An evaluation strategy to select and discard sampling preprocessing methods for imbalanced datasets: A focus on classification models
CHEMOMETRICS AND INTELLIGENT LABORATORY SYSTEMS(2023)
摘要
This work proposes a strategy to help in selecting a synthetic sampling algorithm that enhances the figures of merit of imbalanced pattern recognition models. Three imbalance quantitative structure-activity relationship (QSAR) datasets were used to highlight the efficiency of the present approach. This approach evaluates the pattern recognition algorithms' figures of merit using an experimental design organization. The factor analysis was performed using each figure of merits individually and simultaneously with the Derringer and Suich desirability function. Here, the aim is to better understand the influence of synthetic sampling on pattern recognition models' figure of merits to develop a strategy to choose the sample that may lead to predictions with enhanced overall figures of metric and discard the sample that prejudices the models. Three undersampling (regular downsampling, undersampling based on clustering and Tomek links), three oversampling (regular upsampling, SMOTE, and ADASYN), and two hybrid sampling (SMOTE-TL and SPIDER) methods were used to balance the datasets to build the models. Due to these datasets’ non-Gaussian characteristics proven by the multivariate Shapiro‒Wilk test, the classification models were based on support vector machine with radial base function, C5.0, artificial neural networks, extreme gradient boosting, and random forest algorithms. For these datasets, it was observed that oversampling methods tend to increase the sensitivity and accuracy while undersampling increases the accuracy and specificity. Hybrid methods tend to improve all the figures of merit. However, it is harder to correctly balance the samples between the classes, especially when few variables for a sample are available. Comparison with the original manuscript data results showed that proper sampling preprocessing can enhance the figure of merits of imbalanced datasets.
更多查看译文
关键词
Chemometrics,QSAR,Sampling methods,Imbalanced,Machine learning
AI 理解论文
溯源树
样例
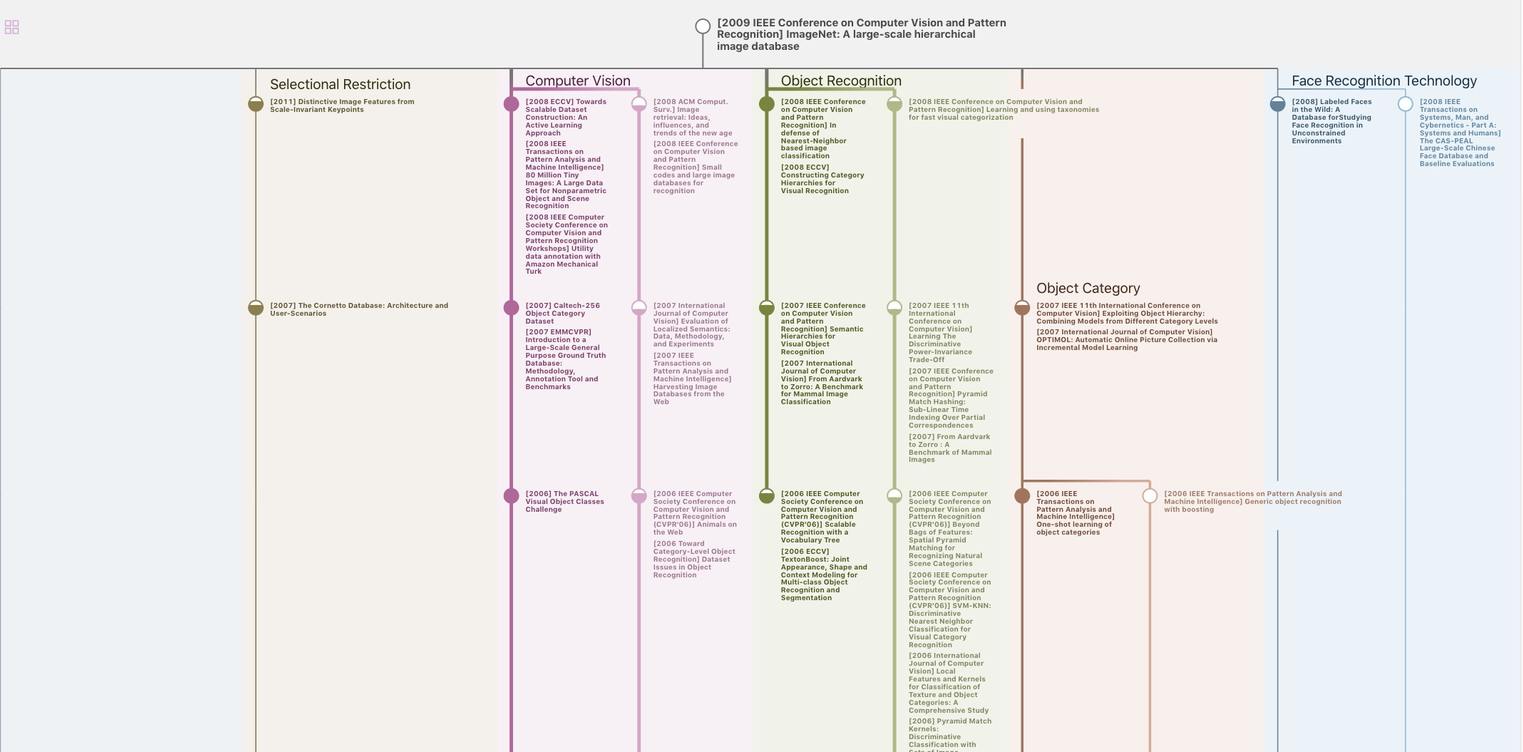
生成溯源树,研究论文发展脉络
Chat Paper
正在生成论文摘要