A systematic survey on recent deep learning-based approaches to multi-object tracking
MULTIMEDIA TOOLS AND APPLICATIONS(2023)
摘要
This survey covers an in-depth review of the state-of-the-art research on Multi-Object Tracking (MOT) from research articles published in 2019 or later in top-tier journals and conferences. We categorize existing MOT research into nine broad categories and discuss the workflow and limitations of each of these categories. Such a classification will enable readers to understand the research trend in different sub-domains of the MOT problem, as well as identify the research gaps. To the best of our knowledge, existing surveys on MOT do not put much emphasis on discussing the tracking step of MOT, which we have addressed here. Additionally, our survey highlights the progress made in MOT by employing recent Deep Learning models such as Transformers, Graph Neural Networks, etc., which also have not been covered in other surveys. It also discusses the challenges faced by the various trackers due to a variety of extrinsic and intrinsic factors. Additionally, we elaborate on the available public datasets, benchmarks, and metrics employed to evaluate the performance of an MOT model and make comparative studies to enlist the important results reported in previous research studies for some popular MOT datasets. This survey will provide the readers with an extensive overview of the state-of-the-art MOT algorithms and their shortcomings, which will help them in designing and developing newer and better MOT algorithms.
更多查看译文
关键词
Deep learning-based MOT,Literature review,MOT datasets,MOT evaluation metrics,MOT comparative study
AI 理解论文
溯源树
样例
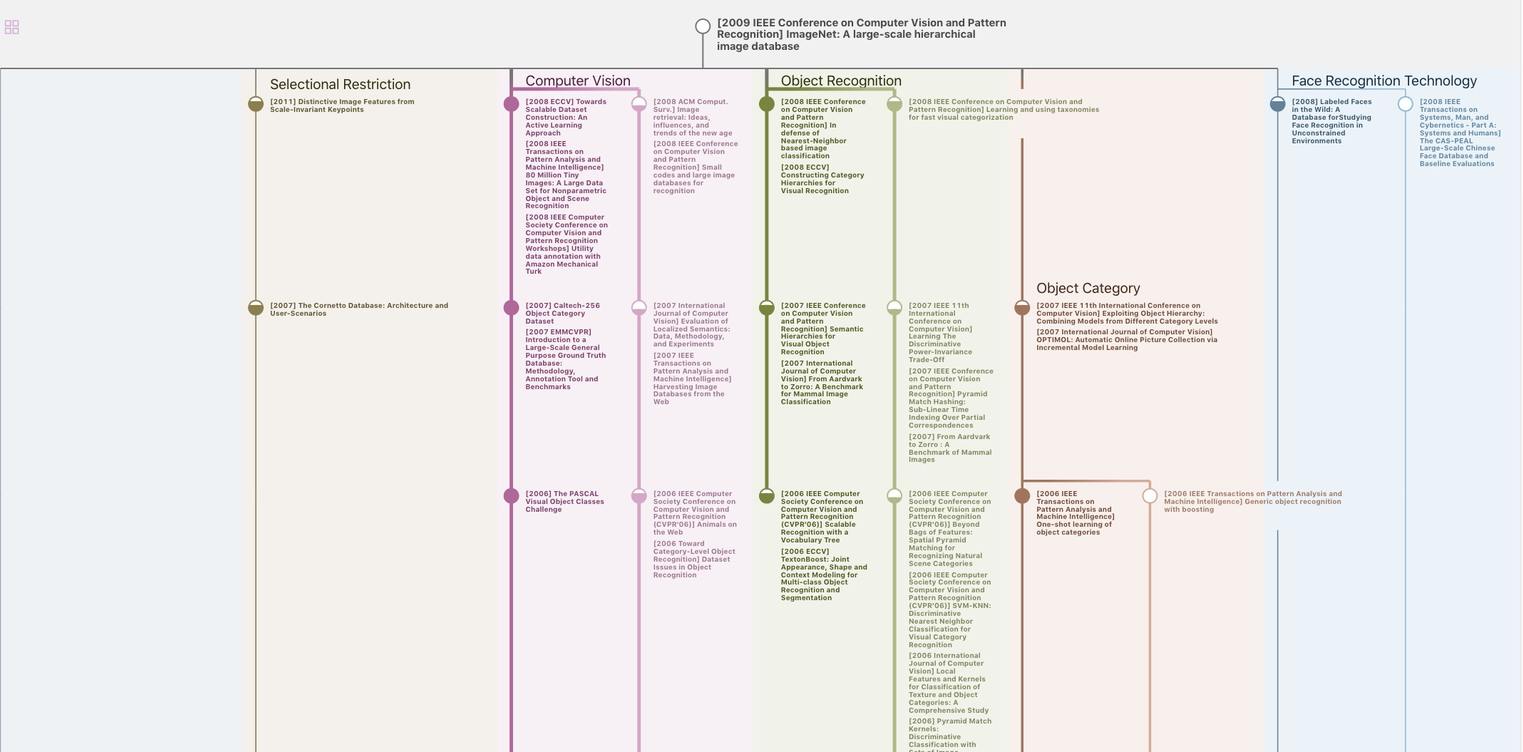
生成溯源树,研究论文发展脉络
Chat Paper
正在生成论文摘要