Surrogate Modeling With Complex-Valued Neural Nets for Signal Integrity Applications
IEEE TRANSACTIONS ON MICROWAVE THEORY AND TECHNIQUES(2023)
Abstract
Neural networks (NNs) are quite attractive in creating surrogate models for many signal integrity (SI) applications. NN-based surrogate models offer the benefits of reducing the design cycle time and providing the designer with a quick prototype that can efficiently analyze the performance of the SI task. This article, therefore, proposes a new end-to-end learning approach for surrogate modeling using complex-valued NNs, incorporating higher functionality and better representation. This approach introduces a deep complex dense network (CDNet), which is built with complex dense blocks to support complex operations using complex-valued weights, and a physically consistent layer to enforce passivity and causality constraints. We also present a robust inverse multiobjective optimization method to minimize the modeling error and optimize the design space parameters. The results show that our model outperforms state-of-the-art deep surrogate models when tasked with forward and inverse learning for a relatively small amount of data. The effectiveness of the proposed approach is demonstrated through two SI design applications, where the model is used to predict broadband S -parameters and obtain optimal design space parameters given the desired target specifications.
MoreTranslated text
Key words
Design space exploration,inverse design,neural networks (NNs),packaging,passivity,signal integrity (SI),surrogate modeling
AI Read Science
Must-Reading Tree
Example
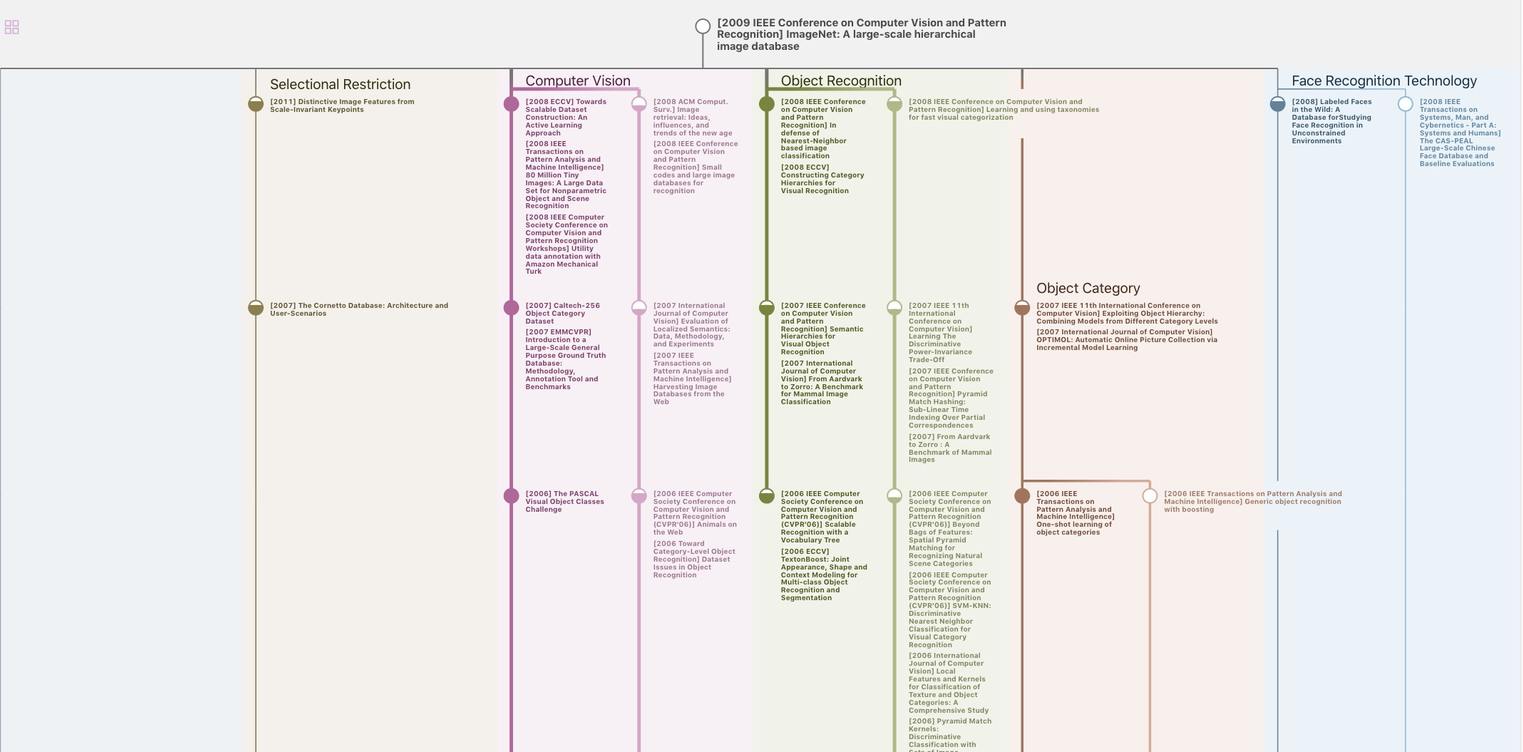
Generate MRT to find the research sequence of this paper
Chat Paper
Summary is being generated by the instructions you defined