Visual Explanations of Differentiable Greedy Model Predictions on the Influence Maximization Problem
BIG DATA AND COGNITIVE COMPUTING(2023)
摘要
Social networks have become important objects of study in recent years. Social media marketing has, for example, greatly benefited from the vast literature developed in the past two decades. The study of social networks has taken advantage of recent advances in machine learning to process these immense amounts of data. Automatic emotional labeling of content on social media has, for example, been made possible by the recent progress in natural language processing. In this work, we are interested in the influence maximization problem, which consists of finding the most influential nodes in the social network. The problem is classically carried out using classical performance metrics such as accuracy or recall, which is not the end goal of the influence maximization problem. Our work presents an end-to-end learning model, SGREEDYNN, for the selection of the most influential nodes in a social network, given a history of information diffusion. In addition, this work proposes data visualization techniques to interpret the augmenting performances of our method compared to classical training. The results of this method are confirmed by visualizing the final influence of the selected nodes on network instances with edge bundling techniques. Edge bundling is a visual aggregation technique that makes patterns emerge. It has been shown to be an interesting asset for decision-making. By using edge bundling, we observe that our method chooses more diverse and high-degree nodes compared to the classical training.
更多查看译文
关键词
influence maximization, end-to-end learning, decision-focused learning, graph visualization, edge bundling, differentiable greedy
AI 理解论文
溯源树
样例
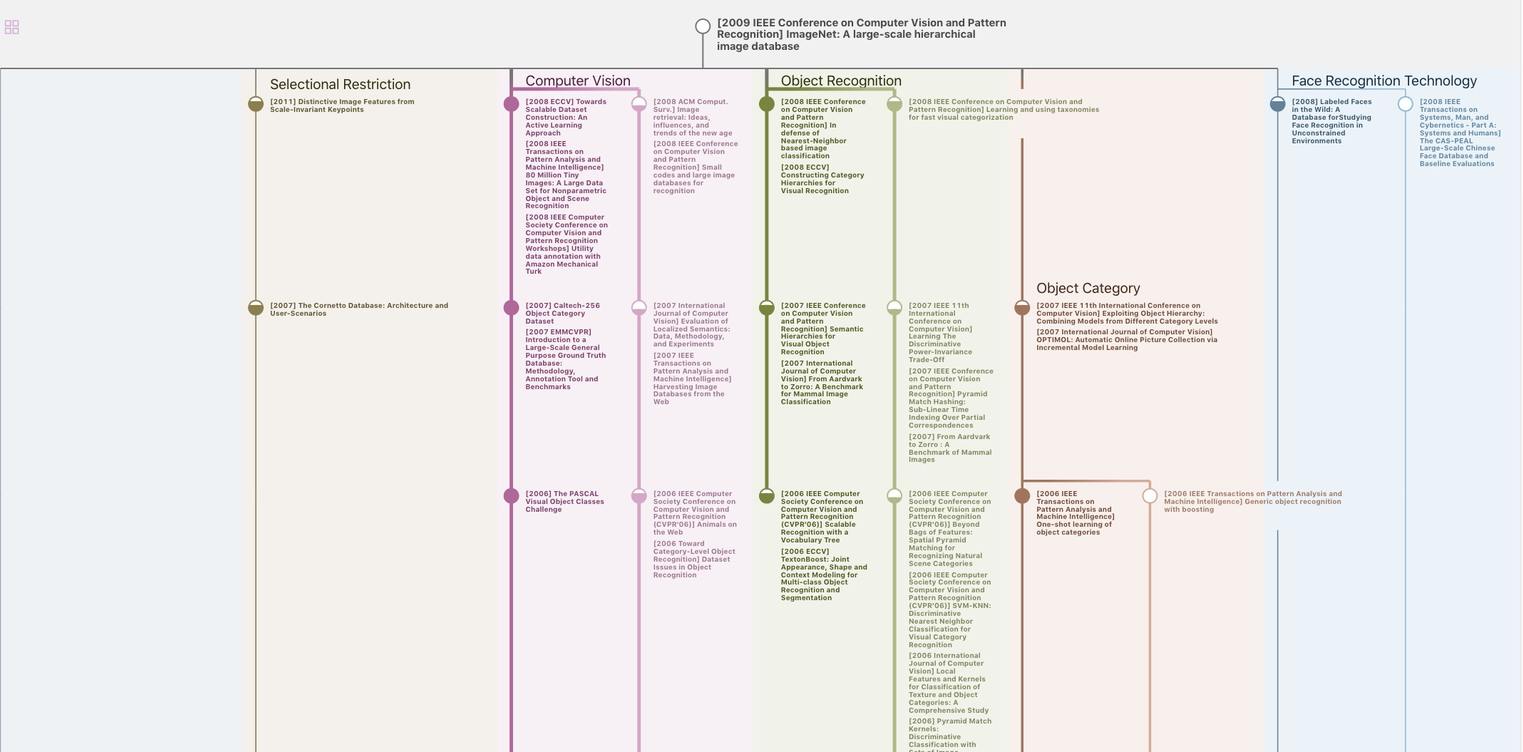
生成溯源树,研究论文发展脉络
Chat Paper
正在生成论文摘要