Semantics-preserved Graph Siamese Representation Learning
INFORMATION PROCESSING & MANAGEMENT(2023)
摘要
Currently, the expressive power of momentum-driven graph Siamese representation learning is mainly constrained by flawed positive selection strategies and data augmentation strategies. To solve these issues, we propose a Semantics-preserved Graph Siamese Representation Learning method named SGSRL. Firstly, we propose a local structure-aware positive selection strategy to reduce the generation of false positive samples. Specifically, the local structural similarity of nodes is obtained through the comparison between corresponding sampled subgraphs, where the anchor node's subgraph is pooled to simplify the calculation. In addition, we propose a class information-aware feature augmentation method. Compared with directly modifying the inputs of the original graph, augmentation in the feature space is more controllable and intuitive. Specifically, we design an auxiliary contrastive learning task to train a class vector generator, and then overlay the class-oriented augmentation vectors generated by this generator onto the randomly augmented feature vectors. This joint feature augmentation strategy increases the variance between positive samples without changing the semanticity of nodes. To validate the superiority of our method, we conduct experiments on five real graph datasets for both node classification and node clustering tasks. The results demonstrate that our proposed SGSRL always outperforms state-of-art baselines.
更多查看译文
关键词
Momentum-driven graph siamese representation learning,Graph positive selection,Graph feature augmentation
AI 理解论文
溯源树
样例
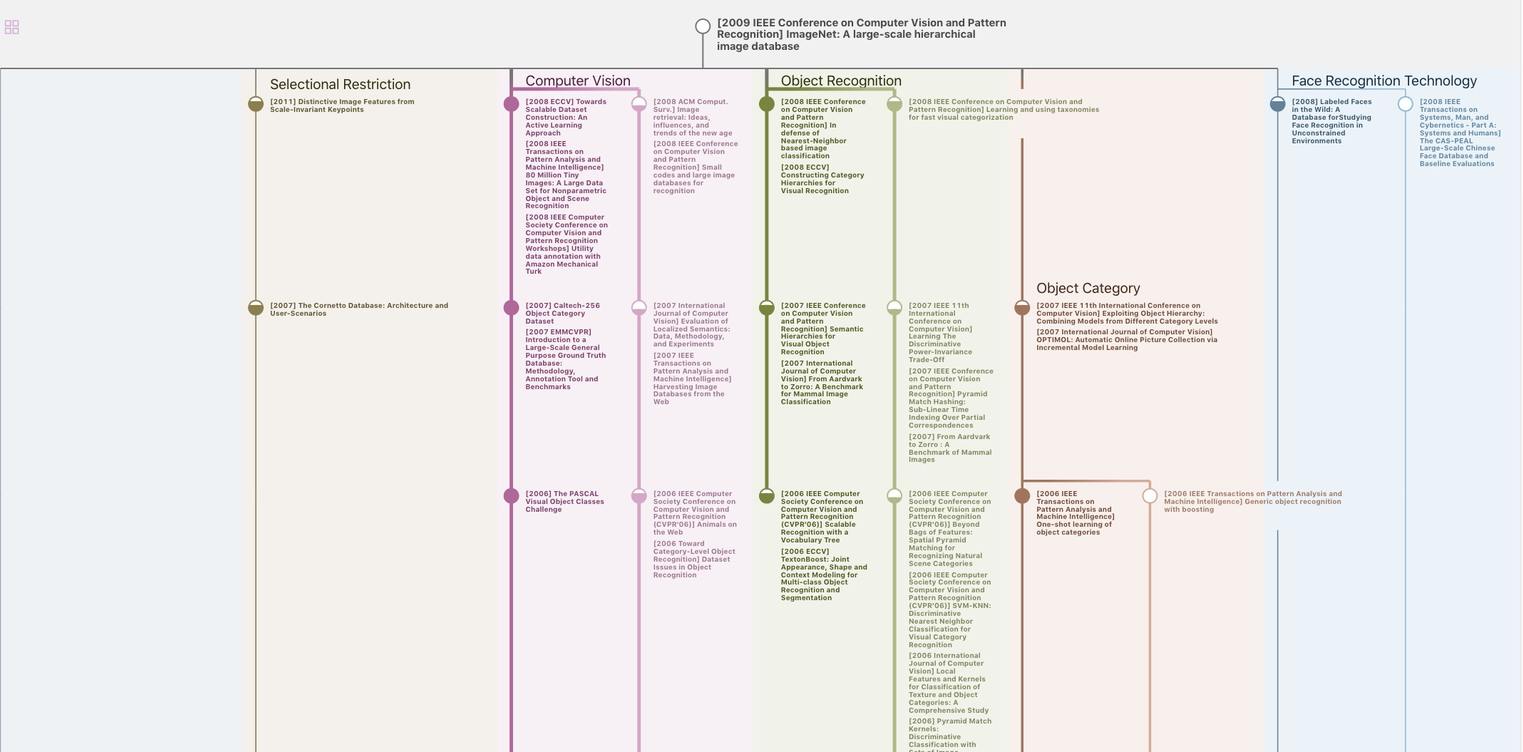
生成溯源树,研究论文发展脉络
Chat Paper
正在生成论文摘要