Prediction of normal boiling point and critical temperature of refrigerants by graph neural network and transfer learning
INTERNATIONAL JOURNAL OF REFRIGERATION(2023)
摘要
Normal boiling point (Tb) and critical temperature (Tc) are two major thermodynamic properties of refrigerants. In this study, a dataset with 742 data points for Tb and 166 data points for Tc was collected from references, and then prediction models of Tb and Tc for refrigerants were established by graph neural network and transfer learning. Graph neural network is applied to correlate the Tb and Tc of refrigerants with their corresponding molecular structure, while transfer learning is used to further improve the prediction accuracy on Tc. Compared with the data in references, the average absolute deviation for Tb is 1.20%, and for Tc, it is reduced from 1.91% to 1.05% with the help of transfer learning, which is lower than the group contribution methods. The results indicate that the graph neural network is a powerful approach to estimating refrigerant properties, and transfer learning can improve the prediction accuracy for the case of insufficient training data.
更多查看译文
关键词
Refrigerants,Thermodynamic property,Graph neural network,Transfer learning
AI 理解论文
溯源树
样例
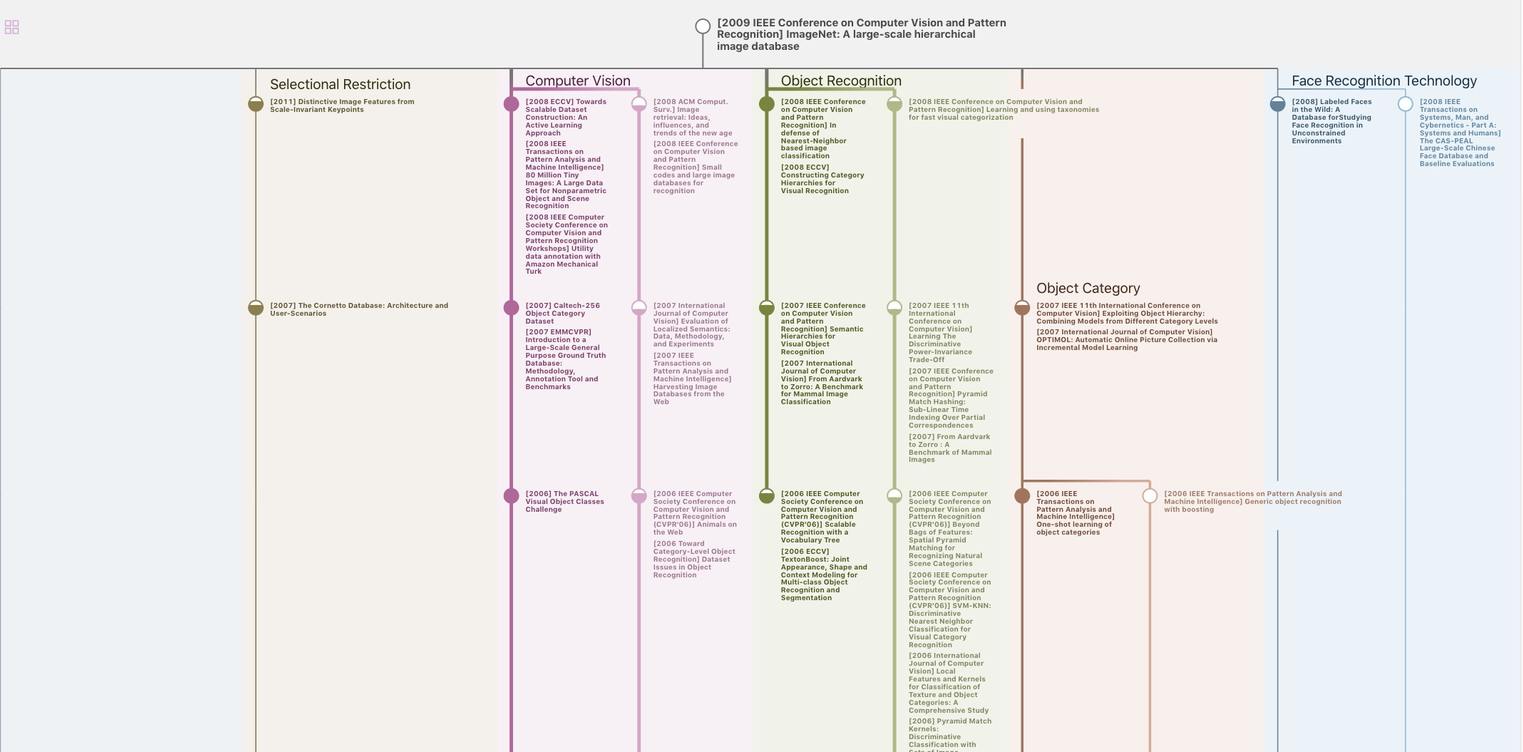
生成溯源树,研究论文发展脉络
Chat Paper
正在生成论文摘要