Compact Model Build Upon Dynamic Weighting Artificial Neural Network Approach for Complementary Field Effect Transistors
IEEE TRANSACTIONS ON ELECTRON DEVICES(2024)
摘要
In this work, a dynamic weighting-artificial neural network (DW-ANN) methodology is presented for quick and automated compact model (CM) generation. It takes advantage of both TCAD simulations for high accuracy and SPICE simulations for cost-effectiveness. This methodology is developed for gate-all-around (GAA) silicon (Si) nanosheet (NS) complementary field effect transistor (CFET), a potential candidate for future CMOS technology due to its innate properties. The critical process variation (PV) sources that severely degrade the CFET performance are estimated using DW-ANN. It reduces the computation cost and predicts the effects of PV sources with less than 1% error. Furthermore, a compact DW-ANN-based Verilog-A model has been developed that captures the dc as well as transient behavior accurately for circuit-level analysis. CFET-based circuits such as inverter, 6T-static random access memory (SRAM), and ring oscillator (RO) are characterized and implemented using DW-ANN-based CM. The overall average error of the model is reported as less than 2%. Therefore, the proposed device and circuit modeling approach provides a feasible solution for the rapid compact modeling of new emerging devices with good convergence and high accuracy.
更多查看译文
关键词
Circuit simulation,compact modeling,complementary FETs,dynamic weights,machine learning,nanosheet (NS),process variation (PV)
AI 理解论文
溯源树
样例
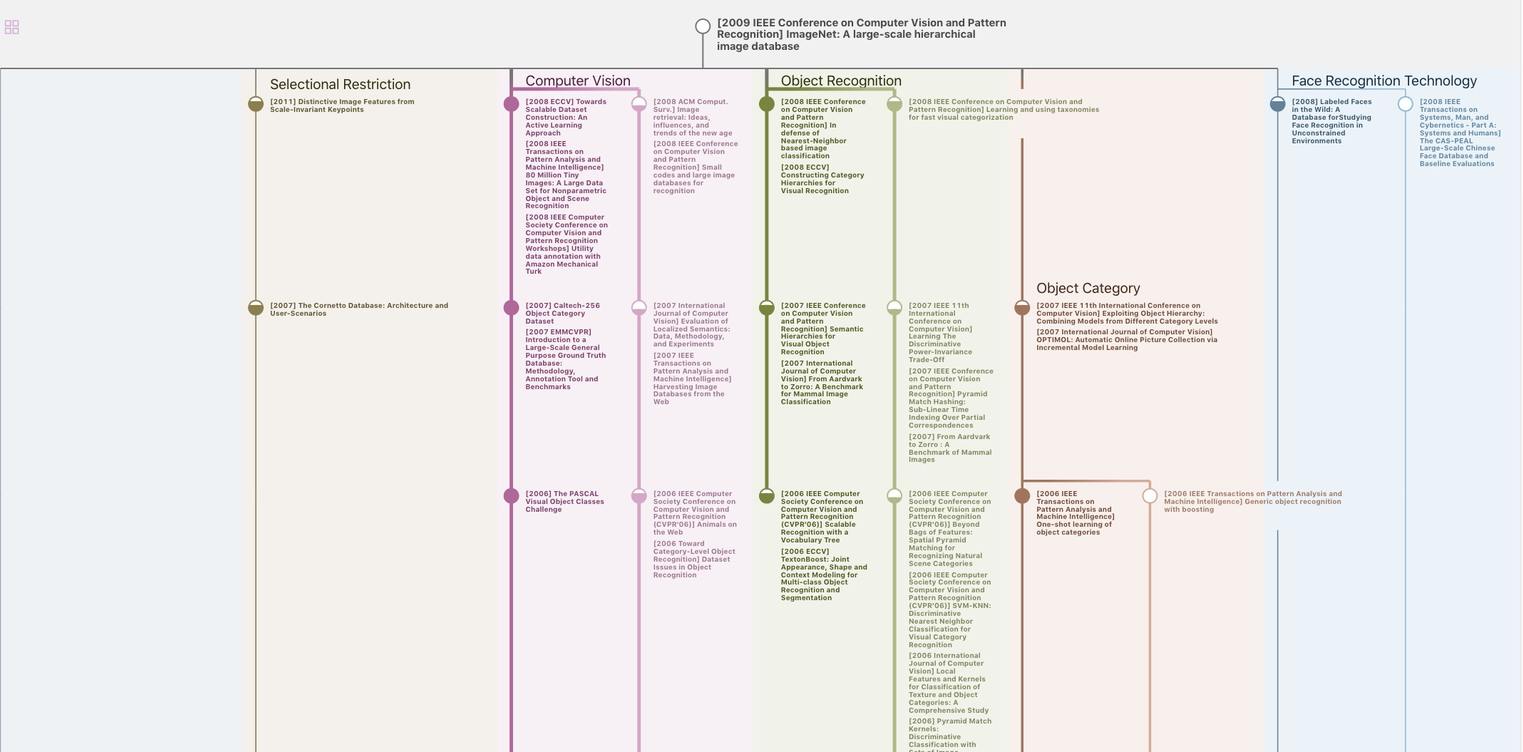
生成溯源树,研究论文发展脉络
Chat Paper
正在生成论文摘要