Breast lesions segmentation and classification in a two-stage process based on Mask-RCNN and Transfer Learning
MULTIMEDIA TOOLS AND APPLICATIONS(2023)
摘要
The most prevalent malignancy of concern among women is breast cancer. Early detection plays a crucial role in improving survival chances. However, the current reliance on mammography for breast cancer detection has limitations due to the delicate nature of breast tissue, human visual system constraints, and variations in accumulated experience, leading to significant false positive and false negative results. This study aims to minimize these errors by developing an intelligent computer-based diagnosis method for breast cancer utilizing digital mammography, employing the Transfer Learning approach. The proposed technique involves two stages. In the first stage, we fine-tune the pre-trained Mask R-CNN model on the COCO dataset to identify and segment breast lesions. In the second stage, various convolutional Deep Learning models such as ResNet101, ResNet34, VGG16, VGG19, AlexNet, and DenseNet121 classify the segmented lesions as benign or malignant. The lesion detection and segmentation stages achieved an average precision of 96.26%, while breast lesion classification using the DenseNet121 model obtained 99.44% accuracy on the INbreast dataset. An additional benefit of this study is the development of a new dataset extracted from the INbreast dataset, containing solely lesion images. This novel dataset reduces storage capacity and computational complexity during Deep neural network training and testing as it avoids the use of entire images. Moreover, the lesion dataset holds potential for use in breast cancer diagnosis research and may be integrated into an advanced computer-assisted diagnostic system for breast cancer screening.
更多查看译文
关键词
Breast Cancer,Lesion Detection and Classification,Deep Learning,Transfer Learning,Mask-RCNN
AI 理解论文
溯源树
样例
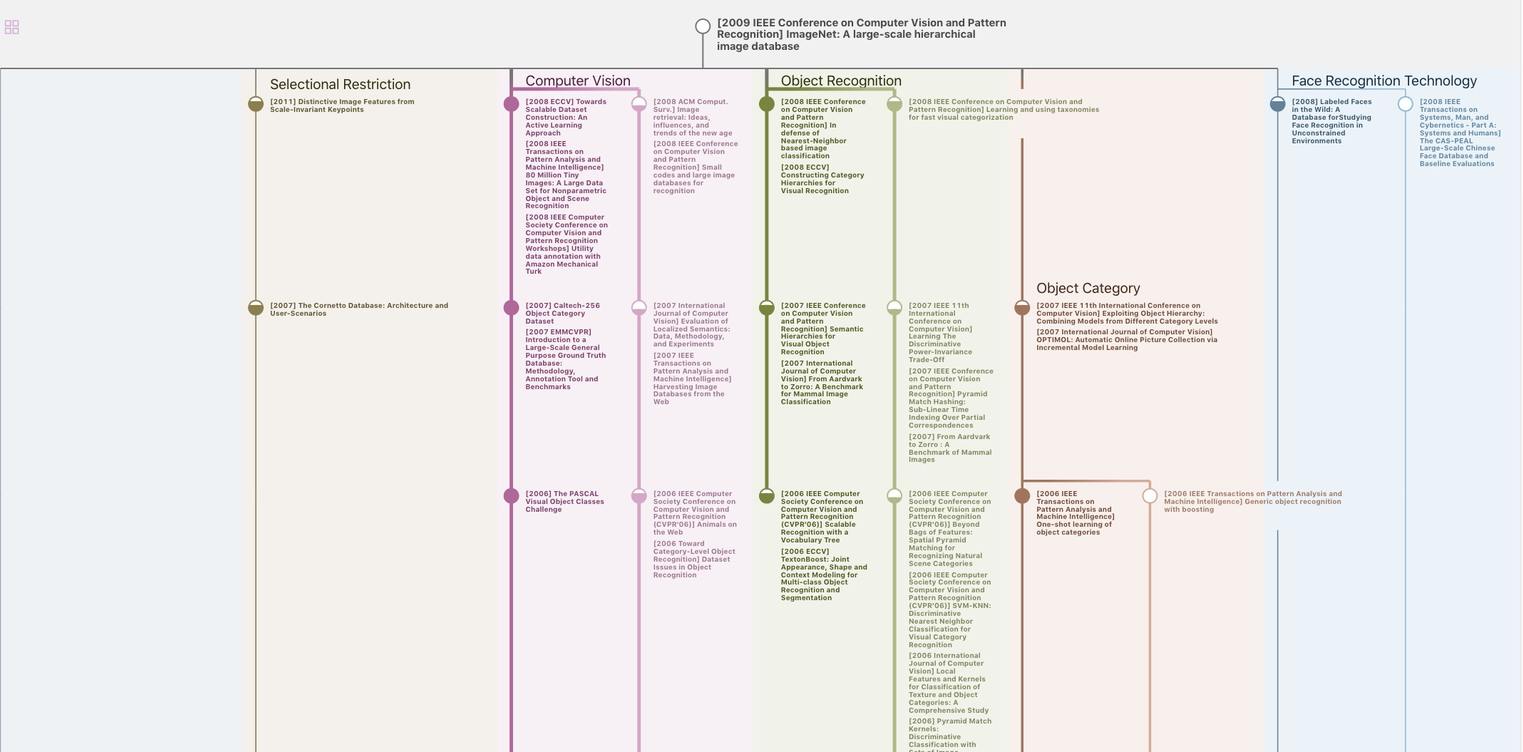
生成溯源树,研究论文发展脉络
Chat Paper
正在生成论文摘要