Automatic phase compensation of a continuous-variable quantum-key-distribution system via deep learning
PHYSICAL REVIEW A(2023)
摘要
Ina practical continuous-variable quantum-key-distribution (CVQKD) system, it is vital to accurately evaluate and then compensate for the phase drifts of the signals, so that the involved system can achieve better performance and stability. In this paper, based on the long short-term memory network (LSTM) model, an automatic phase compensation approach of the CVQKD system is proposed. The LSTM model is first trained to predict the phase drift value of the quantum signal relative to the local oscillator over time. Then, the predicted phase drift value can be used by Alice to reconstruct her data. Finally, Alice and Bob can obtain the raw key, so that the CVQKD system can achieve enhancements in terms of performance and stability. The experimental results indicate that the proposed LSTM-based automatic phase compensation algorithm can accurately predict the phase drift value and perform phase compensation instead of real-time phase drift measurement, which improves the performance of the CVQKD system without requiring any additional quantum resources and extra experimental hardware.
更多查看译文
关键词
automatic phase compensation,deep learning,quantum-key-distribution quantum-key-distribution,continuous-variable
AI 理解论文
溯源树
样例
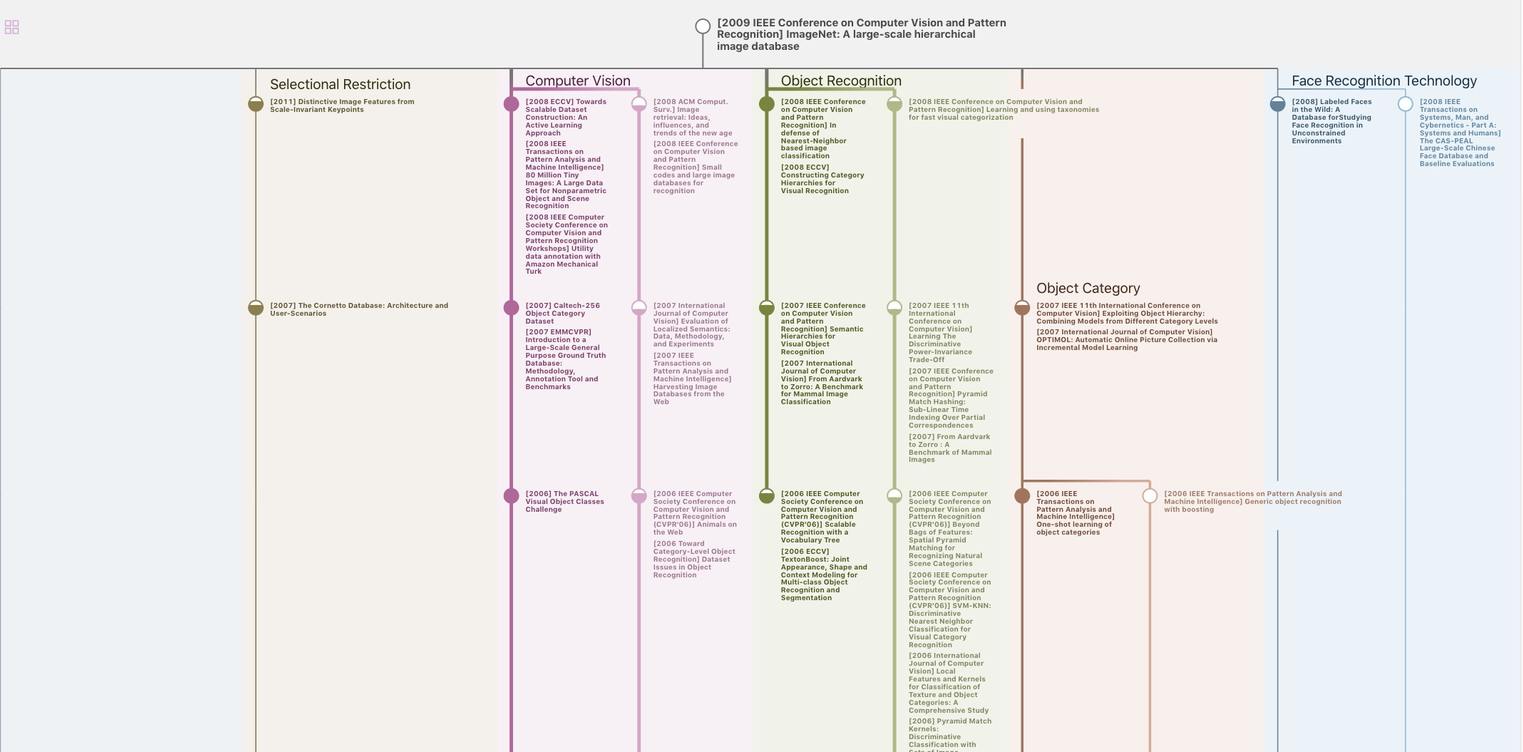
生成溯源树,研究论文发展脉络
Chat Paper
正在生成论文摘要