Robust Q-learning-based multi-objective sheep flock optimizer with a Cauchy operator for effective path planning in unmanned aerial vehicles
INTERNATIONAL JOURNAL OF COMMUNICATION SYSTEMS(2024)
摘要
Unmanned aerial vehicle (UAV) path planning can be treated as a nondeterministic polynomial (NP) hard concern or an optimization problem. The conventional approaches are unable to effectively handle these issues due to discontinuity, non-linearity, multi-modality, and inseparability. On the other hand, meta-heuristic algorithms are effective at tackling these issues because they are simple, adaptable, and derivation free. To enhance the performance in a variety of challenging circumstances, this paper proposes a novel Q-learning-based multi-objective sheep flock optimizer with a Cauchy operator (Q-MOSFO-CA) to solve the constrained UAV path planning issues. The multi-objective functions considered here are costs and constraints (threat, terrain, turning, climbing, and gliding constraints) to determine the feasible and optimal path. To avoid the probability of falling into the local optimum and to address the shortcoming of unbalanced convergence and also to maintain the exploitation and exploration capability, the Cauchy operator (CA) is integrated with the sheep flock optimization (SFO) algorithm. The Q-learning model is introduced to balance both the global and local searches. Here, the exploration model performs the global search whereas the exploitation model performs the local search to attain an optimal solution. In the simulation scenario, the statistical analysis is conducted under two scenarios, and some essential measures such as the number of iterations at convergence (NIC), evaluation time (ET), energy consumption, and convergence analysis are determined. The proposed method obtains NIC of 1305 and 1436, ET of 12.8 and 15.2 s, and energy consumption of 20,600 and 21,465 J for both Scenarios 1 and 2, respectively. A novel technique (Q-MOSFO-CA) for determining efficient UAV path planning is introduced to ensure the vehicle's safety more accurately. To propose a multi-objective sheep flock optimization with Cauchy operator (MOSFO-CA) technique, multi-objective constraints like flight length, altitude cost, terrain, threat, and path length are optimized under low iterations. To achieve effective path planning, Q-learning-based reinforcement learning techniques are introduced to balance the global and local searches.image
更多查看译文
关键词
Cauchy operator,global optimization,path planning,Q-learning model,sheep flock optimization,unmanned aerial vehicle
AI 理解论文
溯源树
样例
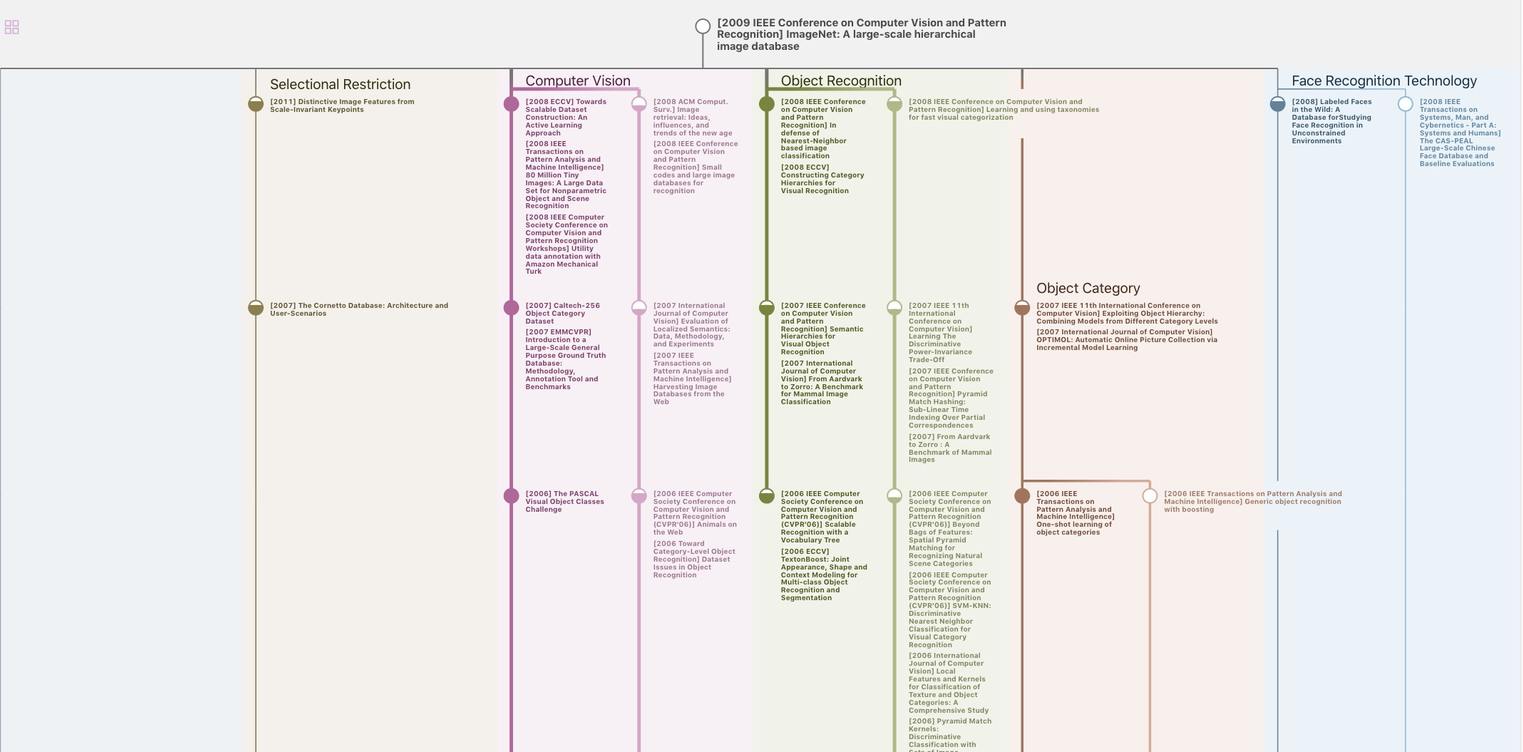
生成溯源树,研究论文发展脉络
Chat Paper
正在生成论文摘要