A Novel Fuzzy Unsupervised Feature Learning Approach
INTERNATIONAL JOURNAL OF COMPUTATIONAL INTELLIGENCE AND APPLICATIONS(2023)
摘要
The effectiveness of machine learning approaches depends on the quality of the data representation. In fact, some representations may mislead such learning approaches upon concealing relevant explanatory variables. Although feature engineering, that utilizes domain knowledge and/or expert supervision, yields typical data representation techniques, generic unsupervised feature learning represents an even more objective alternative to determine relevant attributes and generate optimal feature spaces. In this paper, we propose a new fuzzy unsupervised feature learning approach (FUL) that automatically derives new features by revealing the intrinsic structure of the data. In fact, FUL exploits the clusters and the associated fuzzy memberships generated by a fuzzy C-means algorithm, and devises new basis functions and their corresponding representation. The experiments results showed that FUL overtakes relevant state of the art approaches. It yielded the highest F1-measure with an improvement of 8%, 11%, 3%, and 4% on Parkinson, Epilepsy, Gait, and breast cancer datasets, respectively.
更多查看译文
关键词
Unsupervised feature learning,dictionary learning,sparse representation,fuzzy C-means
AI 理解论文
溯源树
样例
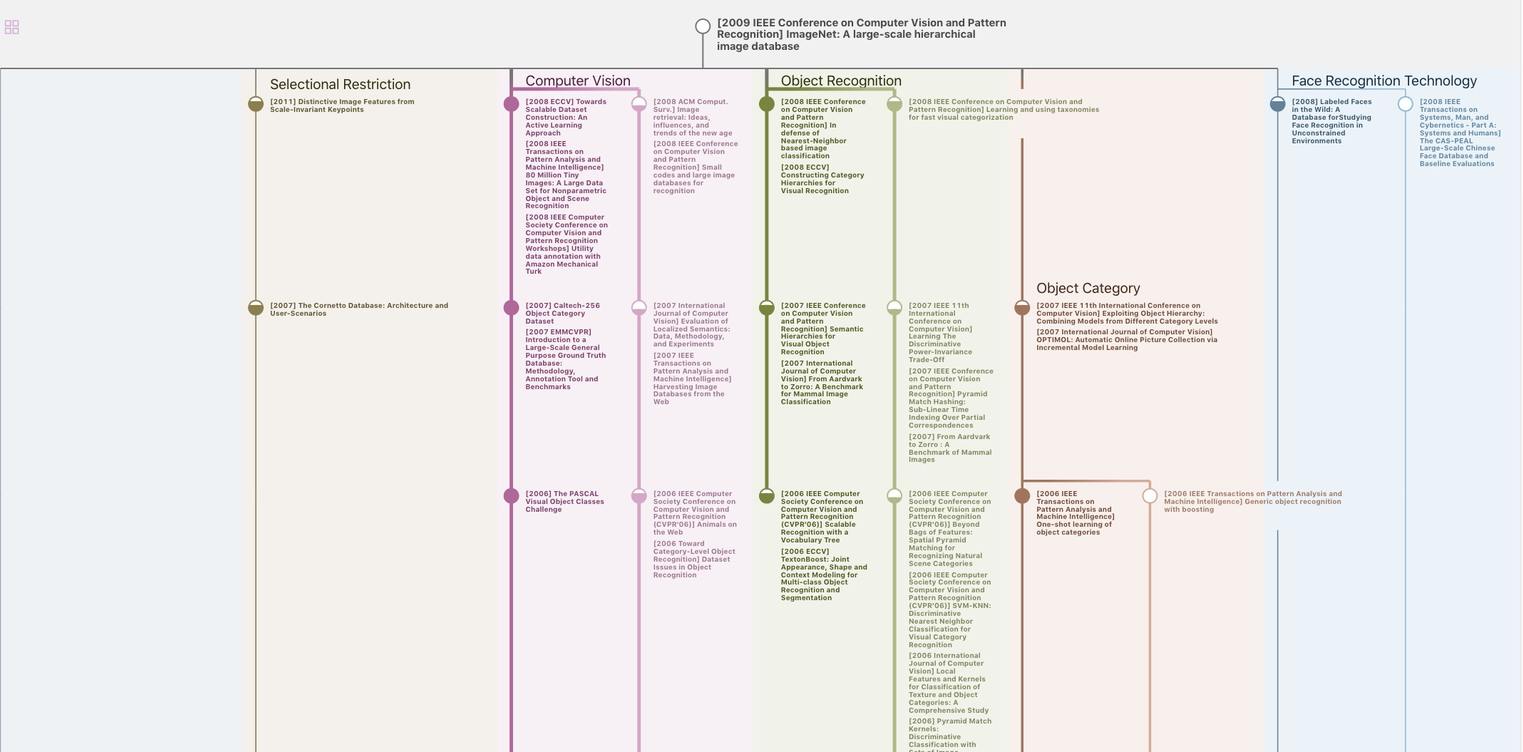
生成溯源树,研究论文发展脉络
Chat Paper
正在生成论文摘要