Imbalanced data classification using improved synthetic minority over-sampling technique
MULTIAGENT AND GRID SYSTEMS(2023)
Abstract
In data mining, deep learning and machine learning models face class imbalance problems, which result in a lower detection rate for minority class samples. An improved Synthetic Minority Over-sampling Technique (SMOTE) is introduced for effective imbalanced data classification. After collecting the raw data from PIMA, Yeast, E.coli, and Breast cancer Wisconsin databases, the pre-processing is performed using min-max normalization, cleaning, integration, and data transformation techniques to achieve data with better uniqueness, consistency, completeness and validity. An improved SMOTE algorithm is applied to the pre-processed data for proper data distribution, and then the properly distributed data is fed to the machine learning classifiers: Support Vector Machine (SVM), Random Forest, and Decision Tree for data classification. Experimental examination confirmed that the improved SMOTE algorithm with random forest attained significant classification results with Area under Curve (AUC) of 94.30%, 91%, 96.40%, and 99.40% on the PIMA, Yeast, E.coli, and Breast cancer Wisconsin databases.
MoreTranslated text
Key words
Data cleaning,imbalanced data,min max normalization,random forest,synthetic minority oversampling technique,transformation
AI Read Science
Must-Reading Tree
Example
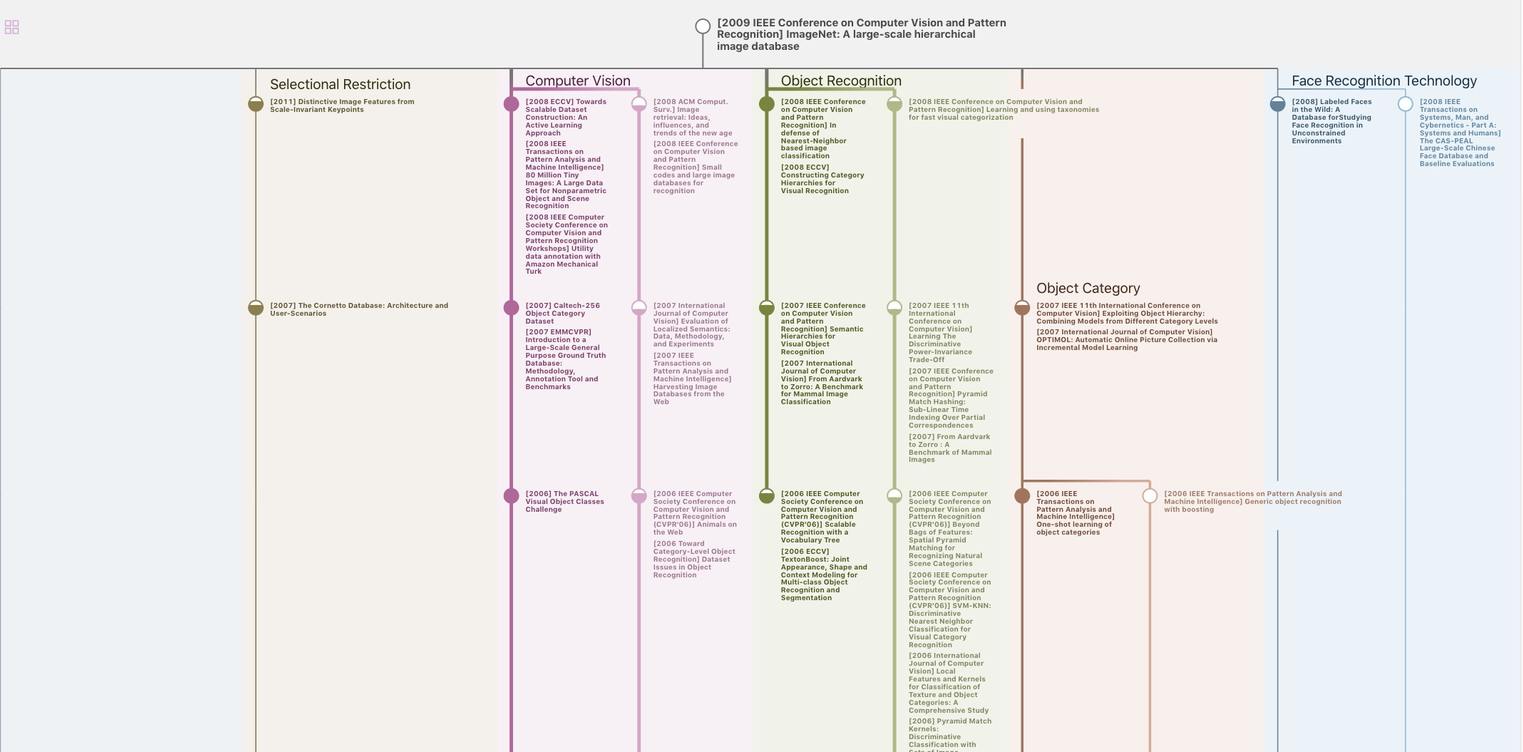
Generate MRT to find the research sequence of this paper
Chat Paper
Summary is being generated by the instructions you defined