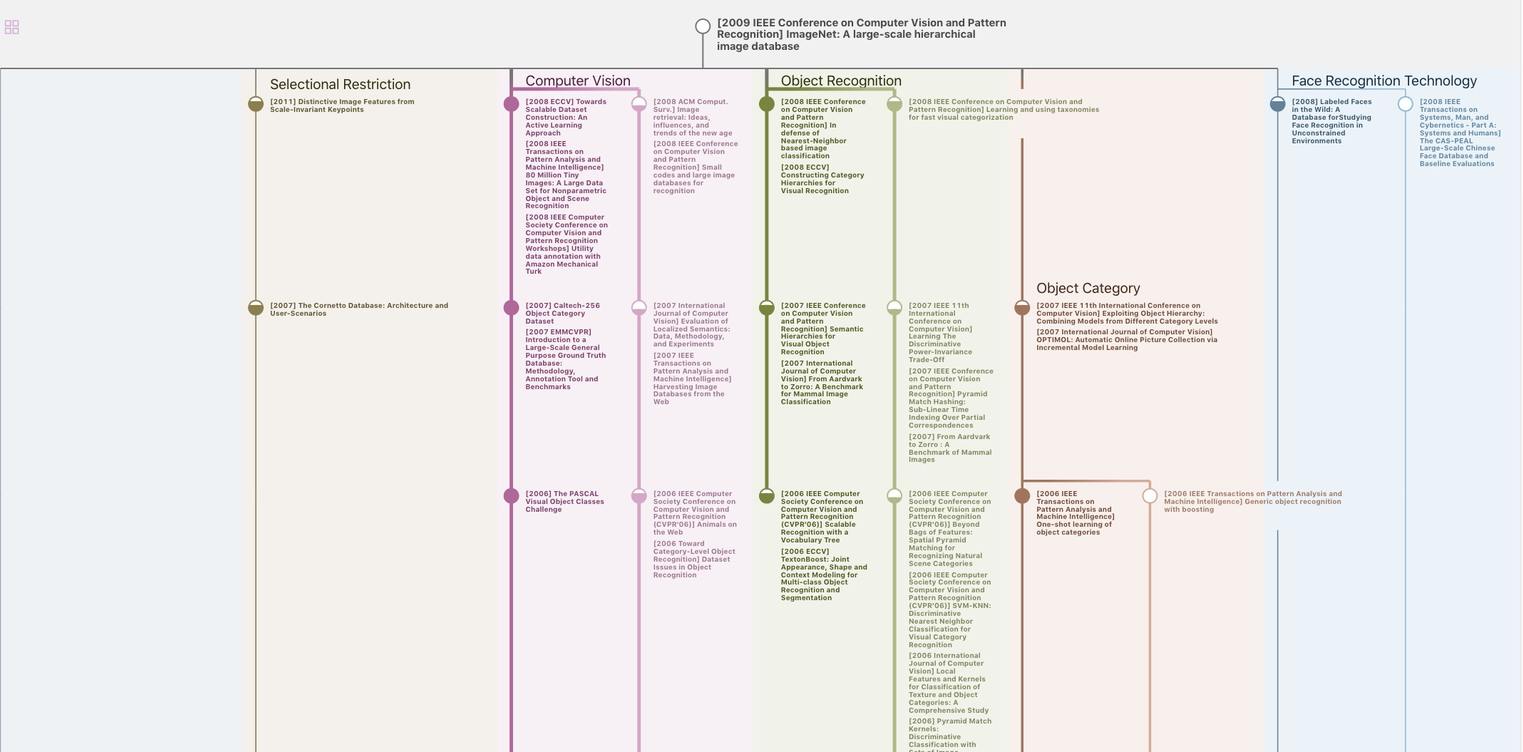
生成溯源树,研究论文发展脉络
Chat Paper
正在生成论文摘要