Unsupervised Domain Adaptation for Source Localization Using Ships of Opportunity With a Deep Vertical Line Array
IEEE JOURNAL OF OCEANIC ENGINEERING(2024)
摘要
Deep-learning source localization methods trained on synthetic replicas calculated by acoustic propagation models (referred to as model-based source localization methods via deep learning) suffer from environmental mismatches between replicas and measurements, which degrade the localization performance. This article introduces deep transfer learning (DTL), specifically the unsupervised domain adaptation (UDA) and fine-tuning methods for source localization using ships of opportunity (SOO) to alleviate the influence of mismatched replicas. First, the range estimation network is trained using simulated replicas and unlabeled SOO data. Subsequently, the parameters of the ranging network are transferred to a depth estimation network and fine-tuned accordingly. Simulation results demonstrate that the proposed method (UDA-DTL) exhibits greater robustness to array tilt and sound speed profile mismatches in the direct arrival zone compared to the Bartlett processor and the ResNet estimator. Experimental data from the South China Sea also verified the validity of the proposed UDA-DTL estimator. The results demonstrate that supplementing model-based supervised learning with unlabeled SOO, the impact of unknown uncertainty on the localization performance is alleviated. This study highlights the potential to utilize unlabeled Big Data in applications of underwater source localization.
更多查看译文
关键词
Deep ocean,deep transfer learning (DTL),passive localization,ships of opportunity (SOO),unsupervised domain adaptation (UDA)
AI 理解论文
溯源树
样例
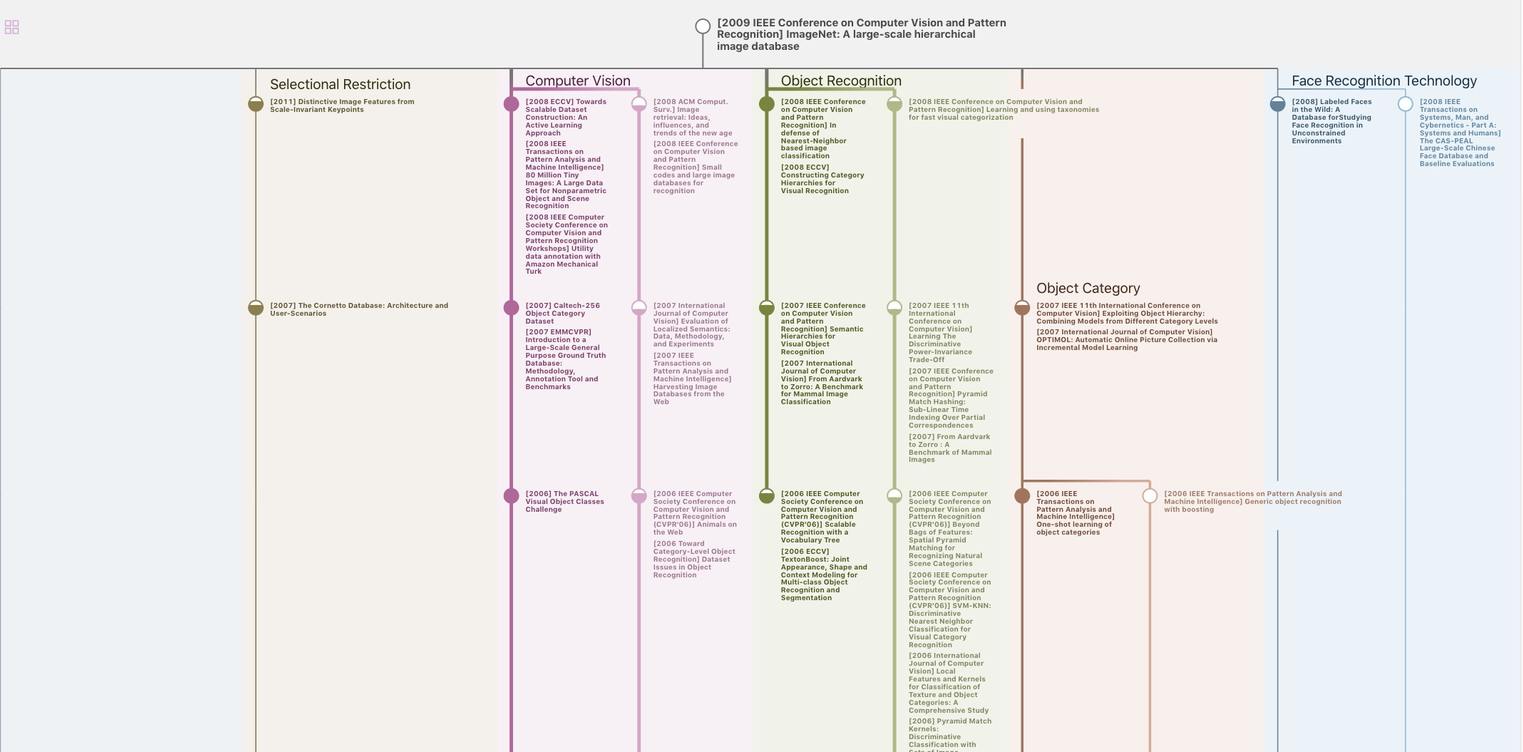
生成溯源树,研究论文发展脉络
Chat Paper
正在生成论文摘要