Revealing the Covariation of Atmospheric O2 and Pollutants in an Industrial Metropolis by Explainable Machine Learning
ENVIRONMENTAL SCIENCE & TECHNOLOGY LETTERS(2023)
摘要
In urban areas, atmospheric O-2 actively participates in the process of anthropogenic emissions and energy consumption. However, the covariation between atmospheric O-2 and the emitted pollutants has yet to be thoroughly explored. This study examines the covariations between atmospheric O-2 and pollutants in Lanzhou, a semi-arid industrial metropolis. A machine learning (ML)-based O-2 simulator coupled with a SHapley Additive exPlanation (SHAP) algorithm is established to explore and interpret their covariations under diverse conditions. Our findings indicate an increase of 16.3 ppm in the O-2 concentration associated with the atmospheric transport of natural dust particles during dusty weather events. This suggests that natural dust transport can mitigate the depletion of atmospheric O-2 caused by primary emissions and secondary formation of anthropogenic particulate matter. Furthermore, we identify a nonlinear relationship between the concentrations of O-2 and pollutant concentrations, which likely arises from their distinct diffusive abilities. The study highlights the unique pollution characteristics in a semi-arid urban downtown and demonstrates the ability of ML-based methodology to reproduce and interpret the environmental and anthropogenic impacts on the local carbon-oxygen balance.
更多查看译文
关键词
urban O-2 observations, explainable machinelearning, air pollution, anthropogenic impact, emission inventory
AI 理解论文
溯源树
样例
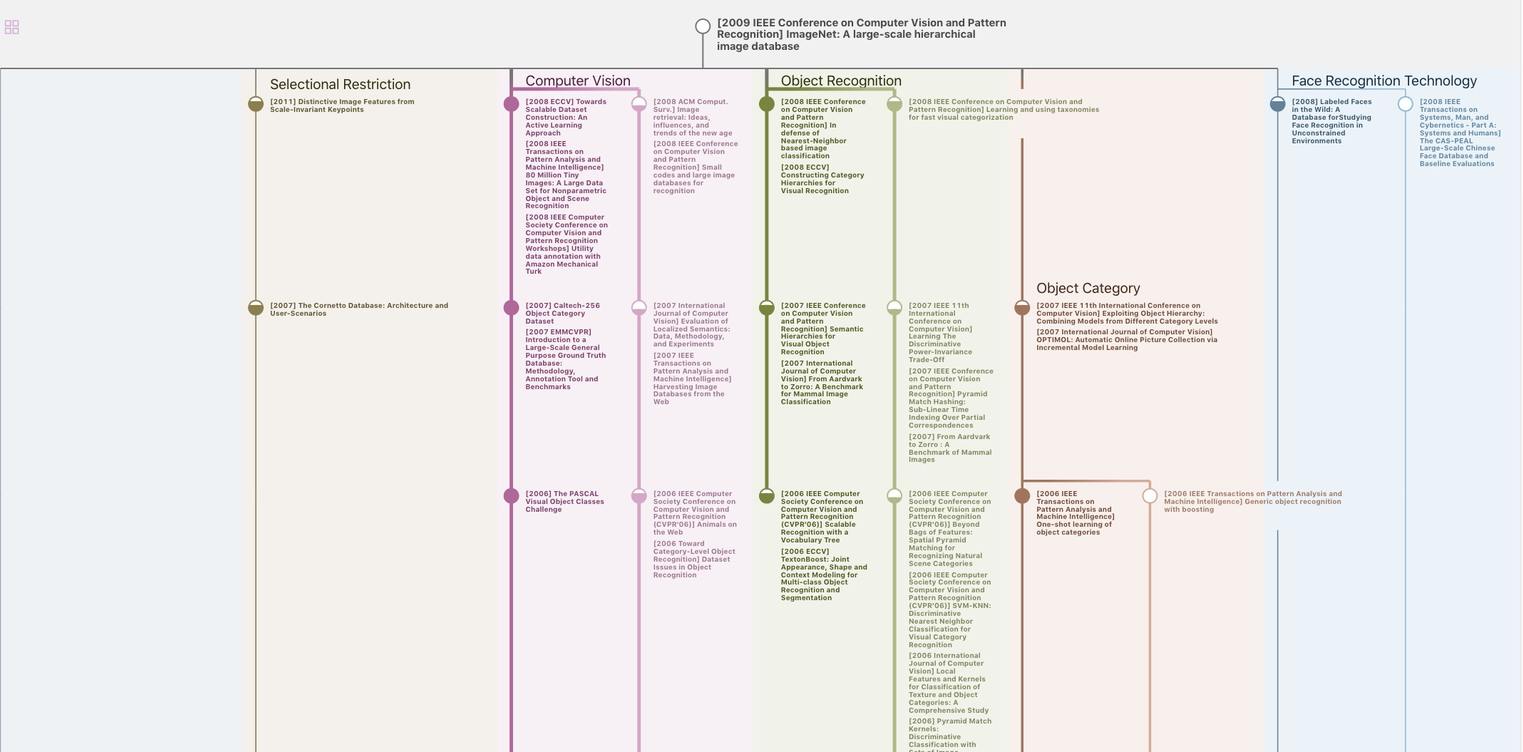
生成溯源树,研究论文发展脉络
Chat Paper
正在生成论文摘要