One and one make eleven: An interpretable neural network for image recognition
KNOWLEDGE-BASED SYSTEMS(2023)
摘要
Although non-interpretable (black-box) deep learning models are well known for their accuracy, interpretable deep learning models should be used for high stake decisions, such as: healthcare. In this paper, we present a novel technique of combining the existing state-of-the-art models, and using them as a base model to build an interpretable deep learning model Comb-ProtoPNet. In contrast to usual technique of combining the logits of two (or more) algorithms to form an ensemble algorithm, we combine the algorithms themselves. Our proposed interpretable model applies a prototype layer on top of the convolutional layers of an ensemble base model. We trained and tested our algorithm over the dataset of chest CT-scan images of COVID-19 patients, pneumonia patients and normal people. The use of a certain combination of blocks from two different state-of-the-art models (statistically) significantly improved the accuracy compared to the individual use of the state-of-the-art models as the base models, and this is where the part of the title “One and one make eleven” comes from.
更多查看译文
关键词
Interpretable,Prototypes,CT-scan,COVID-19,Pneumonia
AI 理解论文
溯源树
样例
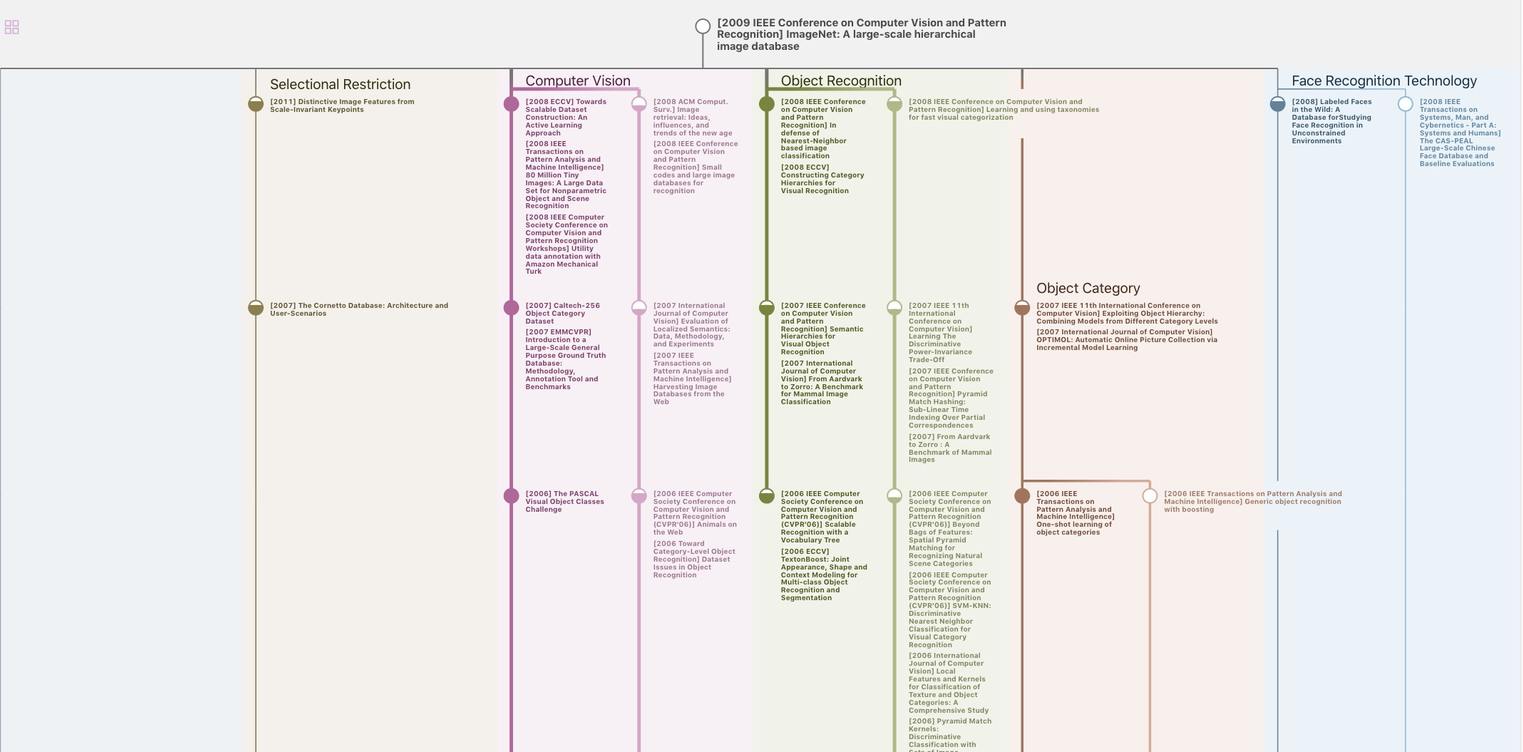
生成溯源树,研究论文发展脉络
Chat Paper
正在生成论文摘要