LCW-Net: Low-light-image-based crop and weed segmentation network using attention module in two decoders
ENGINEERING APPLICATIONS OF ARTIFICIAL INTELLIGENCE(2023)
摘要
Crop segmentation using cameras is commonly used in large agricultural areas, but the time and duration of crop harvesting varies in large farms. Considering this situation, there is a need for low-light image-based segmentation of crop and weed images for late-time harvesting, but no prior research has considered this. As a first study on this topic, we propose a low-light image-based crop and weed segmentation network (LCW-Net) that uses an attention module in two decoders to perform only one step without restoration of low-light images. We also design a loss function to accurately segment regions of objects, crops, and weeds in low-light images to avoid training overfitting and balance the learning task for object, crop, and weed segmentation.There are no existing low-light public databases, and it is difficult to obtain ground truth segmentation information for self-collected database in low-light environments. Therefore, we experimented with converting two public databases, the crop and weed field image dataset (CWFID) and BoniRob dataset, into low-light datasets. The experimental results showed that the mean intersection of unions (mIoUs) of segmentation for crops and weeds were 0.8718 and 0.8693 for the BoniRob dataset, respectively, and 0.8337 and 0.8221 for the CWFID dataset, respectively, indicating that LCW-Net outperforms the state-of-the-art methods.
更多查看译文
关键词
Semantic segmentation for crops and weeds,Low-light image,Attention module in two decoders,LCW-Net
AI 理解论文
溯源树
样例
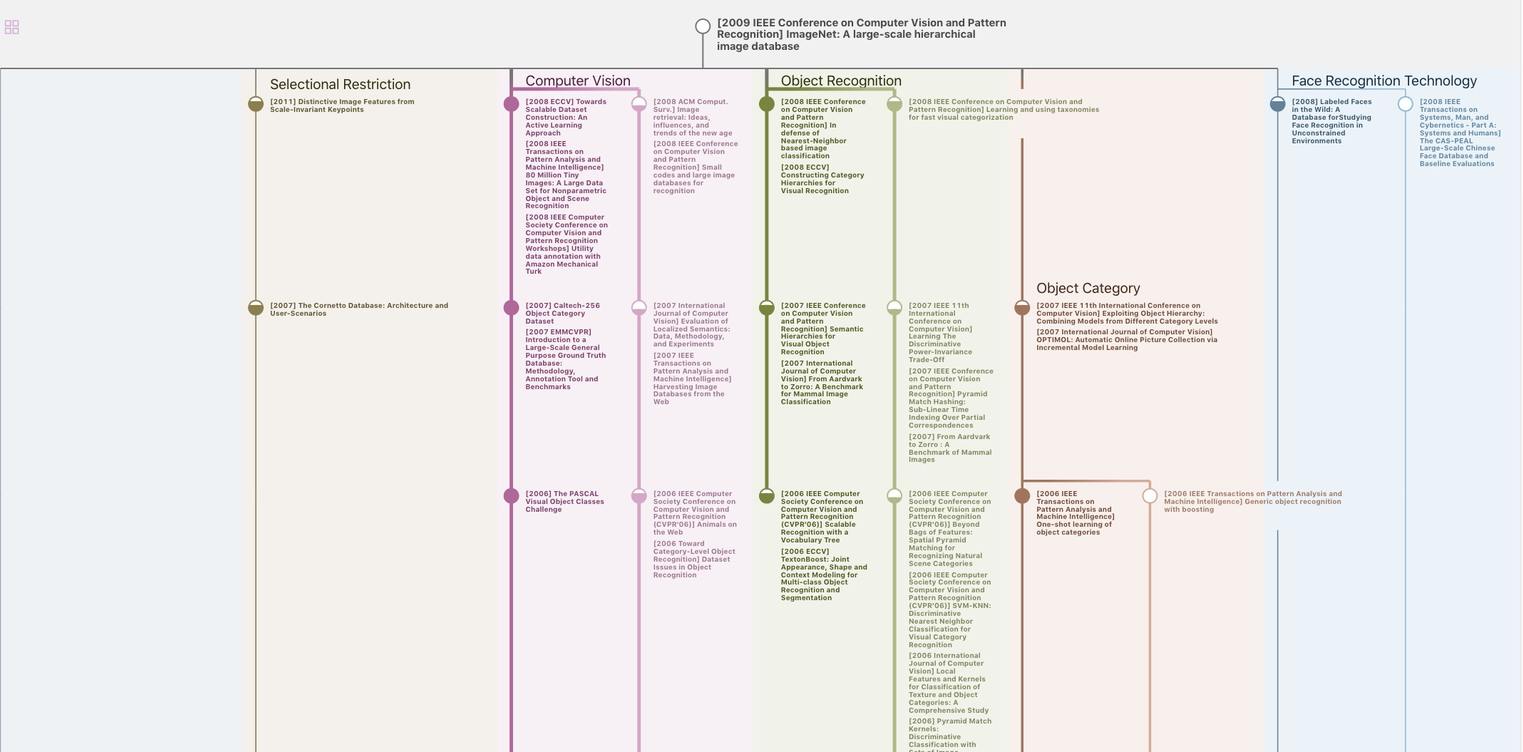
生成溯源树,研究论文发展脉络
Chat Paper
正在生成论文摘要