Deep steerable pyramid wavelet network for unified JPEG compression artifact reduction
SIGNAL PROCESSING-IMAGE COMMUNICATION(2023)
摘要
Although numerous methods have been proposed to remove blocking artifacts in JPEG-compressed images, one important issue not well addressed so far is the construction of a unified model that requires no prior knowledge of the JPEG encoding parameters to operate effectively on different compression-level images (grayscale/color) while occupying relatively small storage space to save and run. To address this issue, in this paper, we present a unified JPEG compression artifact reduction model called DSPW-Net, which employs (1) the deep steerable pyramid wavelet transform network for Y-channel restoration, and (2) the classic U-Net architecture for CbCr-channel restoration. To enable our model to work effectively on images with a wide range of compression levels, the quality factor (QF) related features extracted by the convolutional layers in the QF-estimation network are incorporated in the two restoration branches. Meanwhile, recursive blocks with shared parameters are utilized to drastically reduce model parameters and shared-source residual learning is employed to avoid the gradient vanishing/explosion problem in training. Extensive quantitative and qualitative results tested on various benchmark datasets demonstrate the effectiveness of our model as compared with other state-of-the-art deblocking methods.
更多查看译文
关键词
Compression artifact reduction,Steerable pyramid wavelet transform,Quality factor,Residual learning
AI 理解论文
溯源树
样例
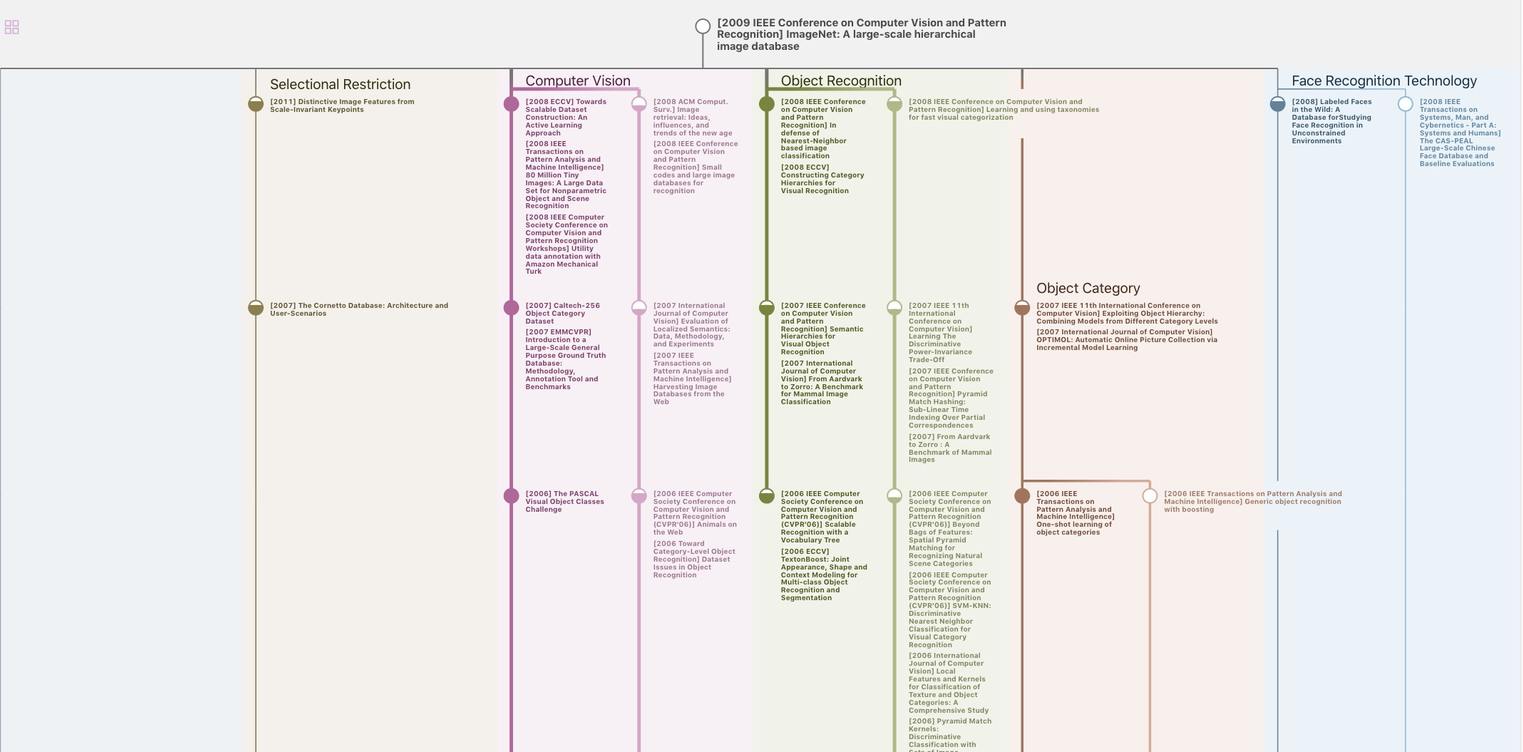
生成溯源树,研究论文发展脉络
Chat Paper
正在生成论文摘要