Universal and interpretable classification of atomistic structural transitions via unsupervised graph learning
APPLIED PHYSICS LETTERS(2023)
摘要
Materials processing often occurs under extreme dynamic conditions leading to a multitude of unique structural environments. These structural environments generally occur at high temperatures and/or high pressures, often under non-equilibrium conditions, which results in drastic changes in the material's structure over time. Computational techniques, such as molecular dynamics simulations, can probe the atomic regime under these extreme conditions. However, characterizing the resulting diverse atomistic structures as a material undergoes extreme changes in its structure has proved challenging due to the inherently non-linear relationship between structures as large-scale changes occur. Here, we introduce SODAS++, a universal graph neural network framework, that can accurately and intuitively quantify the atomistic structural evolution corresponding to the transition between any two arbitrary phases. We showcase SODAS++ for both solid-solid and solid-liquid transitions for systems of increasing geometric and chemical complexity, such as colloidal systems, elemental Al, rutile and amorphous TiO2, and the non-stoichiometric ternary alloy Ag26Au5Cu19. We show that SODAS++ can accurately quantify all transitions in a physically interpretable manner, showcasing the power of unsupervised graph neural network encodings for capturing the complex and non-linear pathway, a material's structure takes as it evolves.
更多查看译文
关键词
atomistic structural transitions,interpretable classification,graph
AI 理解论文
溯源树
样例
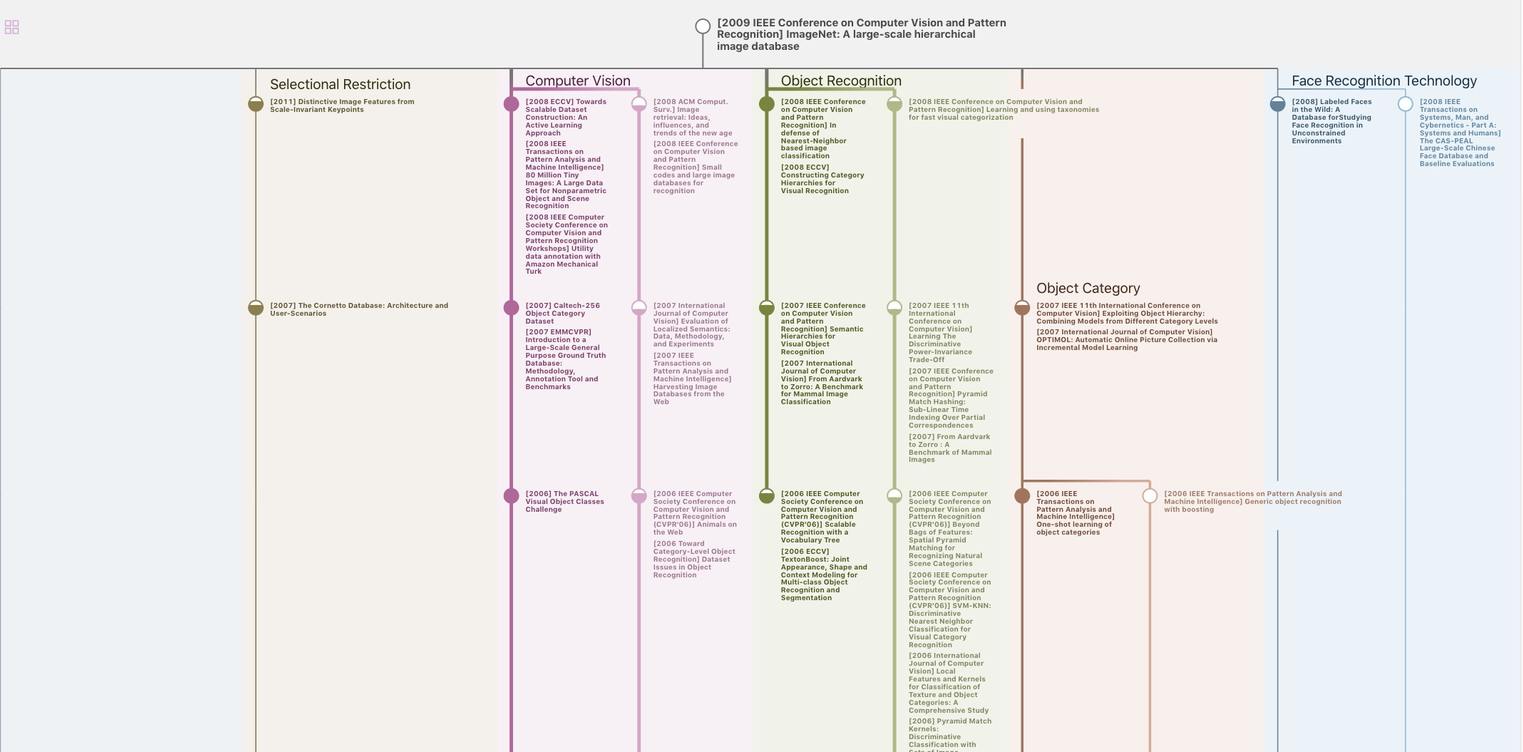
生成溯源树,研究论文发展脉络
Chat Paper
正在生成论文摘要