Maximum angular multiscale entropy: Characterization of the angular self-similarity patterns in two types of SAR images: Oil spills and low-wind conditions images
PHYSICA D-NONLINEAR PHENOMENA(2023)
Abstract
The characterization of irregular patterns in images has become a focal point in numerous studies due to its relevance in various applications. In particular, images depicting natural phenomena exhibit intricate patterns with unique irregularities, such as self-similarity and scaling. The classification of these phenomena based on their patterns has been an ongoing endeavor within the field of complex systems. However, existing techniques for characterizing these complex phenomena typically assume isotropic variability patterns, which is not always feasible. To address these limitations, this paper introduces a novel algorithm, called the maximum angular multiscale entropy (MAMSE), based on the multiscale entropy method. The MAMSE algorithm overcomes the challenges posed by anisotropic fractal properties and is tested on synthetic images featuring both isotropic irregularities and anisotropic fractal properties. The experimental results reveal a new concept of angular self-similarity enabled by MAMSE, which proves instrumental in effectively distinguishing between groups of oil spill and lowwind conditions images. Comparative analysis with the two-dimensional multiscalar entropy algorithm demonstrates the superior performance and efficiency of MAMSE, yielding better and faster results. (c) 2023 Elsevier B.V. All rights reserved.
MoreTranslated text
Key words
Anisotropic imagens,Angular self-similarity,Multiscale entropy,Oil spill
AI Read Science
Must-Reading Tree
Example
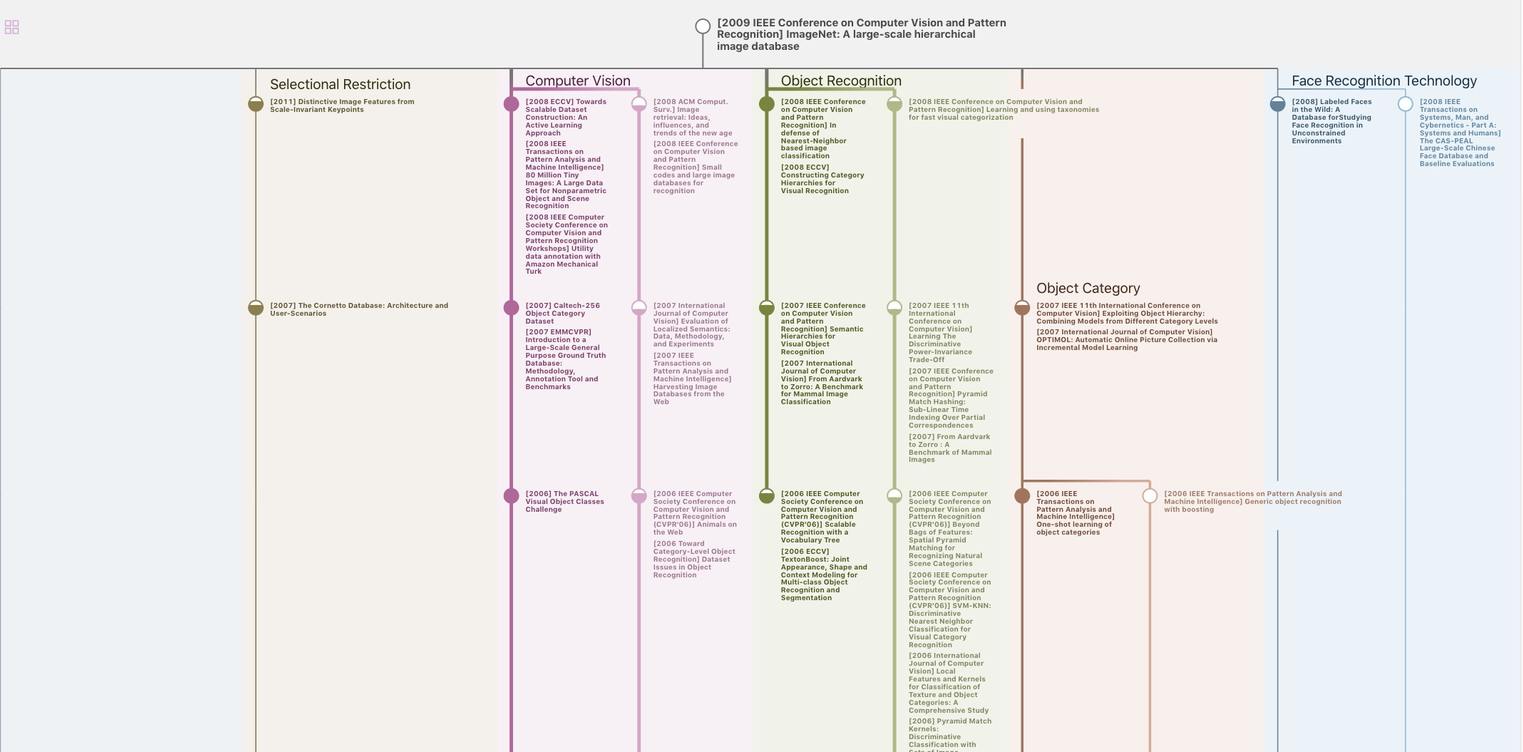
Generate MRT to find the research sequence of this paper
Chat Paper
Summary is being generated by the instructions you defined