Utilizing Mask R-CNN for Automated Evaluation of Diabetic Foot Ulcer Healing Trajectories: A Novel Approach
TRAITEMENT DU SIGNAL(2023)
摘要
The healing trajectory of diabetic foot ulcers (DFUs) is commonly determined through manual inspection, a method which is often subjective and prone to errors. In an effort to address these limitations, this study explores an artificial intelligence-based, computer-aided assessment technique as an alternative. This approach leverages the power of a Hue, Saturation, and Value (HSV)-based image fusion technique, integrating thermal and visual data to deliver precise wound characterizations. Further, through the deployment of an instance segmentation-based Mask Region-based Convolutional Neural Network (Mask-RCNN), the area of the wound is estimated. This randomized, prospective, single-blind study was conducted over a 12-week period, focusing on neuropathic DFUs (Wagner grade 2) located on the plantar aspect of the foot. Forty-two patients were enrolled, with an average age of 54.28 +/- 7.45 years and an average ulcer duration of 5.86 +/- 2.22 years. The healing trajectory of eight patients, observed weekly, was further analyzed. The absolute temperature difference (ATD) between contralateral ulcer regions was found to be 2.63 +/- 1.99 degrees C, with the respective z-score values of ATD providing a significant p-value of 0.000040412 (i.e., p<0.05). The correlation between the ground truth (ulcer area estimation by clinicians using Woundly software) and the proposed method was found to be at an average of 92.50%. The study ultimately concludes that the Mask-RCNN technique, when applied to fused images, can facilitate automated and user-independent assessments of DFUs. This method has the potential to aid in the accurate characterization of healing trajectories, thereby enhancing the overall understanding of wound progression in diabetic patients.
更多查看译文
关键词
healing,r-cnn
AI 理解论文
溯源树
样例
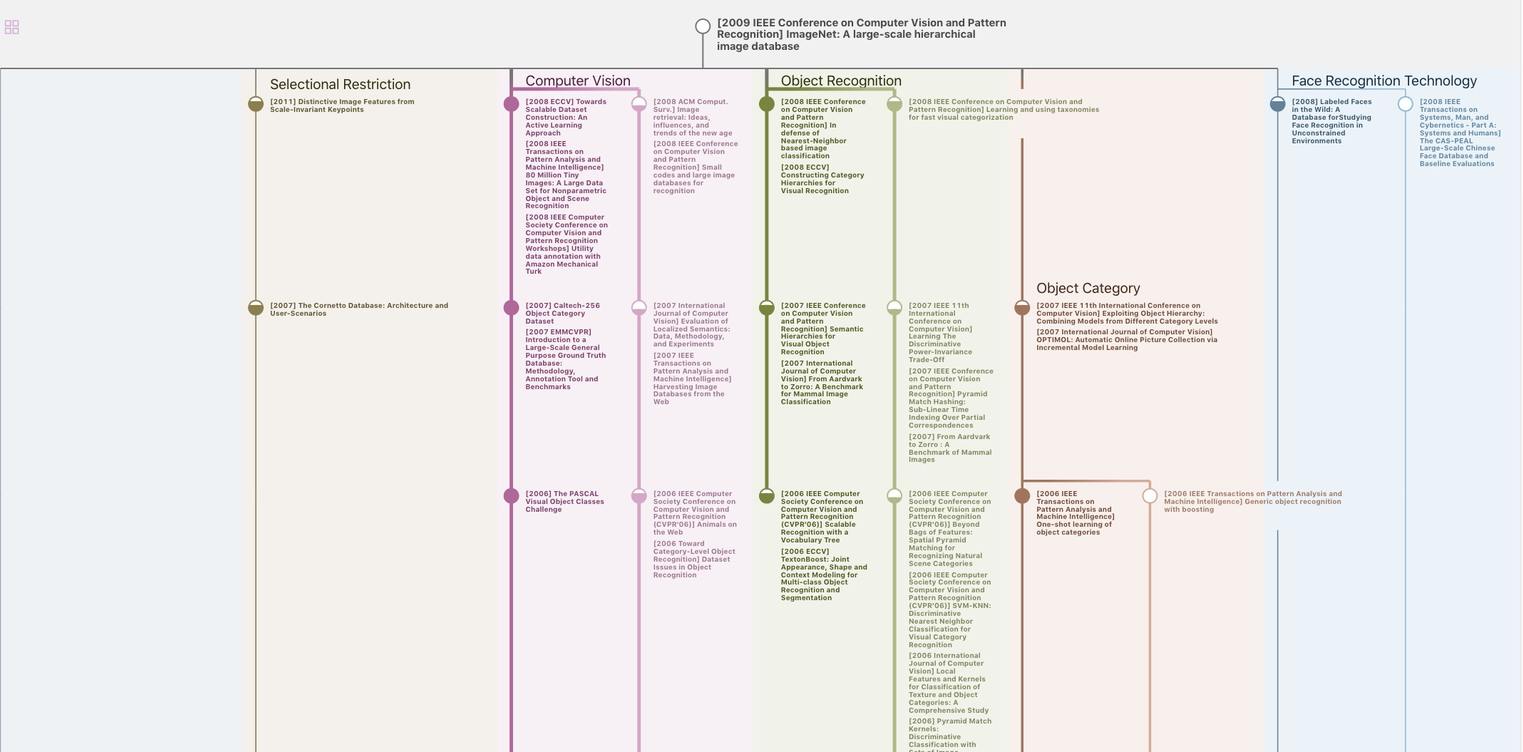
生成溯源树,研究论文发展脉络
Chat Paper
正在生成论文摘要