Multi-agent reinforcement learning satellite guidance for triangulation of a moving object in a relative orbit frame
JOURNAL OF DEFENSE MODELING AND SIMULATION-APPLICATIONS METHODOLOGY TECHNOLOGY-JDMS(2023)
摘要
Multi-agent systems are of ever-increasing importance in a contested space environment-use of multiple, cooperative satellites potentially increases positive mission outcomes on orbit, while autonomy becomes an ever-increasing requirement to increase reaction time to dynamic situations and lower the burden on space operators. This research explores multi-agent satellite swarm Guidance, Navigation, and Control (GNC) using deep reinforcement learning (DRL). DRL policies are trained to provide guidance inputs to agents in multi-agent swarm environments for completing complex, teamwork-focused objectives in geosynchronous orbit. An example scenario is explored for a group of satellite agents maneuvering to triangulate an object that is non-stationary in the relative orbit frame. Reward shaping is used to encourage learning guidance that positions swarm members to maximize triangulation accuracy, using angles-only observations for navigation relative to the target. Results show the policies successfully learn guidance through reward shaping to improve triangulation accuracy by a significant factor.
更多查看译文
关键词
triangulation,relative orbit frame,satellite,reinforcement,multi-agent
AI 理解论文
溯源树
样例
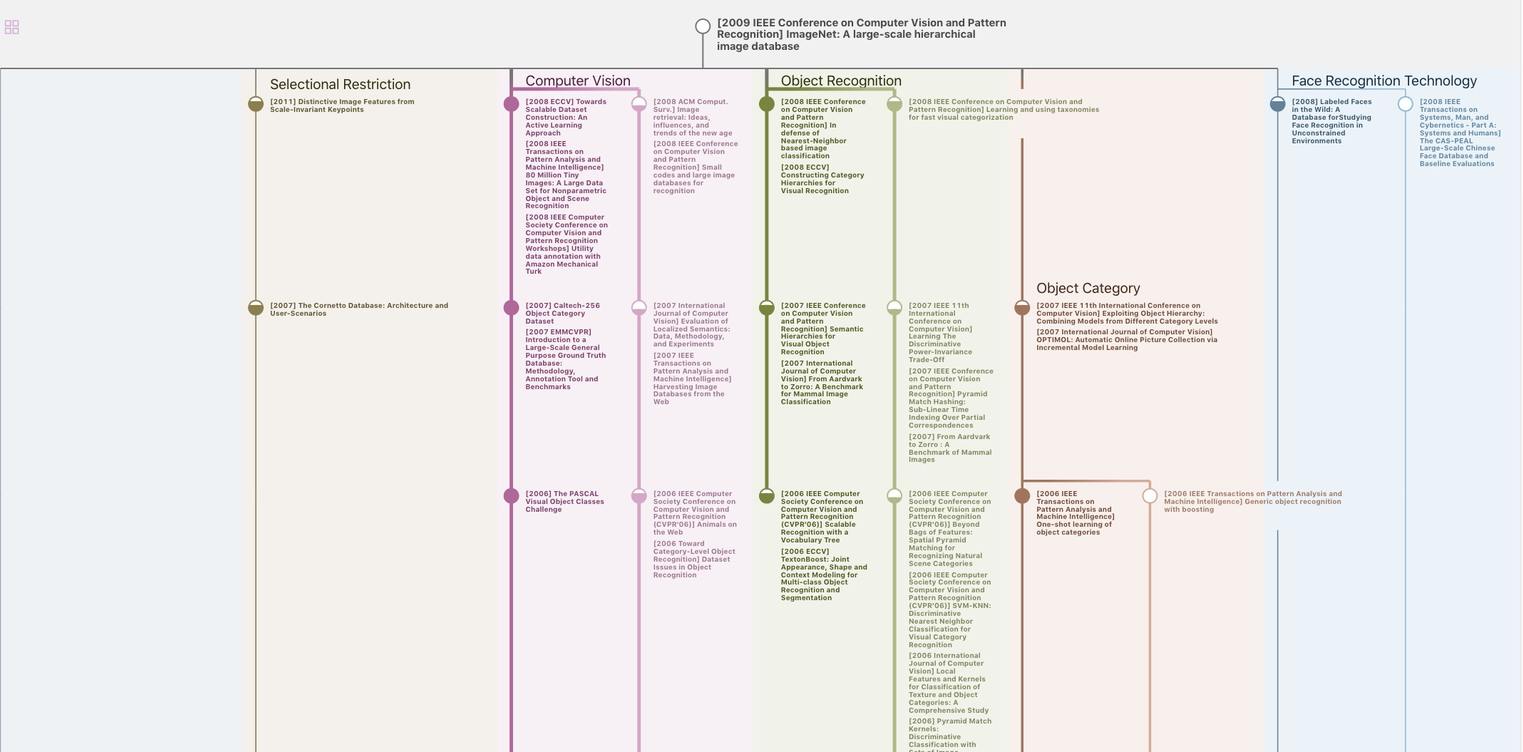
生成溯源树,研究论文发展脉络
Chat Paper
正在生成论文摘要