Performance analysis of neural network-based unified physical layer for indoor hybrid LiFi–WiFi flying networks
NEURAL COMPUTING & APPLICATIONS(2023)
摘要
The recent developments in unmanned aerial vehicles (UAVs) and indoor hybrid LiFi–WiFi networks (HLWNs) present a significant opportunity for creating low-cost, power-efficient, reliable, flexible, and ad-hoc HLWN-enabled indoor flying networks (IFNs). However, to efficiently operate and practically realize indoor HLWN, a unified physical layer (UniPHY) is indispensable for joint communication (control and data transfer) and sensing (e.g., localization). A UniPHY structure reduces costs and increases overall flexibility for HLWN-based IFNs. While conventional block-based wireless transceivers independently designed for LiFi and WiFi offer mediocre performance for a composite UniPHY waveform, a machine learning-based end-to-end learning framework for UniPHY can improve overall error performance and reduce the complexity of UAV transceiver hardware. Therefore, this paper proposes a novel generic end-to-end learning framework for a UniPHY system that can efficiently enable HLWN. The performance of the proposed learning framework based on deep neural networks (DNNs) and convolutional neural networks (CNNs) is investigated. Additionally, we assess the computational complexity of the proposed DNN and CNN learning frameworks. The results demonstrate that the performance of DNNs and CNNs varies depending on the considered channel model. Specifically, the analysis reveals that CNNs outperform traditional DNNs in WiFi (Rayleigh fading-based) channels. In contrast, traditional DNNs perform better than CNNs in LiFi (additive white Gaussian noise (AWGN)-based) channels.
更多查看译文
关键词
indoor hybrid lifi–wifi,unified physical layer,network-based
AI 理解论文
溯源树
样例
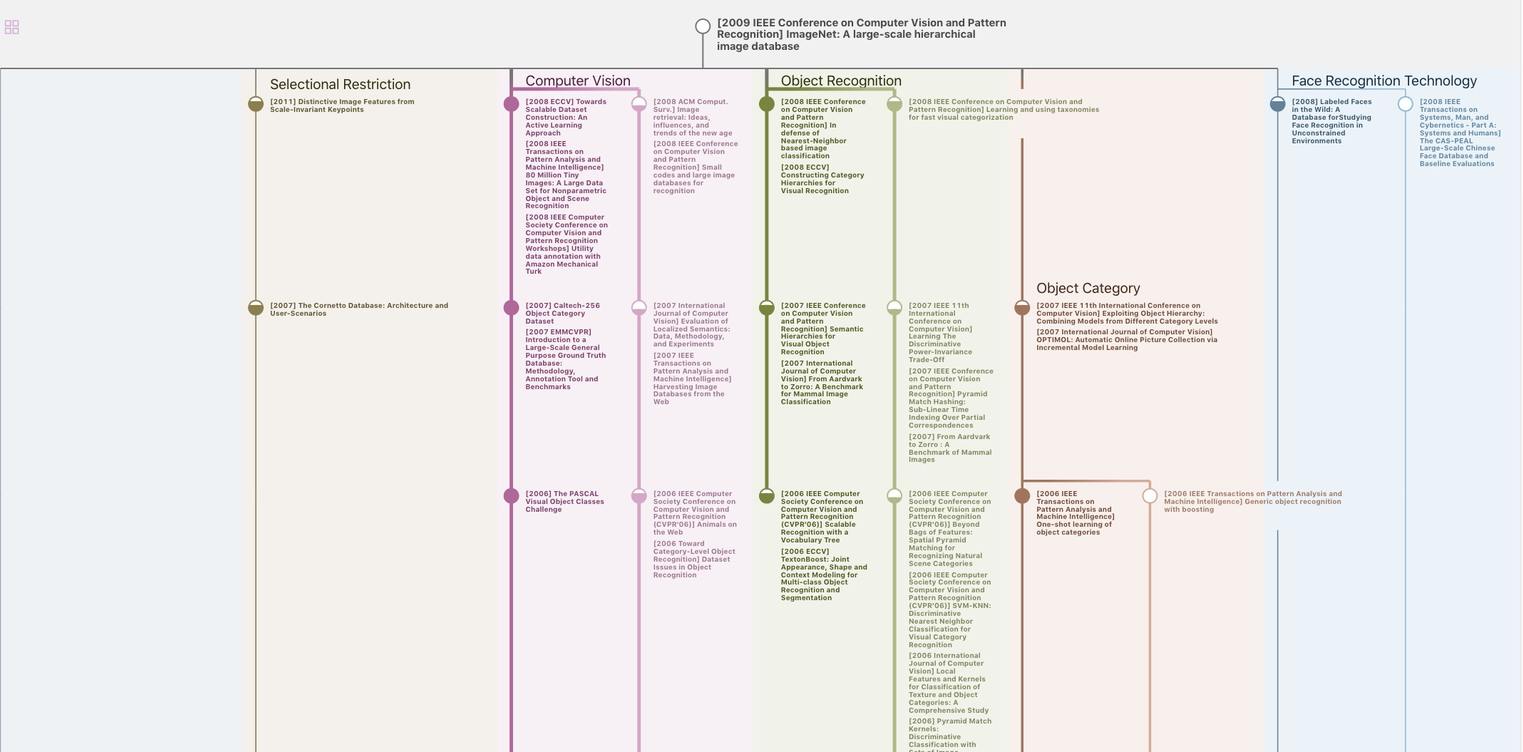
生成溯源树,研究论文发展脉络
Chat Paper
正在生成论文摘要