Automatic recognition of surface defects of hot rolled strip steel based on deep parallel attention convolution neural network
MATERIALS LETTERS(2023)
Abstract
In general, the existence of surface defects in hot rolled strip steel will seriously affect the quality and appearance of industrial products. In order to improve the classification efficiency and accuracy of surface defect recognition. Firstly, a lightweight and high-precision deep learning neural network, deep parallel attention convolutional neural network, is proposed in this paper. In this network, fusion attention module is combined with parallel multi-scale module to focus important feature information and capture context feature information without losing important feature information. Secondly, in order to verify the lightweight of the proposed model, the Parameters, FLOPs, Inference time and FPS of the deep parallel attention convolutional neural network are compared with the current popular classification networks. The experimental results show that the deep parallel attention convolutional neural network can be applied to the actual steel defect detection. The experimental results show that the recognition accuracy, precision and area under the curve of the deep parallel attention convolutional neural network model reach 99.57%, 99.6% and 99.8%, respectively. This method significantly improves the average accuracy of classification and is an effective tool for detecting steel defects.
MoreTranslated text
Key words
Artificial Intelligence,Defects,Deep Parallel Attention Convolutional Neural Network,Attention Mechanism,Multi-scale integration
AI Read Science
Must-Reading Tree
Example
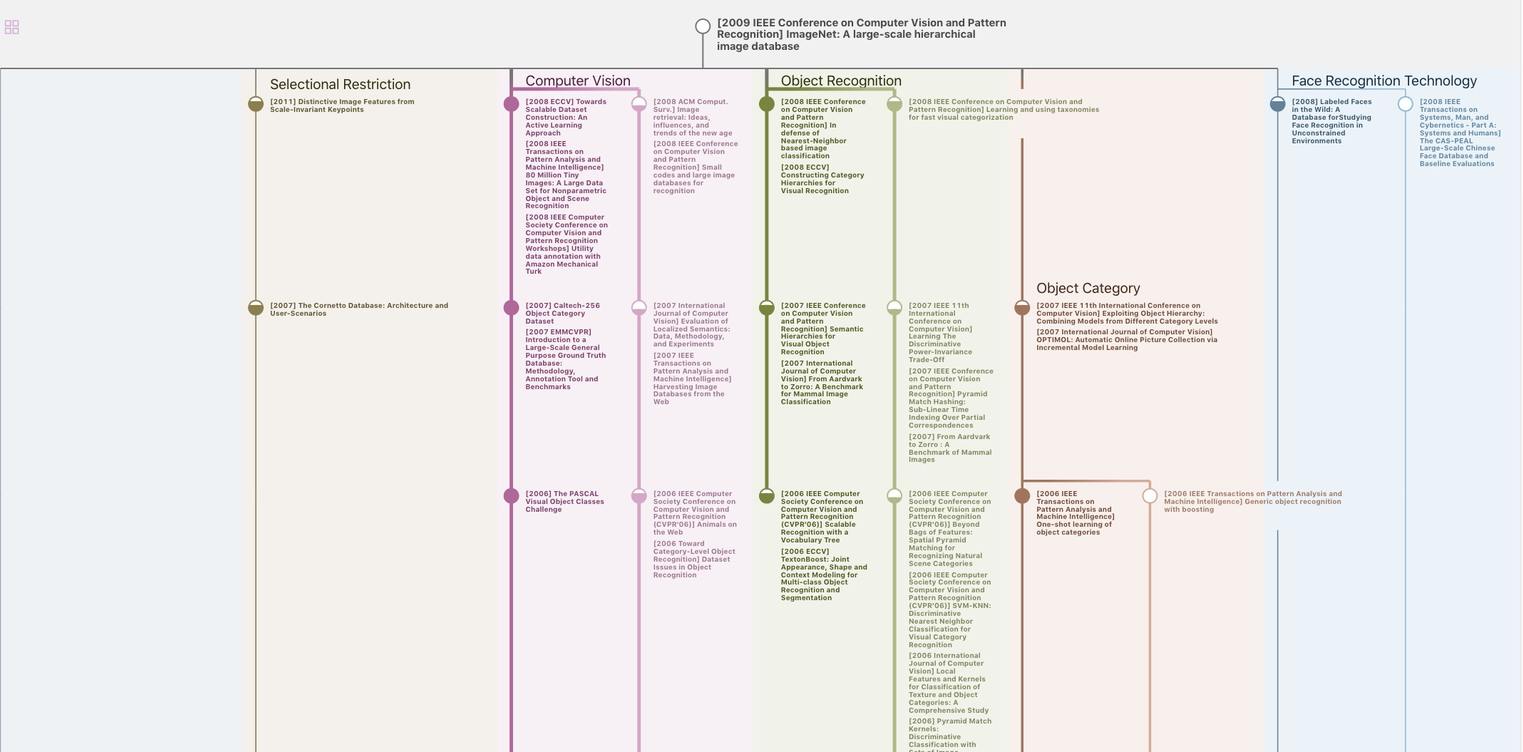
Generate MRT to find the research sequence of this paper
Chat Paper
Summary is being generated by the instructions you defined