Open-vocabulary Queryable Scene Representations for Real World Planning
2023 IEEE INTERNATIONAL CONFERENCE ON ROBOTICS AND AUTOMATION (ICRA 2023)(2023)
Abstract
Large language models (LLMs) have unlocked new capabilities of task planning from human instructions. However, prior attempts to apply LLMs to real-world robotic tasks are limited by the lack of grounding in the surrounding scene. In this paper, we develop NLMap, an open-vocabulary and queryable scene representation to address this problem. NLMap serves as a framework to gather and integrate contextual information into LLM planners, allowing them to see and query available objects in the scene before generating a context-conditioned plan. NLMap first establishes a natural language queryable scene representation with Visual Language models (VLMs). An LLM based object proposal module parses instructions and proposes involved objects to query the scene representation for object availability and location. An LLM planner then plans with such information about the scene. NLMap allows robots to operate without a fixed list of objects nor executable options, enabling real robot operation unachievable by previous methods. Project website: https://nlmap-saycan.github.io
MoreTranslated text
AI Read Science
Must-Reading Tree
Example
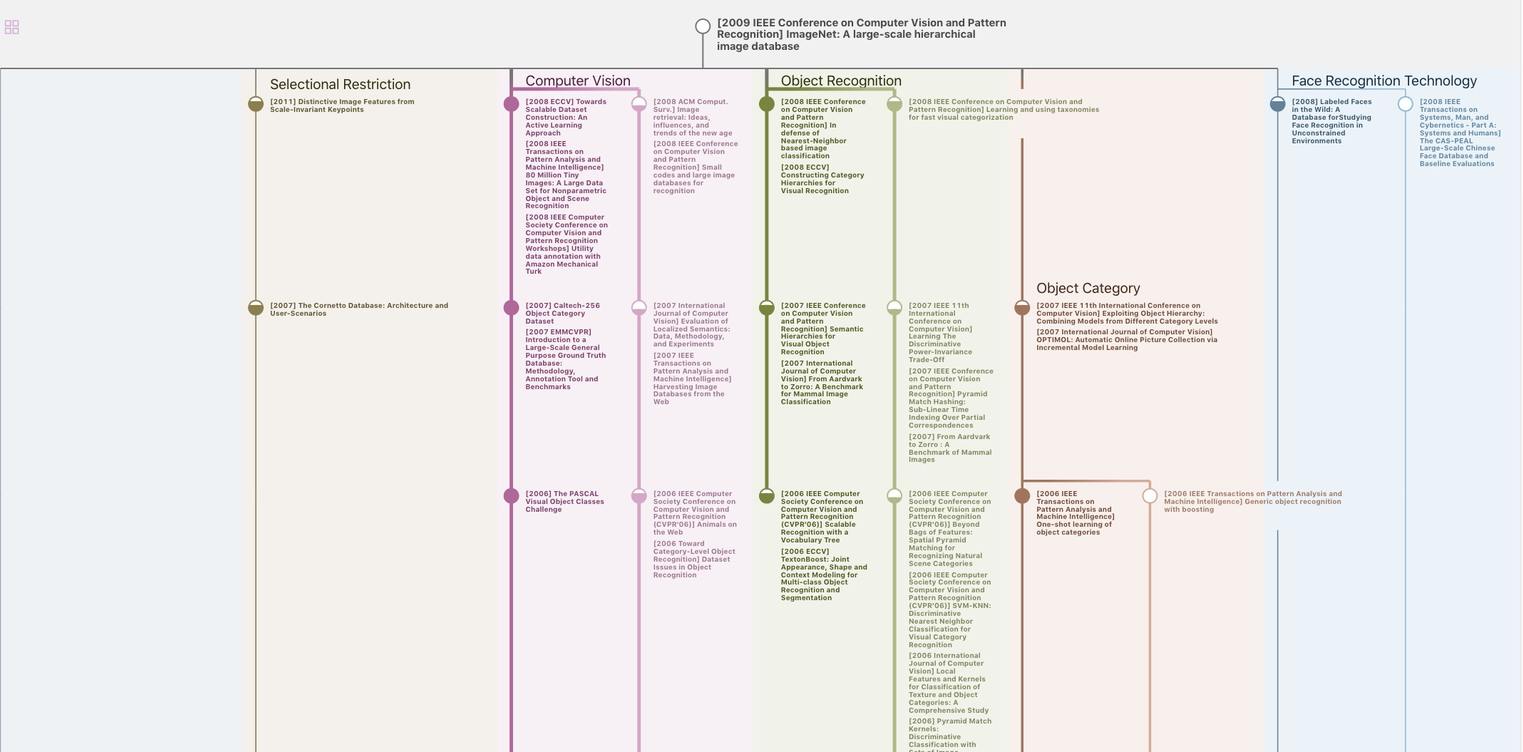
Generate MRT to find the research sequence of this paper
Chat Paper
Summary is being generated by the instructions you defined