An Elastic Expandable Fault Diagnosis Method of Three-Phase Motors Using Continual Learning for Class-Added Sample Accumulations
IEEE TRANSACTIONS ON INDUSTRIAL ELECTRONICS(2023)
摘要
Continual learning is promising in intelligent fault diagnosis of three-phase motors, which allows diagnosis networks to increase diagnosable fault classes without tedious retraining during various mechanical and electrical fault sample accumulations. Existing studies, however, have the following limitations. 1) The representation learning ability of diagnosis networks is nonaugmentable because of predefined and fixed network structures. 2) The impacts of different working conditions are not explicitly considered during continual learning. To overcome the abovementioned limitations, an elastic expandable continual learning framework is proposed for class-added motor fault diagnosis. First, an elastic expansion mechanism is developed to improve the representation learning ability during continual learning by progressively introducing new network branches. In addition, the new branches are pruned concurrently with training to elastically adjust the network sizes, thereby avoiding bringing excessive computational complexity. Then, an adaptive multiscale convolution mechanism is designed to learn robust fault features by fusing working condition information, increasing the diagnostic accuracy of continual learning under complex working conditions. Finally, a new training strategy with a multiobjective loss is formulated to guide continual learning. The effectiveness of the proposed method is demonstrated through motor fault simulation experiments.
更多查看译文
关键词
Adaptive multiscale convolution (AMSC),continual learning,elastic expansion mechanism,intelligent fault diagnosis,three-phase motor
AI 理解论文
溯源树
样例
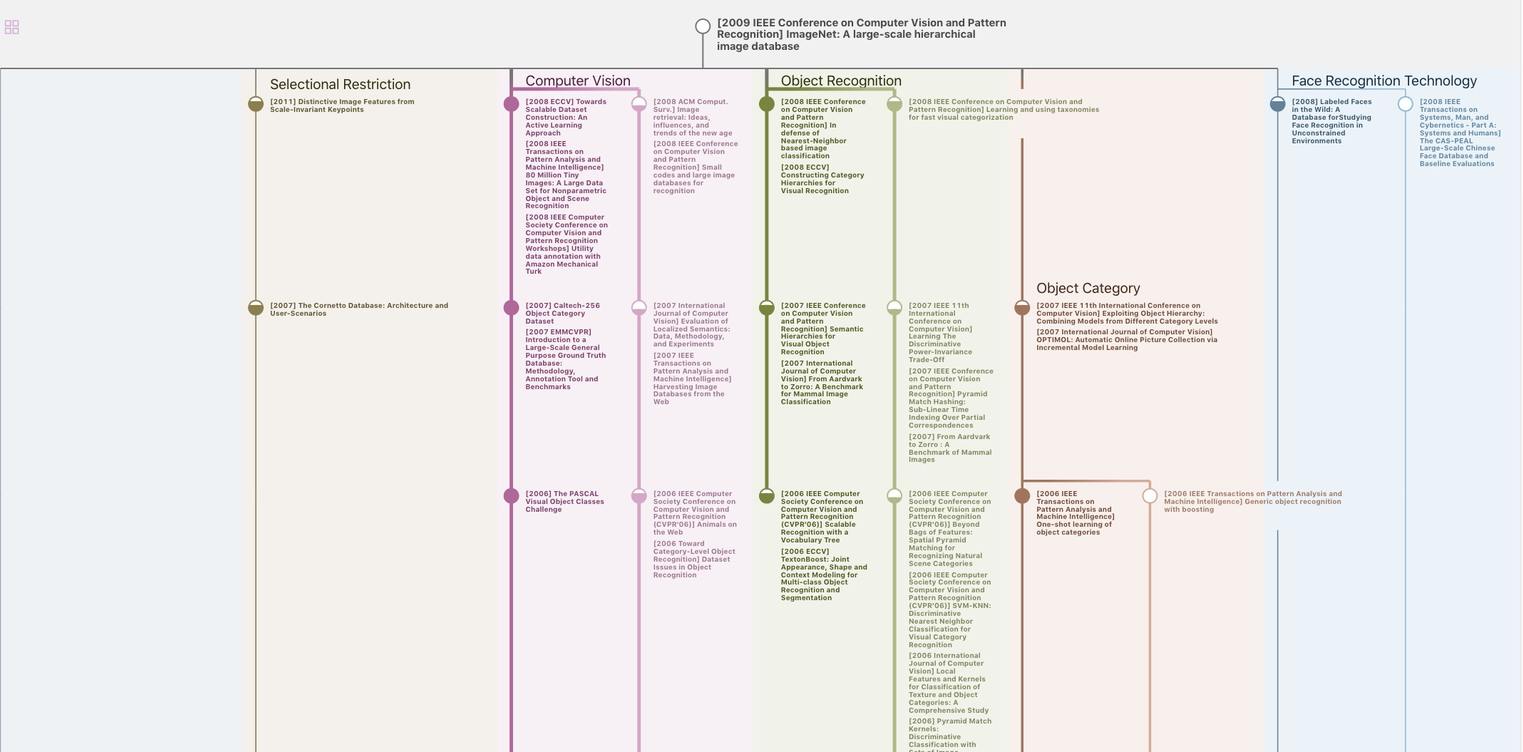
生成溯源树,研究论文发展脉络
Chat Paper
正在生成论文摘要