Low-Rank Multimanifold Embedding Learning for Multimode Process Monitoring
IEEE TRANSACTIONS ON INDUSTRIAL INFORMATICS(2024)
摘要
Modern industrial processes are in general characterized by multiple operation modes in response to diverse product requirements, and it is challenging to monitor such complex processes. Most of the existing multimodal monitoring strategies tend to model each mode individually, where relationships among different modes are ignored, leaving room for improving process monitoring performance. In this article, we propose a new monitoring approach called low-rank multimanifold embedding (LRME) for monitoring complex industrial processes with multiple operation modes, in which individualities in each mode, connections between modes, and commonalities among all modes are captured simultaneously. LRME can learn the lowest rank representation shared by all modes, revealing the common information among all modes. The within-mode and between-mode adjacency matrices are constituted to characterize the within-mode individualities and between-mode connections, which enables the reduced-dimensional representations extracted faithfully to reveal more intrinsic characterizations of multimodal data and further enhance the process monitoring capability. In addition, a point-to-manifold metric is developed to identify the running-on operation mode during online monitoring. The monitoring results on the Tennessee Eastman process and a practical industrial process demonstrate the effectiveness of our proposed approach.
更多查看译文
关键词
Index Terms-Embedding learning,low-rank represen- tation (LRR),multimanifold learning,multimode process monitoring
AI 理解论文
溯源树
样例
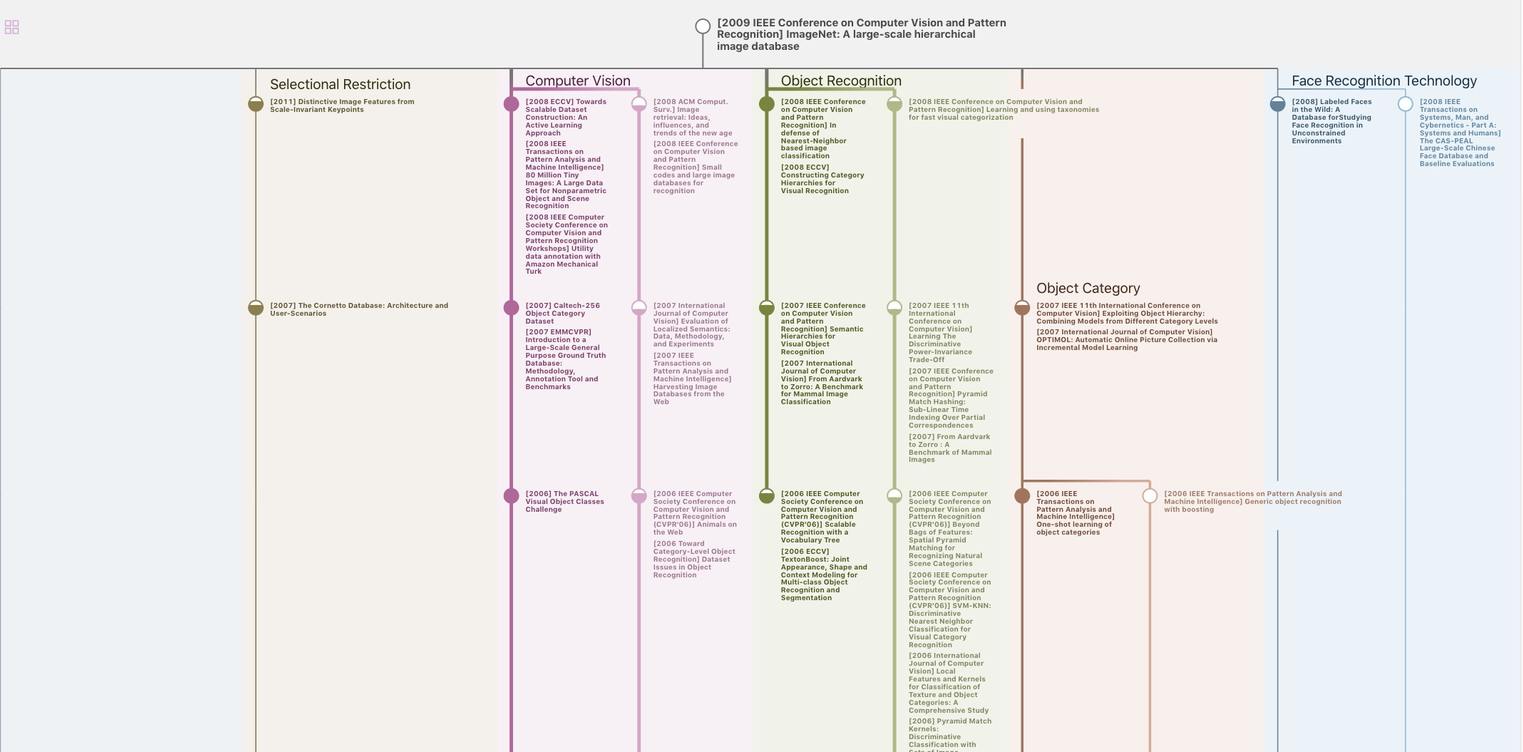
生成溯源树,研究论文发展脉络
Chat Paper
正在生成论文摘要