A novel state of health estimation method for lithium-ion batteries based on constant-voltage charging partial data and convolutional neural network
ENERGY(2023)
摘要
State-of-health (SOH) estimation is critical for the reliable operation of lithium-ion batteries. Existing methods for manually extracting health features from constant-current charging, constant-voltage (CV) charging, and relaxation phases are limited for practical applications. This study proposes a SOH estimation method for lithium-ion batteries based on partial CV charging phase data and a convolutional neural network (CNN). By analysing the current profile trend with battery aging in the CV charging phase, we propose the estimation of the SOH using partial CV charging data. We suggest adding differential current data as input to extract battery aging information from limited data. Additionally, we design a CNN-based SOH estimation framework that can automatically extract features from current and differential current data in the early stage of CV charging phase, thereby avoiding complex feature engineering. In addition, we devise a transfer learning strategy to improve the model's generalization. A battery dataset comprising three materials is used to validate the proposed method. The results show that the proposed method requires only the first 1000 s of data from the CV charging phase to achieve a highly accurate estimation of the SOH, demonstrating the method's practicality and its excellent generalization for batteries composed of different materials.
更多查看译文
关键词
Lithium-ion battery,State of health,Constant-voltage (CV) charging phase,One dimensional convolutional neural network,Transfer learning
AI 理解论文
溯源树
样例
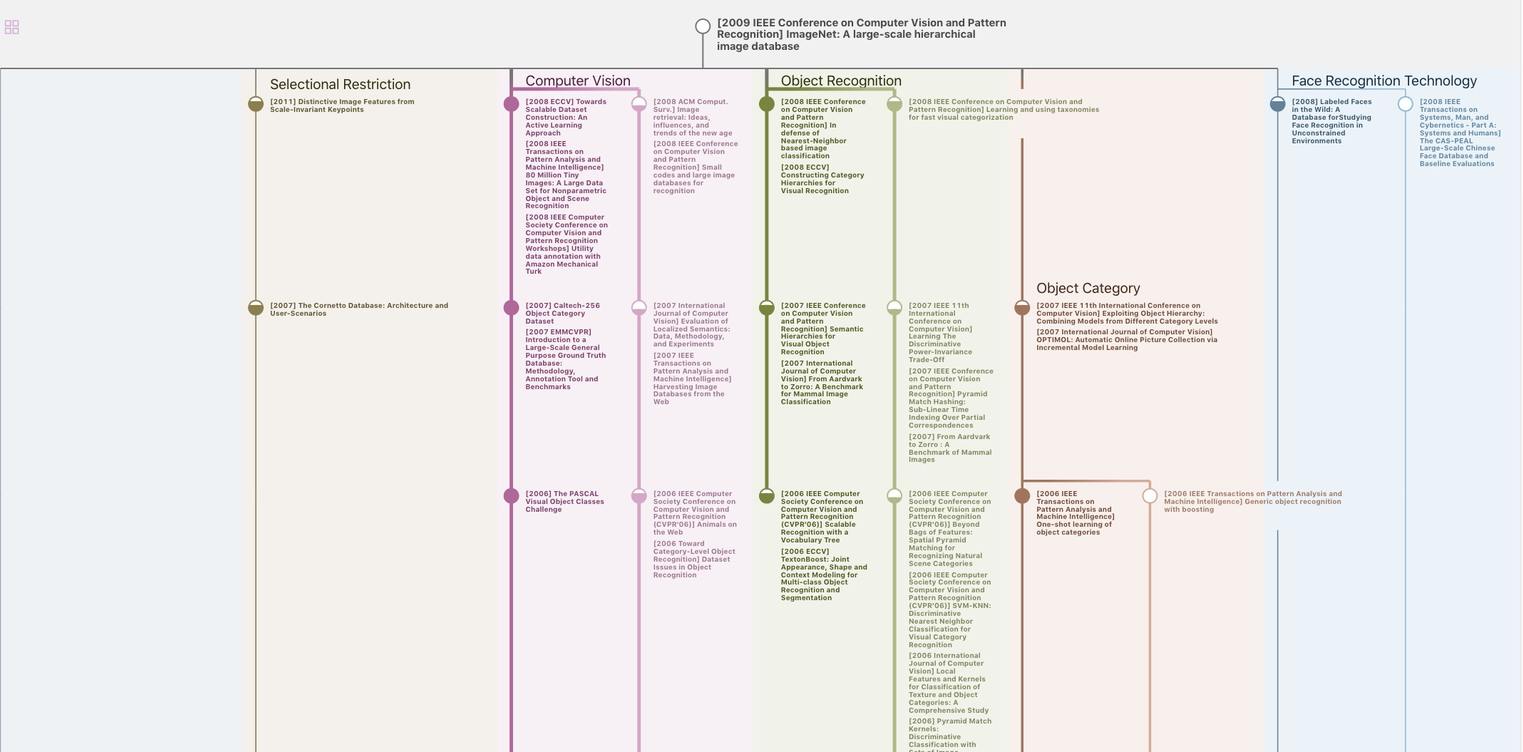
生成溯源树,研究论文发展脉络
Chat Paper
正在生成论文摘要