Dynamic visual-guided selection for zero-shot learning
JOURNAL OF SUPERCOMPUTING(2024)
摘要
Zero-shot learning (ZSL) methods currently employed to identify seen or unseen classes rely on semantic attribute prototypes or class information. However, hand-annotated attributes are only for the category rather than for each image belonging to that category. Furthermore, attribute information is inconsistent across different images of the same category due to variant views. Therefore, we propose a dynamic visual-guided selection (DVGS) which helps dynamically focus on different regions and refines class prototype on each image. Instead of directly aligning an image's global feature with its semantic class vector or its local features with all attribute vectors, the proposed method learns a vision-guided soft mask to refine the class prototype for each image. Additionally, it discovers the most task-relevant regions for fine-grained recognition with the refined class prototype. Extensive experiments on three benchmarks verify the effectiveness of our DVGS and achieve the new state-of-the-art. Our DVGS achieved the best results on fine-grained datasets within both the conventional zero-shot learning (CZSL) and generalized zero-shot learning (GZSL) settings. In particular, on the SUN dataset, our DVGS demonstrates a significant superiority of 10.2% in the CZSL setting compared with the second-best approach. Similarly, our method outperforms the second-best method by an average of 4% on CUB in both the CZSL and GZSL settings. Despite securing the second-best result on the AWA2 dataset, DVGS remains closely competitive, trailing the best performance by a mere 3.4% in CZSL and 1.2% in GZSL.
更多查看译文
关键词
Visual-guided selection,Class prototype refinement,Task-relevant regions,Zero-shot learning
AI 理解论文
溯源树
样例
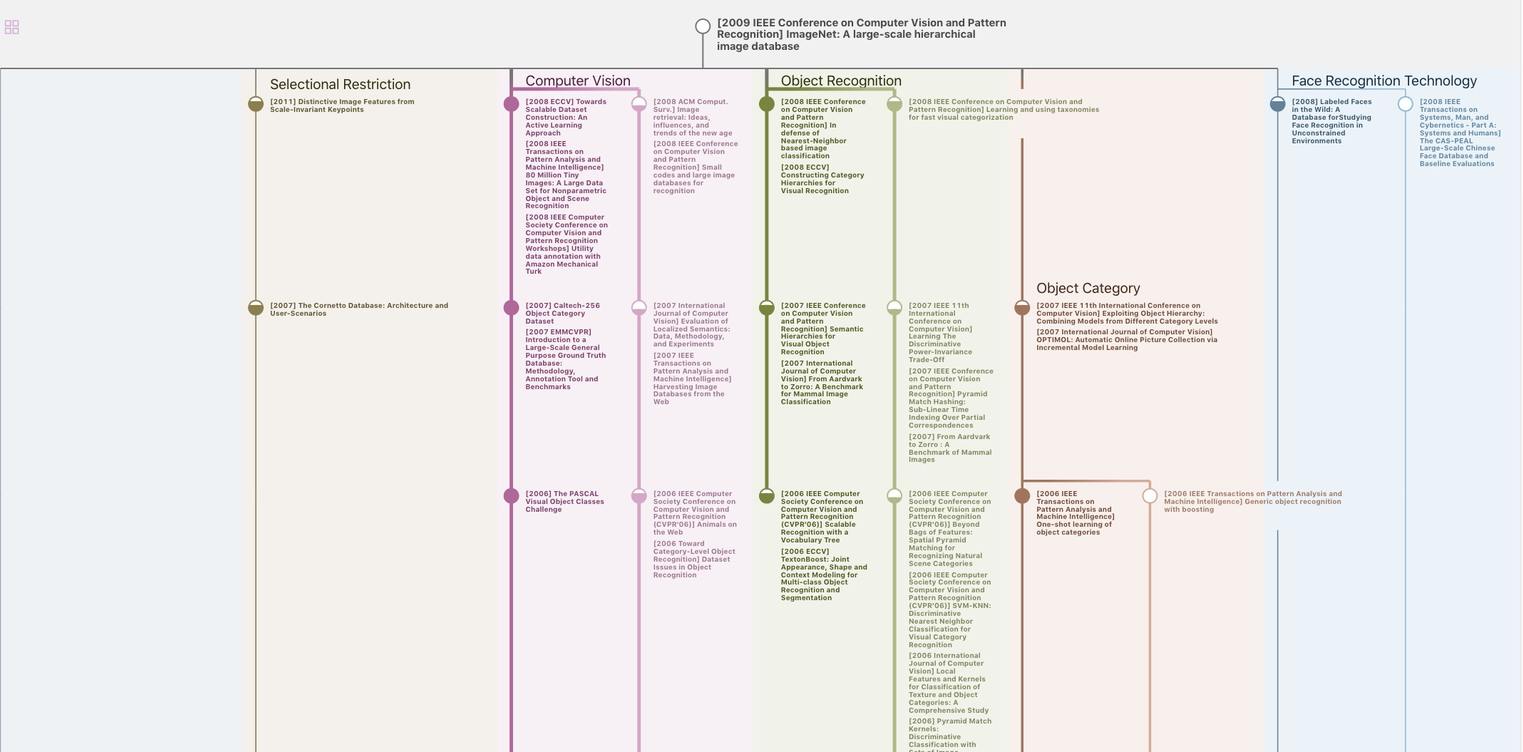
生成溯源树,研究论文发展脉络
Chat Paper
正在生成论文摘要