Knowledge-enhanced multi-task recommendation in hyperbolic space
APPLIED INTELLIGENCE(2023)
摘要
Multi-task learning has recently inspired a series of fruitful research in the field of recommendation due to its ability to handle complex scenarios by associating information between tasks. However, various information suitable for multi-task recommender systems usually produces different degrees of noise. For example, parameter information suitable for one task will affect other tasks, and the real data that is difficult to observe correctly in Euclidean space may be regarded as noise data. To tackle this problem, we propose a novel knowledge-enhanced multi-task recommendation algorithm in hyperbolic space named KMRH. The algorithm employs the alternate training method to alleviate the parameter noise problem in complex recommendation scenarios. Specifically, we design a novel knowledge enhancement strategy in the Poincare sphere, which exploits hyperbolic embeddings to capture knowledge graphs of complex structured data. Finally, we adopt spatial distance as a metric to distinguish positive and negative samples at different locations, thereby limiting the detrimental impact of noise components on the recommendation model. Extensive experiments on three benchmark datasets demonstrate that our proposed algorithm achieves significant improvements over other state-of-the-art algorithms.
更多查看译文
关键词
hyperbolic space,knowledge-enhanced,multi-task
AI 理解论文
溯源树
样例
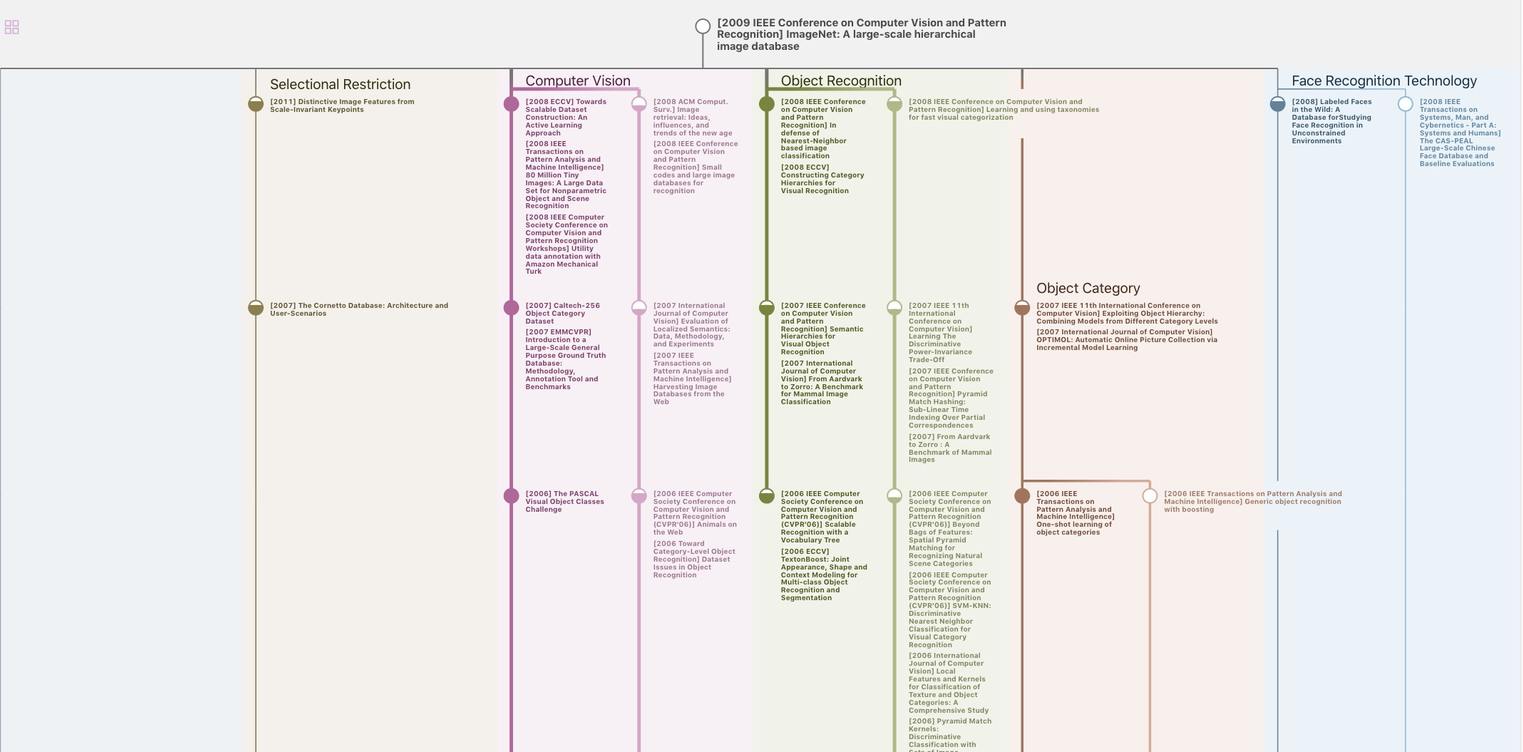
生成溯源树,研究论文发展脉络
Chat Paper
正在生成论文摘要