A long short-term memory model for forecasting the surgical case volumes at a hospital
IISE TRANSACTIONS ON HEALTHCARE SYSTEMS ENGINEERING(2023)
摘要
Surgical procedures are the primary source of expenditures and revenues for hospitals. Accurate forecasts of the volume of surgical cases enable hospitals to efficiently deliver high-quality care to patients. We propose an algorithm to forecast the expected volume of surgical procedures using multivariate time-series data. This algorithm uses feature engineering techniques to determine factors that affect the volume of surgical cases, such as the number of available providers, federal holidays, weather conditions, etc. These features are incorporated in a long short-term memory (LSTM) network to predict the number of surgical procedures in the upcoming week. The hyperparameters of this model are tuned via grid search and Bayesian optimization techniques. We develop and verify the model using historical data of daily case volume from 2014 to 2020 at an academic hospital in North America. The proposed model is validated using data from 2021. The results show that the proposed model can make accurate predictions six weeks in advance, and the average R 2 = 0.855, RMSE = 2.017, MAE = 1.104. These results demonstrate the benefits of incorporating additional features to improve the model's predictive power for time series forecasting.
更多查看译文
关键词
Clustering,forecasting surgical case volume,feature engineering,long short-term memory model,machine learning algorithm
AI 理解论文
溯源树
样例
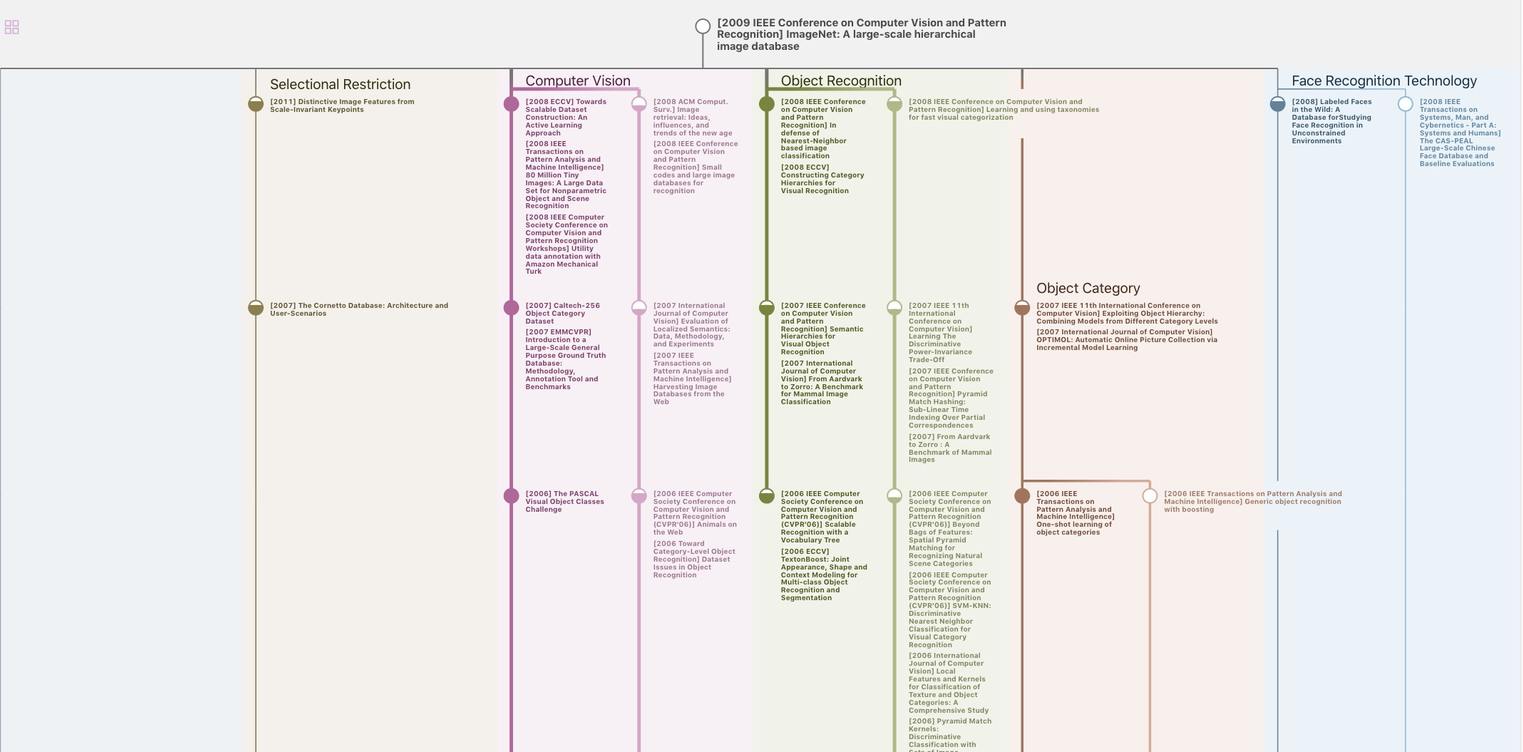
生成溯源树,研究论文发展脉络
Chat Paper
正在生成论文摘要