Instance-based transfer learning method for locating loose particles inside aerospace equipment
MEASUREMENT(2023)
Abstract
The existing supervised-learning-based loose particle localization method requires a lot of resources to create a complete loose particle localization data set, which leads to the problems of long localization testing cycle, large data storage requirement and low resource utilization. In this study, the authors proposed an instance-based transfer learning method for locating loose particles inside aerospace equipment. It used correlations between the localization data sets corresponding to the aerospace equipment with similar structure or size, to complete the knowledge transfer from the source localization data set to the new localization data set. Specifically, based on the existing localization data set containing a large amount of localization data, when facing new aerospace equipment, only a new localization data set containing small amounts of localization data needed to be created. The TrAdaBoost algorithm is used to learn on the new localization data set, the weights of the localization data in the existing localization data set are calculated, the localization data with higher weights are filtered to add to the new localization data set, thus create the transfer training data set and train the loose particle transfer localization (abbr. LPTL) model. This effectively improves the localization efficiency and data resource utilization. In addition, based on engineering application requirements, the authors proposed the definition of equipment-level loose particle localization accuracy. Tests on aerospace power supplies and aerospace electronic single-machines show that, the appropriate weight threshold was set to 0.35, 6004 pieces of localization data were filtered from the existing localization data set. The average classification accuracy of 82.85% and the average localization accuracy of 83.33% were achieved by the LPTL model based on the parameter-optimized XGBoost ensemble classifier. In theory, this method can be extended and applied to the loose particle material identification and weight estimation research, and has high reference value.
MoreTranslated text
Key words
Loose particle localization,Instance-based transfer learning,TrAdaBoost algorithm,XGBoost ensemble classifier,Localization accuracy,Aerospace equipment
AI Read Science
Must-Reading Tree
Example
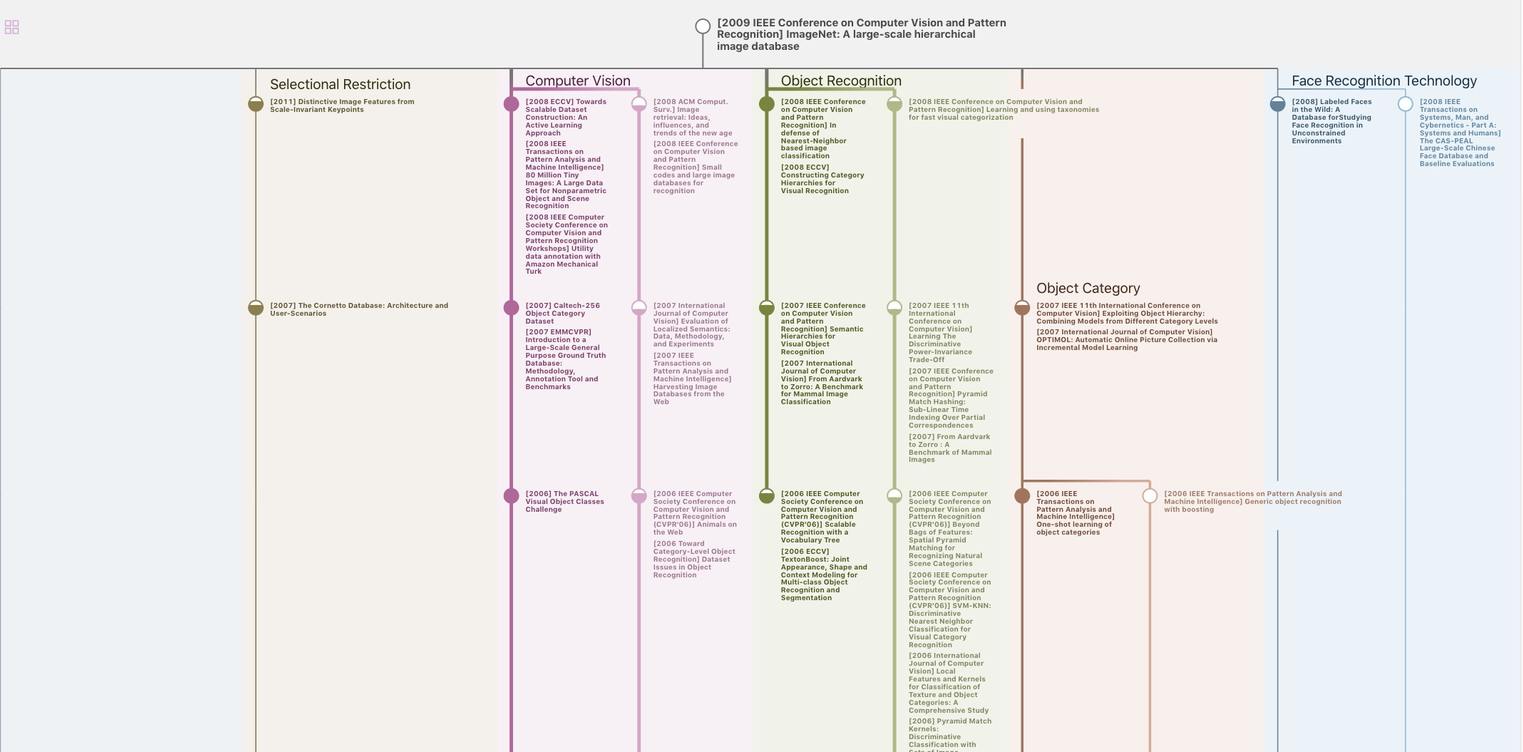
Generate MRT to find the research sequence of this paper
Chat Paper
Summary is being generated by the instructions you defined