A data-driven layout optimization framework of large-scale wind farms based on machine learning
RENEWABLE ENERGY(2023)
摘要
This paper presents a data-driven wind farm layout optimization framework that uses a machine learning wake model that considers physical control stages. The machine learning wake model is trained using well-validated Computational Fluid Dynamics (CFD) simulation data, and consists of thousands of sub-models, each of which is an artificial neural network (ANN) wake model. The ANN wake models are trained separately for low-speed and high-speed inflows to ensure high accuracy of the predictions, with less than 2% error compared to CFD simulations. The accuracy and efficiency of the framework are validated, and the results show better agreement with CFD simulation than an analytical wake model developed in recent years. A parametric study on the number of wind turbines in the Horns Rev wind farm demonstrates that more wind turbines can be added with a minor decrease in average power, with more even and staggered layouts. Under full-wake uniform inflow, the selected analytical wake model exhibits a power prediction error of 5%-8%, while the differences between optimal layouts searched by different wake models range from 2% to 8%. When introducing a wider range of inflow direction sectors, the discrepancy between optimal layout performances decreases.
更多查看译文
关键词
Machine learning,Wake model,Artificial neural networks,Offshore wind farm,Layout optimization
AI 理解论文
溯源树
样例
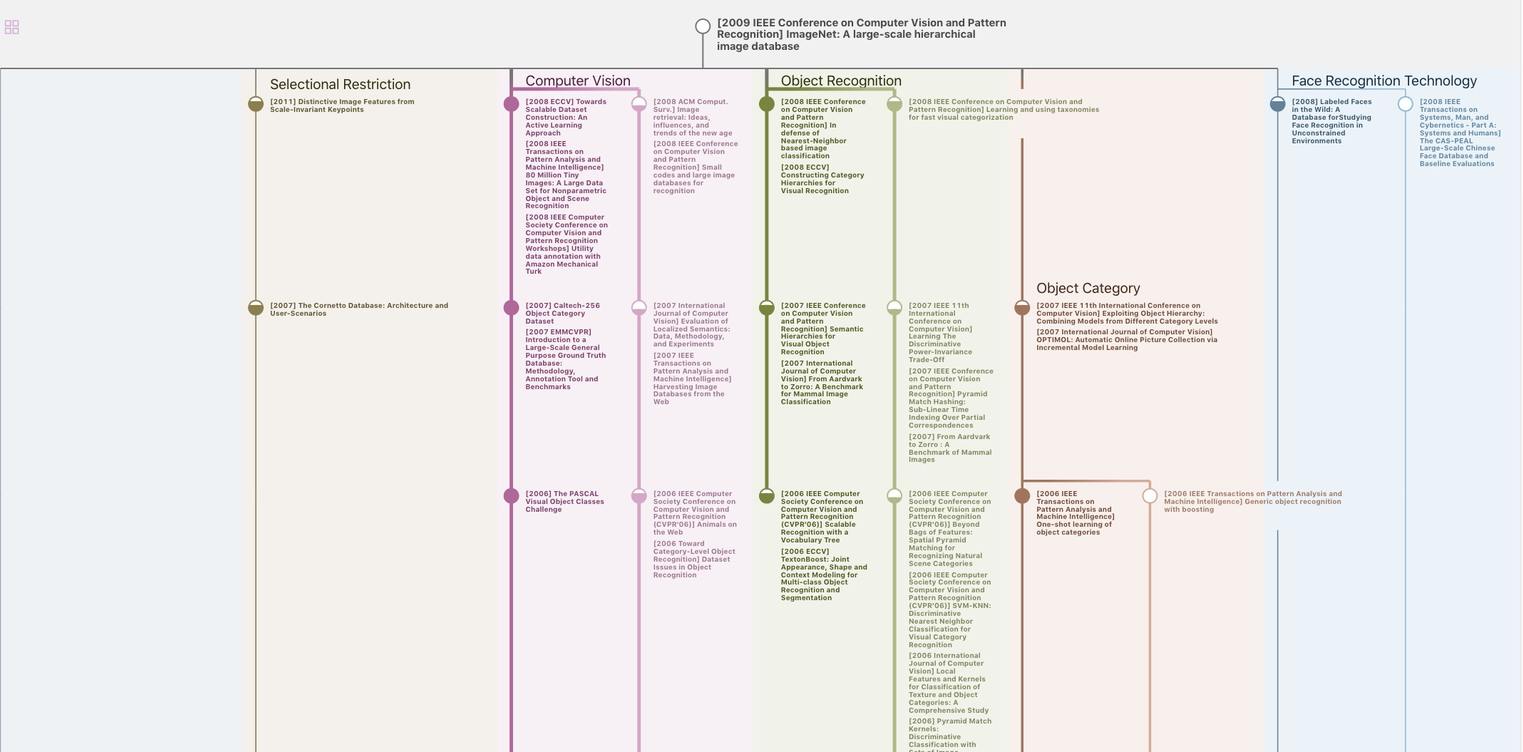
生成溯源树,研究论文发展脉络
Chat Paper
正在生成论文摘要