IoUNet plus plus : Spatial cross-layer interaction-based bounding box regression for visual tracking
IET COMPUTER VISION(2024)
Abstract
Accurate target prediction, especially bounding box estimation, is a key problem in visual tracking. Many recently proposed trackers adopt the refinement module called IoU predictor by designing a high-level modulation vector to achieve bounding box estimation. However, due to the lack of spatial information that is important for precise box estimation, this simple one-dimensional modulation vector has limited refinement representation capability. In this study, a novel IoU predictor (IoUNet++) is designed to achieve more accurate bounding box estimation by investigating spatial matching with a spatial cross-layer interaction model. Rather than using a one-dimensional modulation vector to generate representations of the candidate bounding box for overlap prediction, this paper first extracts and fuses multi-level features of the target to generate template kernel with spatial description capability. Then, when aggregating the features of the template and the search region, the depthwise separable convolution correlation is adopted to preserve the spatial matching between the target feature and candidate feature, which makes their IoUNet++ network have better template representation and better fusion than the original network. The proposed IoUNet++ method with a plug-and-play style is applied to a series of strengthened trackers including DiMP++, SuperDiMP++ and SuperDIMP_AR++, which achieve consistent performance gain. Finally, experiments conducted on six popular tracking benchmarks show that their trackers outperformed the state-of-the-art trackers with significantly fewer training epochs. This paper proposes a novel IoU predictor (IoUNet++) for visual tracking that uses multi-layer fused spatial template features and depthwise separable convolutional correlations to achieve more accurate bounding box estimation. The tracker improved by the IoUNet++ method outperforms state-of-the-art trackers on six popular tracking benchmarks.image
MoreTranslated text
Key words
computer vision,convolutional neural nets,object tracking
AI Read Science
Must-Reading Tree
Example
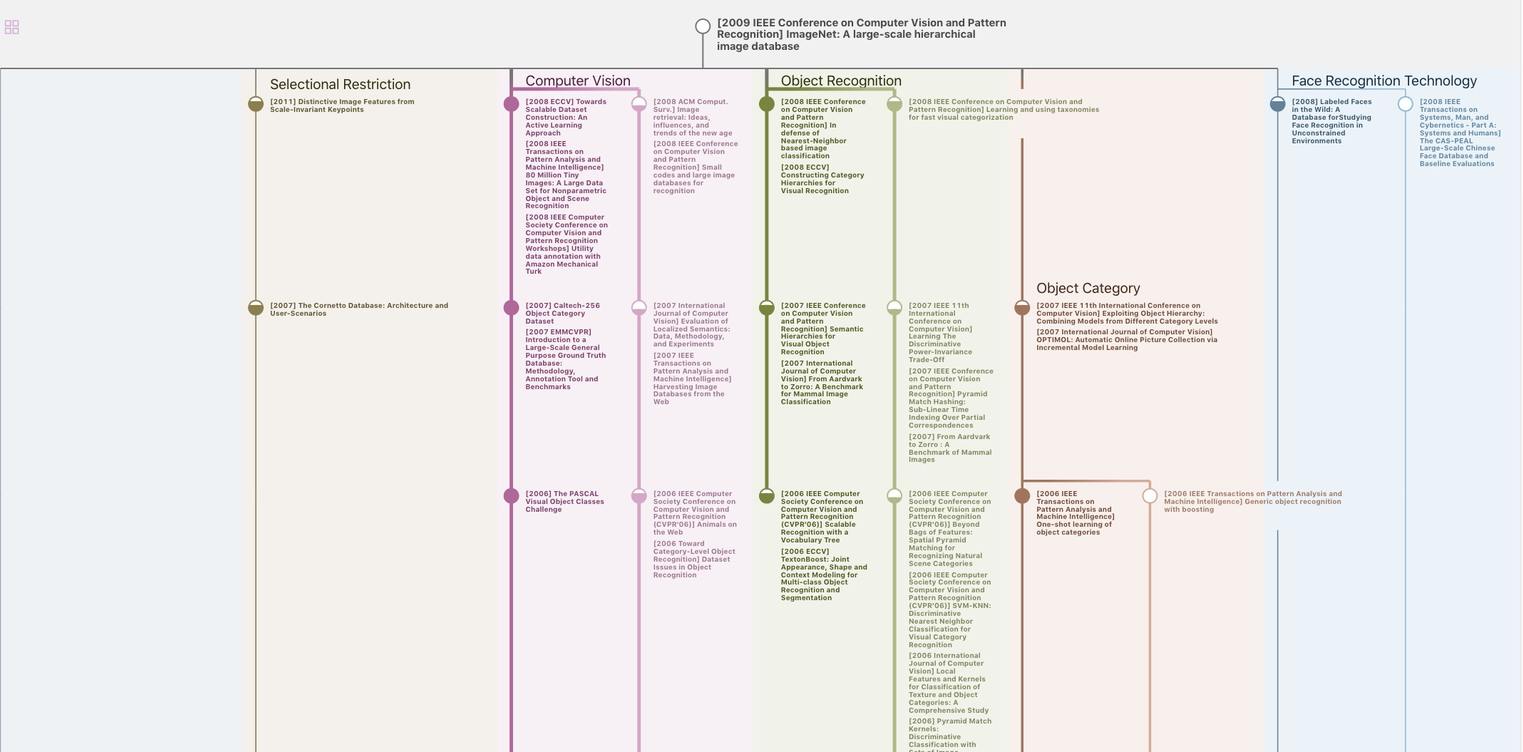
Generate MRT to find the research sequence of this paper
Chat Paper
Summary is being generated by the instructions you defined