Physics-informed ensemble deep learning framework for improving state of charge estimation of lithium-ion batteries
JOURNAL OF ENERGY STORAGE(2023)
摘要
With the advances in computer science, deep learning (DL) has been developed for battery management systems (BMSs) with artificial intelligence. State of charge (SOC) estimation of lithium-ion batteries is the fundament and core of BMS, and improving the accuracy, robustness and generalization of model predictions is still challenging. Herein, this paper proposes a physics-informed ensemble deep learning (PIEDL) framework to enable the physical information introduction and multi-model integration. Firstly, a battery simplified electrochemical model (SEM) is used to quickly extract the physical information related to the battery SOC. Subsequently, the open-circuit voltage and reaction polarization resistance from the SEM are integrated as key physical information into the DL model and combined with the original input variables to construct the physics-informed deep learning (PIDL) part of the framework. Then, DL models improved with different techniques are used as base learners for the ensemble deep learning (EDL). At the second level of the EDL, a meta learner is used to integrate multiple heterogeneous base models based on the blending strategy without any weight calculation. The results show that PIEDL outperforms all base models and all models with fewer input variables, and improves the result by more than 60 % relative to the original model with original inputs. Finally, the generalization of the trained model is validated using different battery types. The PIEDL framework is not only important for improving the performance and application scope of BMS, but also provides new ideas and methods for the field of DL.
更多查看译文
关键词
Lithium-ion batteries,State of charge estimation,Physics-informed learning,Ensemble deep learning,Electrochemical model
AI 理解论文
溯源树
样例
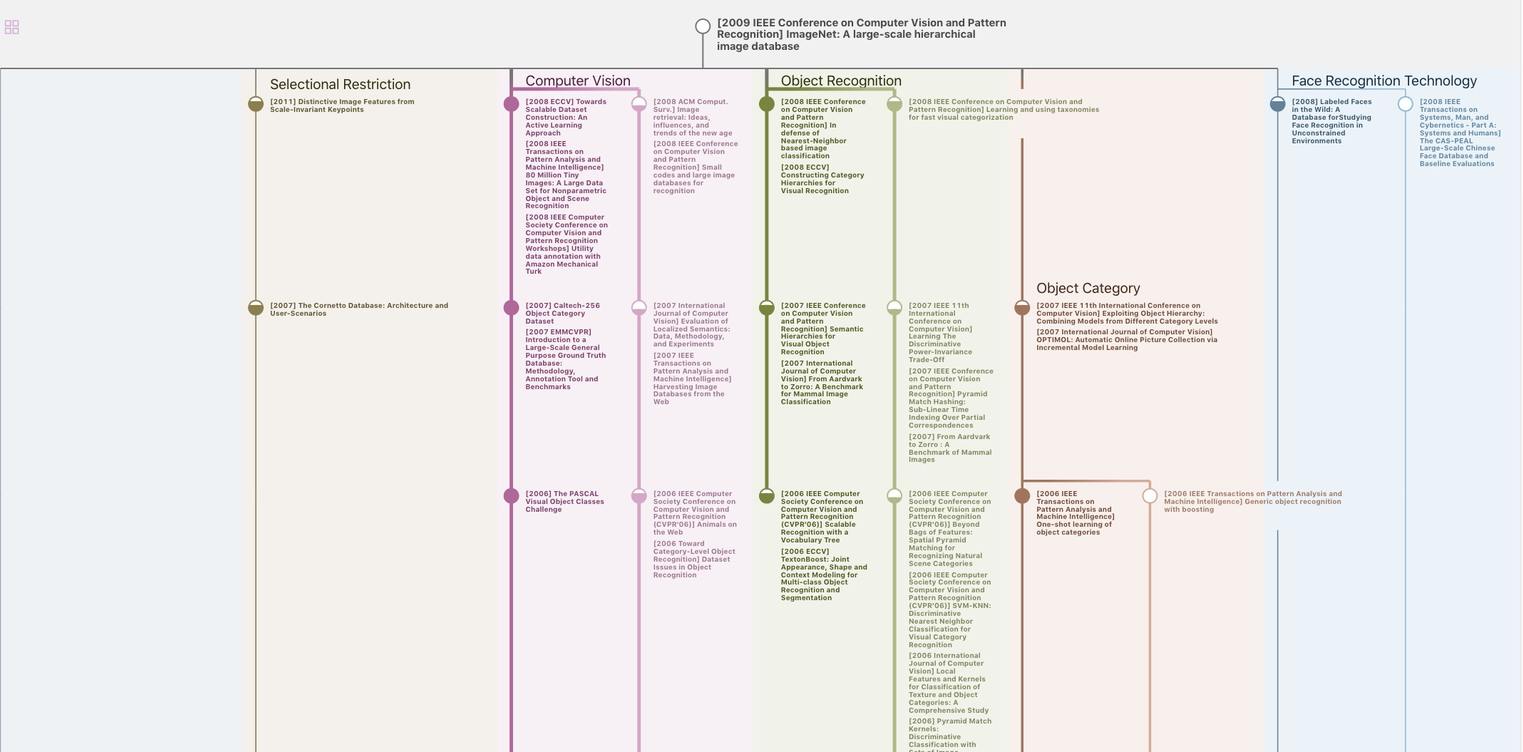
生成溯源树,研究论文发展脉络
Chat Paper
正在生成论文摘要